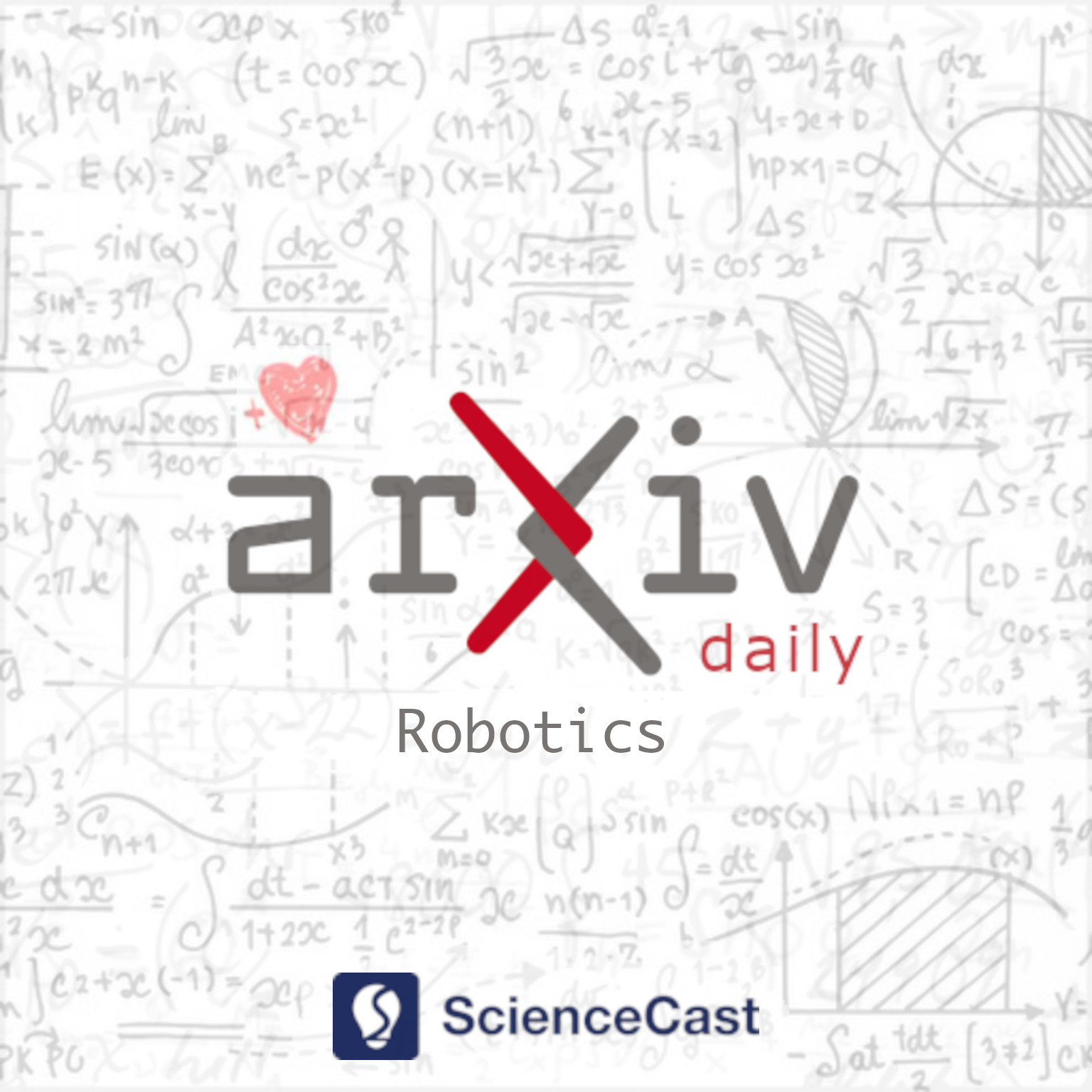
Robotics (cs.RO)
Fri, 18 Aug 2023
1.Distributed Robust Learning-Based Backstepping Control Aided with Neurodynamics for Consensus Formation Tracking of Underwater Vessels
Authors:Tao Yan, Zhe Xu, Simon X. Yang
Abstract: This paper addresses distributed robust learning-based control for consensus formation tracking of multiple underwater vessels, in which the system parameters of the marine vessels are assumed to be entirely unknown and subject to the modeling mismatch, oceanic disturbances, and noises. Towards this end, graph theory is used to allow us to synthesize the distributed controller with a stability guarantee. Due to the fact that the parameter uncertainties only arise in the vessels' dynamic model, the backstepping control technique is then employed. Subsequently, to overcome the difficulties in handling time-varying and unknown systems, an online learning procedure is developed in the proposed distributed formation control protocol. Moreover, modeling errors, environmental disturbances, and measurement noises are considered and tackled by introducing a neurodynamics model in the controller design to obtain a robust solution. Then, the stability analysis of the overall closed-loop system under the proposed scheme is provided to ensure the robust adaptive performance at the theoretical level. Finally, extensive simulation experiments are conducted to further verify the efficacy of the presented distributed control protocol.
2.Multi-Level Compositional Reasoning for Interactive Instruction Following
Authors:Suvaansh Bhambri, Byeonghwi Kim, Jonghyun Choi
Abstract: Robotic agents performing domestic chores by natural language directives are required to master the complex job of navigating environment and interacting with objects in the environments. The tasks given to the agents are often composite thus are challenging as completing them require to reason about multiple subtasks, e.g., bring a cup of coffee. To address the challenge, we propose to divide and conquer it by breaking the task into multiple subgoals and attend to them individually for better navigation and interaction. We call it Multi-level Compositional Reasoning Agent (MCR-Agent). Specifically, we learn a three-level action policy. At the highest level, we infer a sequence of human-interpretable subgoals to be executed based on language instructions by a high-level policy composition controller. At the middle level, we discriminatively control the agent's navigation by a master policy by alternating between a navigation policy and various independent interaction policies. Finally, at the lowest level, we infer manipulation actions with the corresponding object masks using the appropriate interaction policy. Our approach not only generates human interpretable subgoals but also achieves 2.03% absolute gain to comparable state of the arts in the efficiency metric (PLWSR in unseen set) without using rule-based planning or a semantic spatial memory.
3.Robust Quadrupedal Locomotion via Risk-Averse Policy Learning
Authors:Jiyuan Shi, Chenjia Bai, Haoran He, Lei Han, Dong Wang, Bin Zhao, Xiu Li, Xuelong Li
Abstract: The robustness of legged locomotion is crucial for quadrupedal robots in challenging terrains. Recently, Reinforcement Learning (RL) has shown promising results in legged locomotion and various methods try to integrate privileged distillation, scene modeling, and external sensors to improve the generalization and robustness of locomotion policies. However, these methods are hard to handle uncertain scenarios such as abrupt terrain changes or unexpected external forces. In this paper, we consider a novel risk-sensitive perspective to enhance the robustness of legged locomotion. Specifically, we employ a distributional value function learned by quantile regression to model the aleatoric uncertainty of environments, and perform risk-averse policy learning by optimizing the worst-case scenarios via a risk distortion measure. Extensive experiments in both simulation environments and a real Aliengo robot demonstrate that our method is efficient in handling various external disturbances, and the resulting policy exhibits improved robustness in harsh and uncertain situations in legged locomotion. Videos are available at https://risk-averse-locomotion.github.io/.
4.Integrating Expert Guidance for Efficient Learning of Safe Overtaking in Autonomous Driving Using Deep Reinforcement Learning
Authors:Jinxiong Lu, Gokhan Alcan, Ville Kyrki
Abstract: Overtaking on two-lane roads is a great challenge for autonomous vehicles, as oncoming traffic appearing on the opposite lane may require the vehicle to change its decision and abort the overtaking. Deep reinforcement learning (DRL) has shown promise for difficult decision problems such as this, but it requires massive number of data, especially if the action space is continuous. This paper proposes to incorporate guidance from an expert system into DRL to increase its sample efficiency in the autonomous overtaking setting. The guidance system developed in this study is composed of constrained iterative LQR and PID controllers. The novelty lies in the incorporation of a fading guidance function, which gradually decreases the effect of the expert system, allowing the agent to initially learn an appropriate action swiftly and then improve beyond the performance of the expert system. This approach thus combines the strengths of traditional control engineering with the flexibility of learning systems, expanding the capabilities of the autonomous system. The proposed methodology for autonomous vehicle overtaking does not depend on a particular DRL algorithm and three state-of-the-art algorithms are used as baselines for evaluation. Simulation results show that incorporating expert system guidance improves state-of-the-art DRL algorithms greatly in both sample efficiency and driving safety.
5.Pose-Following with Dual Quaternions
Authors:Jon Arrizabalaga, Markus Ryll
Abstract: This work focuses on pose-following, a variant of path-following in which the goal is to steer the system's position and attitude along a path with a moving frame attached to it. Full body motion control, while accounting for the additional freedom to self-regulate the progress along the path, is an appealing trade-off. Towards this end, we extend the well-established dual quaternion-based pose-tracking method into a pose-following control law. Specifically, we derive the equations of motion for the full pose error between the geometric reference and the rigid body in the form of a dual quaternion and dual twist. Subsequently, we formulate an almost globally asymptotically stable control law. The global attractivity of the presented approach is validated in a spatial example, while its benefits over pose-tracking are showcased through a planar case-study.
6.3D Model-free Visual localization System from Essential Matrix under Local Planar Motion
Authors:Yanmei Jiao, Binxin Zhang, Peng Jiang, Rong Xiong, Yue Wang
Abstract: Visual localization plays a critical role in the functionality of low-cost autonomous mobile robots. Current state-of-the-art approaches to accurate visual localization are 3D scene-specific, requiring additional computational and storage resources to construct a 3D scene model when facing a new environment. An alternative approach of directly using a database of 2D images for visual localization offers more flexibility. However, such methods currently suffer from limited localization accuracy. In this paper, we propose a robust and accurate multiple checking-based 3D model-free visual localization system that addresses the aforementioned issues. The core idea is to model the local planar motion characteristic of general ground-moving robots into both essential matrix estimation and triangulation stages to obtain two minimal solutions. By embedding the proposed minimal solutions into the multiple checking scheme, the proposed 3D model-free visual localization framework demonstrates high accuracy and robustness in both simulation and real-world experiments.
7.Towards Human-Robot Collaboration with Parallel Robots by Kinetostatic Analysis, Impedance Control and Contact Detection
Authors:Aran Mohammad, Moritz Schappler, Tobias Ortmaier
Abstract: Parallel robots provide the potential to be leveraged for human-robot collaboration (HRC) due to low collision energies even at high speeds resulting from their reduced moving masses. However, the risk of unintended contact with the leg chains increases compared to the structure of serial robots. As a first step towards HRC, contact cases on the whole parallel robot structure are investigated and a disturbance observer based on generalized momenta and measurements of motor current is applied. In addition, a Kalman filter and a second-order sliding-mode observer based on generalized momenta are compared in terms of error and detection time. Gearless direct drives with low friction improve external force estimation and enable low impedance. The experimental validation is performed with two force-torque sensors and a kinetostatic model. This allows a new identification method of the motor torque constant of an assembled parallel robot to estimate external forces from the motor current and via a dynamics model. A Cartesian impedance control scheme for compliant robot-environmental dynamics with stiffness from 0.1-2N/mm and the force observation for low forces over the entire structure are validated. The observers are used for collisions and clamping at velocities of 0.4-0.9m/s for detection within 9-58ms and a reaction in the form of a zero-g mode.
8.Collision Isolation and Identification Using Proprioceptive Sensing for Parallel Robots to Enable Human-Robot Collaboration
Authors:Aran Mohammad, Moritz Schappler, Tobias Ortmaier
Abstract: Parallel robots (PRs) allow for higher speeds in human-robot collaboration due to their lower moving masses but are more prone to unintended contact. For a safe reaction, knowledge of the location and force of a collision is useful. A novel algorithm for collision isolation and identification with proprioceptive information for a real PR is the scope of this work. To classify the collided body, the effects of contact forces at the links and platform of the PR are analyzed using a kinetostatic projection. This insight enables the derivation of features from the line of action of the estimated external force. The significance of these features is confirmed in experiments for various load cases. A feedforward neural network (FNN) classifies the collided body based on these physically modeled features. Generalization with the FNN to 300k load cases on the whole robot structure in other joint angle configurations is successfully performed with a collision-body classification accuracy of 84% in the experiments. Platform collisions are isolated and identified with an explicit solution, while a particle filter estimates the location and force of a contact on a kinematic chain. Updating the particle filter with estimated external joint torques leads to an isolation error of less than 3cm and an identification error of 4N in a real-world experiment.
9.Safe Collision and Clamping Reaction for Parallel Robots During Human-Robot Collaboration
Authors:Aran Mohammad, Moritz Schappler, Tim-Lukas Habich, Tobias Ortmaier
Abstract: Parallel robots (PRs) offer the potential for safe human-robot collaboration because of their low moving masses. Due to the in-parallel kinematic chains, the risk of contact in the form of collisions and clamping at a chain increases. Ensuring safety is investigated in this work through various contact reactions on a real planar PR. External forces are estimated based on proprioceptive information and a dynamics model, which allows contact detection. Retraction along the direction of the estimated line of action provides an instantaneous response to limit the occurring contact forces within the experiment to 70N at a maximum velocity 0.4m/s. A reduction in the stiffness of a Cartesian impedance control is investigated as a further strategy. For clamping, a feedforward neural network (FNN) is trained and tested in different joint angle configurations to classify whether a collision or clamping occurs with an accuracy of 80%. A second FNN classifies the clamping kinematic chain to enable a subsequent kinematic projection of the clamping joint angle onto the rotational platform coordinates. In this way, a structure opening is performed in addition to the softer retraction movement. The reaction strategies are compared in real-world experiments at different velocities and controller stiffnesses to demonstrate their effectiveness. The results show that in all collision and clamping experiments the PR terminates the contact in less than 130ms.
10.Quantifying Uncertainties of Contact Classifications in a Human-Robot Collaboration with Parallel Robots
Authors:Aran Mohammad, Hendrik Muscheid, Moritz Schappler, Thomas Seel
Abstract: In human-robot collaboration, unintentional physical contacts occur in the form of collisions and clamping, which must be detected and classified separately for a reaction. If certain collision or clamping situations are misclassified, reactions might occur that make the true contact case more dangerous. This work analyzes data-driven modeling based on physically modeled features like estimated external forces for clamping and collision classification with a real parallel robot. The prediction reliability of a feedforward neural network is investigated. Quantification of the classification uncertainty enables the distinction between safe versus unreliable classifications and optimal reactions like a retraction movement for collisions, structure opening for the clamping joint, and a fallback reaction in the form of a zero-g mode. This hypothesis is tested with experimental data of clamping and collision cases by analyzing dangerous misclassifications and then reducing them by the proposed uncertainty quantification. Finally, it is investigated how the approach of this work influences correctly classified clamping and collision scenarios.
11.Towards a Modular Architecture for Science Factories
Authors:Rafael Vescovi, Tobias Ginsburg, Kyle Hippe, Doga Ozgulbas, Casey Stone, Abraham Stroka, Rory Butler, Ben Blaiszik, Tom Brettin, Kyle Chard, Mark Hereld, Arvind Ramanathan, Rick Stevens, Aikaterini Vriza, Jie Xu, Qingteng Zhang, Ian Foster
Abstract: Advances in robotic automation, high-performance computing (HPC), and artificial intelligence (AI) encourage us to conceive of science factories: large, general-purpose computation- and AI-enabled self-driving laboratories (SDLs) with the generality and scale needed both to tackle large discovery problems and to support thousands of scientists. Science factories require modular hardware and software that can be replicated for scale and (re)configured to support many applications. To this end, we propose a prototype modular science factory architecture in which reconfigurable modules encapsulating scientific instruments are linked with manipulators to form workcells, that can themselves be combined to form larger assemblages, and linked with distributed computing for simulation, AI model training and inference, and related tasks. Workflows that perform sets of actions on modules can be specified, and various applications, comprising workflows plus associated computational and data manipulation steps, can be run concurrently. We report on our experiences prototyping this architecture and applying it in experiments involving 15 different robotic apparatus, five applications (one in education, two in biology, two in materials), and a variety of workflows, across four laboratories. We describe the reuse of modules, workcells, and workflows in different applications, the migration of applications between workcells, and the use of digital twins, and suggest directions for future work aimed at yet more generality and scalability. Code and data are available at https://ad-sdl.github.io/wei2023 and in the Supplementary Information
12.DoCRL: Double Critic Deep Reinforcement Learning for Mapless Navigation of a Hybrid Aerial Underwater Vehicle with Medium Transition
Authors:Ricardo B. Grando, Junior C. de Jesus, Victor A. Kich, Alisson H. Kolling, Rodrigo S. Guerra, Paulo L. J. Drews-Jr
Abstract: Deep Reinforcement Learning (Deep-RL) techniques for motion control have been continuously used to deal with decision-making problems for a wide variety of robots. Previous works showed that Deep-RL can be applied to perform mapless navigation, including the medium transition of Hybrid Unmanned Aerial Underwater Vehicles (HUAUVs). These are robots that can operate in both air and water media, with future potential for rescue tasks in robotics. This paper presents new approaches based on the state-of-the-art Double Critic Actor-Critic algorithms to address the navigation and medium transition problems for a HUAUV. We show that double-critic Deep-RL with Recurrent Neural Networks using range data and relative localization solely improves the navigation performance of HUAUVs. Our DoCRL approaches achieved better navigation and transitioning capability, outperforming previous approaches.
13.High Aspect Ratio Multi-stage Ducted Electroaerodynamic Thrusters for Micro Air Vehicle Propulsion
Authors:C. Luke Nelson, Daniel S. Drew
Abstract: Electroaerodynamic propulsion, where force is produced through collisions between electrostatically accelerated ions and neutral air molecules, is an attractive alternative to propeller- and flapping wing-based methods for micro air vehicle (MAV) flight due to its silent and solid-state nature. One major barrier to adoption is its limited thrust efficiency at useful disk loading levels. Ducted actuators comprising multiple serially-integrated acceleration stages are a potential solution, allowing individual stages to operate at higher efficiency while maintaining a useful total thrust, and potentially improving efficiency through various aerodynamic and fluid dynamic mechanisms. In this work, we investigate the effects of duct and emitter electrode geometries on actuator performance, then show how a combination of increasing cross-sectional aspect ratio and serial integration of multiple stages can be used to produce overall thrust densities comparable to commercial propulsors. An optimized five-stage device attains a thrust density of about 18 N/m$^2$ at a thrust efficiency of about 2 mN/W, among the highest values ever measured at this scale. We further show how this type of thruster can be integrated under the wings of a MAV-scale fixed wing platform, pointing towards future use as a distributed propulsion system.