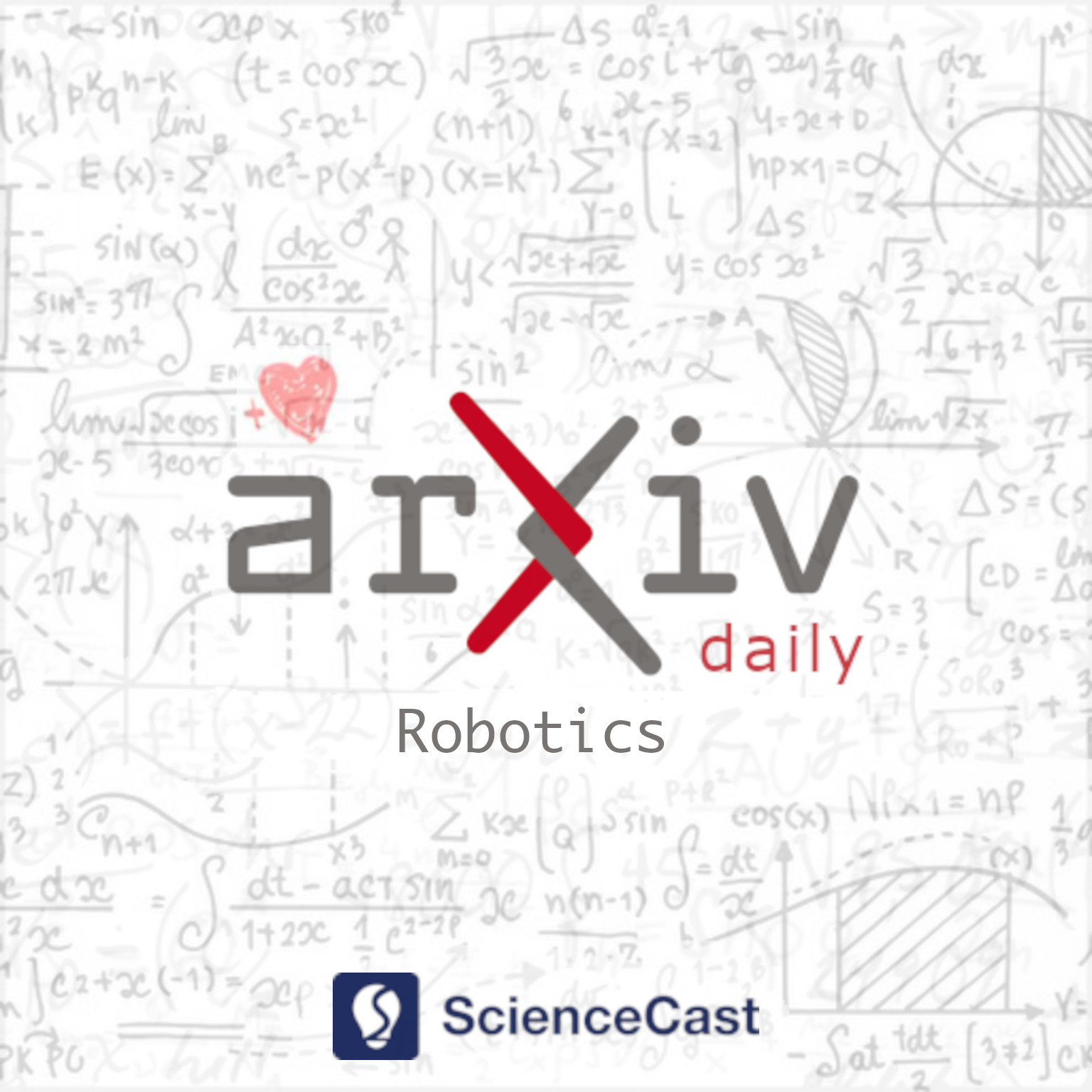
Robotics (cs.RO)
Wed, 23 Aug 2023
1.Value of Assistance for Mobile Agents
Authors:Adi Amuzig, David Dovrat, Sarah Keren
Abstract: Mobile robotic agents often suffer from localization uncertainty which grows with time and with the agents' movement. This can hinder their ability to accomplish their task. In some settings, it may be possible to perform assistive actions that reduce uncertainty about a robot's location. For example, in a collaborative multi-robot system, a wheeled robot can request assistance from a drone that can fly to its estimated location and reveal its exact location on the map or accompany it to its intended location. Since assistance may be costly and limited, and may be requested by different members of a team, there is a need for principled ways to support the decision of which assistance to provide to an agent and when, as well as to decide which agent to help within a team. For this purpose, we propose Value of Assistance (VOA) to represent the expected cost reduction that assistance will yield at a given point of execution. We offer ways to compute VOA based on estimations of the robot's future uncertainty, modeled as a Gaussian process. We specify conditions under which our VOA measures are valid and empirically demonstrate the ability of our measures to predict the agent's average cost reduction when receiving assistance in both simulated and real-world robotic settings.
2.Multi-Modal Multi-Task (3MT) Road Segmentation
Authors:Erkan Milli, Özgür Erkent, Asım Egemen Yılmaz
Abstract: Multi-modal systems have the capacity of producing more reliable results than systems with a single modality in road detection due to perceiving different aspects of the scene. We focus on using raw sensor inputs instead of, as it is typically done in many SOTA works, leveraging architectures that require high pre-processing costs such as surface normals or dense depth predictions. By using raw sensor inputs, we aim to utilize a low-cost model thatminimizes both the pre-processing andmodel computation costs. This study presents a cost-effective and highly accurate solution for road segmentation by integrating data from multiple sensorswithin a multi-task learning architecture.Afusion architecture is proposed in which RGB and LiDAR depth images constitute the inputs of the network. Another contribution of this study is to use IMU/GNSS (inertial measurement unit/global navigation satellite system) inertial navigation system whose data is collected synchronously and calibrated with a LiDAR-camera to compute aggregated dense LiDAR depth images. It has been demonstrated by experiments on the KITTI dataset that the proposed method offers fast and high-performance solutions. We have also shown the performance of our method on Cityscapes where raw LiDAR data is not available. The segmentation results obtained for both full and half resolution images are competitive with existing methods. Therefore, we conclude that our method is not dependent only on raw LiDAR data; rather, it can be used with different sensor modalities. The inference times obtained in all experiments are very promising for real-time experiments.
3.MARC: Multipolicy and Risk-aware Contingency Planning for Autonomous Driving
Authors:Tong Li, Lu Zhang, Sikang Liu, Shaojie Shen
Abstract: Generating safe and non-conservative behaviors in dense, dynamic environments remains challenging for automated vehicles due to the stochastic nature of traffic participants' behaviors and their implicit interaction with the ego vehicle. This paper presents a novel planning framework, Multipolicy And Risk-aware Contingency planning (MARC), that systematically addresses these challenges by enhancing the multipolicy-based pipelines from both behavior and motion planning aspects. Specifically, MARC realizes a critical scenario set that reflects multiple possible futures conditioned on each semantic-level ego policy. Then, the generated policy-conditioned scenarios are further formulated into a tree-structured representation with a dynamic branchpoint based on the scene-level divergence. Moreover, to generate diverse driving maneuvers, we introduce risk-aware contingency planning, a bi-level optimization algorithm that simultaneously considers multiple future scenarios and user-defined risk tolerance levels. Owing to the more unified combination of behavior and motion planning layers, our framework achieves efficient decision-making and human-like driving maneuvers. Comprehensive experimental results demonstrate superior performance to other strong baselines in various environments.
4.Identifying Reaction-Aware Driving Styles of Stochastic Model Predictive Controlled Vehicles by Inverse Reinforcement Learning
Authors:Ni Dang, Tao Shi, Zengjie Zhang, Wanxin Jin, Marion Leibold, Martin Buss
Abstract: The driving style of an Autonomous Vehicle (AV) refers to how it behaves and interacts with other AVs. In a multi-vehicle autonomous driving system, an AV capable of identifying the driving styles of its nearby AVs can reliably evaluate the risk of collisions and make more reasonable driving decisions. However, there has not been a consistent definition of driving styles for an AV in the literature, although it is considered that the driving style is encoded in the AV's trajectories and can be identified using Maximum Entropy Inverse Reinforcement Learning (ME-IRL) methods as a cost function. Nevertheless, an important indicator of the driving style, i.e., how an AV reacts to its nearby AVs, is not fully incorporated in the feature design of previous ME-IRL methods. In this paper, we describe the driving style as a cost function of a series of weighted features. We design additional novel features to capture the AV's reaction-aware characteristics. Then, we identify the driving styles from the demonstration trajectories generated by the Stochastic Model Predictive Control (SMPC) using a modified ME-IRL method with our newly proposed features. The proposed method is validated using MATLAB simulation and an off-the-shelf experiment.
5.Path-Constrained State Estimation for Rail Vehicles
Authors:Cornelius von Einem, Andrei Cramariuc, Roland Siegwart, Cesar Cadena, Florian Tschopp
Abstract: Globally rising demand for transportation by rail is pushing existing infrastructure to its capacity limits, necessitating the development of accurate, robust, and high-frequency positioning systems to ensure safe and efficient train operation. As individual sensor modalities cannot satisfy the strict requirements of robustness and safety, a combination thereof is required. We propose a path-constrained sensor fusion framework to integrate various modalities while leveraging the unique characteristics of the railway network. To reflect the constrained motion of rail vehicles along their tracks, the state is modeled in 1D along the track geometry. We further leverage the limited action space of a train by employing a novel multi-hypothesis tracking to account for multiple possible trajectories a vehicle can take through the railway network. We demonstrate the reliability and accuracy of our fusion framework on multiple tram datasets recorded in the city of Zurich, utilizing Visual-Inertial Odometry for local motion estimation and a standard GNSS for global localization. We evaluate our results using ground truth localizations recorded with a RTK-GNSS, and compare our method to standard baselines. A Root Mean Square Error of 4.78 m and a track selectivity score of up to 94.9 % have been achieved.
6.Trajectory Tracking Control of Dual-PAM Soft Actuator with Hysteresis Compensator
Authors:Junyi Shen, Tetsuro Miyazaki, Shingo Ohno, Maina Sogabe, Kenji Kawashima
Abstract: Soft robotics is an emergent and swiftly evolving field. Pneumatic actuators are suitable for driving soft robots because of their superior performance. However, their control is not easy due to their hysteresis characteristics. In response to these challenges, we propose an adaptive control method to compensate hysteresis of a soft actuator. Employing a novel dual pneumatic artificial muscle (PAM) bending actuator, the innovative control strategy abates hysteresis effects by dynamically modulating gains within a traditional PID controller corresponding with the predicted motion of the reference trajectory. Through comparative experimental evaluation, we found that the new control method outperforms its conventional counterparts regarding tracking accuracy and response speed. Our work reveals a new direction for advancing control in soft actuators.
7.Constrained Stein Variational Trajectory Optimization
Authors:Thomas Power, Dmitry Berenson
Abstract: We present Constrained Stein Variational Trajectory Optimization (CSVTO), an algorithm for performing trajectory optimization with constraints on a set of trajectories in parallel. We frame constrained trajectory optimization as a novel form of constrained functional minimization over trajectory distributions, which avoids treating the constraints as a penalty in the objective and allows us to generate diverse sets of constraint-satisfying trajectories. Our method uses Stein Variational Gradient Descent (SVGD) to find a set of particles that approximates a distribution over low-cost trajectories while obeying constraints. CSVTO is applicable to problems with arbitrary equality and inequality constraints and includes a novel particle resampling step to escape local minima. By explicitly generating diverse sets of trajectories, CSVTO is better able to avoid poor local minima and is more robust to initialization. We demonstrate that CSVTO outperforms baselines in challenging highly-constrained tasks, such as a 7DoF wrench manipulation task, where CSVTO succeeds in 20/20 trials vs 13/20 for the closest baseline. Our results demonstrate that generating diverse constraint-satisfying trajectories improves robustness to disturbances and initialization over baselines.
8.Multi-UAV Deployment in Obstacle-Cluttered Environments with LOS Connectivity
Authors:Yuda Chen, Meng Guo
Abstract: A reliable communication network is essential for multiple UAVs operating within obstacle-cluttered environments, where limited communication due to obstructions often occurs. A common solution is to deploy intermediate UAVs to relay information via a multi-hop network, which introduces two challenges: (i) how to design the structure of multi-hop networks; and (ii) how to maintain connectivity during collaborative motion. To this end, this work first proposes an efficient constrained search method based on the minimum-edge RRT$^\star$ algorithm, to find a spanning-tree topology that requires a less number of UAVs for the deployment task. To achieve this deployment, a distributed model predictive control strategy is proposed for the online motion coordination. It explicitly incorporates not only the inter-UAV and UAV-obstacle distance constraints, but also the line-of-sight (LOS) connectivity constraint. These constraints are well-known to be nonlinear and often tackled by various approximations. In contrast, this work provides a theoretical guarantee that all agent trajectories are ensured to be collision-free with a team-wise LOS connectivity at all time. Numerous simulations are performed in 3D valley-like environments, while hardware experiments validate its dynamic adaptation when the deployment position changes online.
9.In-Hand Cube Reconfiguration: Simplified
Authors:Sumit Patidar, Adrian Sieler, Oliver Brock
Abstract: We present a simple approach to in-hand cube reconfiguration. By simplifying planning, control, and perception as much as possible, while maintaining robust and general performance, we gain insights into the inherent complexity of in-hand cube reconfiguration. We also demonstrate the effectiveness of combining GOFAI-based planning with the exploitation of environmental constraints and inherently compliant end-effectors in the context of dexterous manipulation. The proposed system outperforms a substantially more complex system for cube reconfiguration based on deep learning and accurate physical simulation, contributing arguments to the discussion about what the most promising approach to general manipulation might be. Project website: https://rbo.gitlab-pages.tu-berlin.de/robotics/simpleIHM/
10.A Heuristic Informative-Path-Planning Algorithm for Autonomous Mapping of Unknown Areas
Authors:Mobolaji O. Orisatoki, Mahdi Amouzadi, Arash M. Dizqah
Abstract: Informative path planning algorithms are of paramount importance in applications like disaster management to efficiently gather information through a priori unknown environments. This is, however, a complex problem that involves finding a globally optimal path that gathers the maximum amount of information (e.g., the largest map with a minimum travelling distance) while using partial and uncertain local measurements. This paper addresses this problem by proposing a novel heuristic algorithm that continuously estimates the potential mapping gain for different sub-areas across the partially created map, and then uses these estimations to locally navigate the robot. Furthermore, this paper presents a novel algorithm to calculate a benchmark solution, where the map is a priori known to the planar, to evaluate the efficacy of the developed heuristic algorithm over different test scenarios. The findings indicate that the efficiency of the proposed algorithm, measured in terms of the mapped area per unit of travelling distance, ranges from 70% to 80% of the benchmark solution in various test scenarios. In essence, the algorithm demonstrates the capability to generate paths that come close to the globally optimal path provided by the benchmark solution.
11.Electromagnets Under the Table: an Unobtrusive Magnetic Navigation System for Microsurgery
Authors:Adam Schonewille, Changyan He, Cameron Forbrigger, Nancy Wu, James Drake, Thomas Looi, Eric Diller
Abstract: Miniature magnetic tools have the potential to enable minimally invasive surgical techniques to be applied to space-restricted surgical procedures in areas such as neurosurgery. However, typical magnetic navigation systems, which create the magnetic fields to drive such tools, either cannot generate large enough fields, or surround the patient in a way that obstructs surgeon access to the patient. This paper introduces the design of a magnetic navigation system with eight electromagnets arranged completely under the operating table, to endow the system with maximal workspace accessibility, which allows the patient to lie down on the top surface of the system without any constraints. The found optimal geometric layout of the electromagnets maximizes the field strength and uniformity over a reasonable neurosurgical operating volume. The system can generate non-uniform magnetic fields up to 38 mT along the x and y axes and 47 mT along the z axis at a working distance of 120 mm away from the actuation system workbench, deep enough to deploy magnetic microsurgical tools in the brain. The forces which can be exerted on millimeter-scale magnets used in prototype neurosurgical tools are validated experimentally. Due to its large workspace, this system could be used to control milli-robots in a variety of surgical applications.
12.NimbRo wins ANA Avatar XPRIZE Immersive Telepresence Competition: Human-Centric Evaluation and Lessons Learned
Authors:Christian Lenz, Max Schwarz, Andre Rochow, Bastian Pätzold, Raphael Memmesheimer, Michael Schreiber, Sven Behnke
Abstract: Robotic avatar systems can enable immersive telepresence with locomotion, manipulation, and communication capabilities. We present such an avatar system, based on the key components of immersive 3D visualization and transparent force-feedback telemanipulation. Our avatar robot features an anthropomorphic upper body with dexterous hands. The remote human operator drives the arms and fingers through an exoskeleton-based operator station, which provides force feedback both at the wrist and for each finger. The robot torso is mounted on a holonomic base, providing omnidirectional locomotion on flat floors, controlled using a 3D rudder device. Finally, the robot features a 6D movable head with stereo cameras, which stream images to a VR display worn by the operator. Movement latency is hidden using spherical rendering. The head also carries a telepresence screen displaying an animated image of the operator's face, enabling direct interaction with remote persons. Our system won the \$10M ANA Avatar XPRIZE competition, which challenged teams to develop intuitive and immersive avatar systems that could be operated by briefly trained judges. We analyze our successful participation in the semifinals and finals and provide insight into our operator training and lessons learned. In addition, we evaluate our system in a user study that demonstrates its intuitive and easy usability.
13.Operational requirements for localization in autonomous vehicles
Authors:Arpan Kusari, Satabdi Saha
Abstract: Autonomous vehicles (AVs) need to determine their position and orientation accurately with respect to global coordinate system or local features under different scene geometries, traffic conditions and environmental conditions. \cite{reid2019localization} provides a comprehensive framework for the localization requirements for AVs. However, the framework is too restrictive whereby - (a) only a very small deviation from the lane is tolerated (one every $10^{8}$ hours), (b) all roadway types are considered same without any attention to restriction provided by the environment onto the localization and (c) the temporal nature of the location and orientation is not considered in the requirements. In this research, we present a more practical view of the localization requirement aimed at keeping the AV safe during an operation. We present the following novel contributions - (a) we propose a deviation penalty as a cumulative distribution function of the Weibull distribution which starts from the adjacent lane boundary, (b) we customize the parameters of the deviation penalty according to the current roadway type, particular lane boundary that the ego vehicle is against and roadway curvature and (c) we update the deviation penalty based on the available gap in the adjacent lane. We postulate that this formulation can provide a more robust and achievable view of the localization requirements than previous research while focusing on safety.