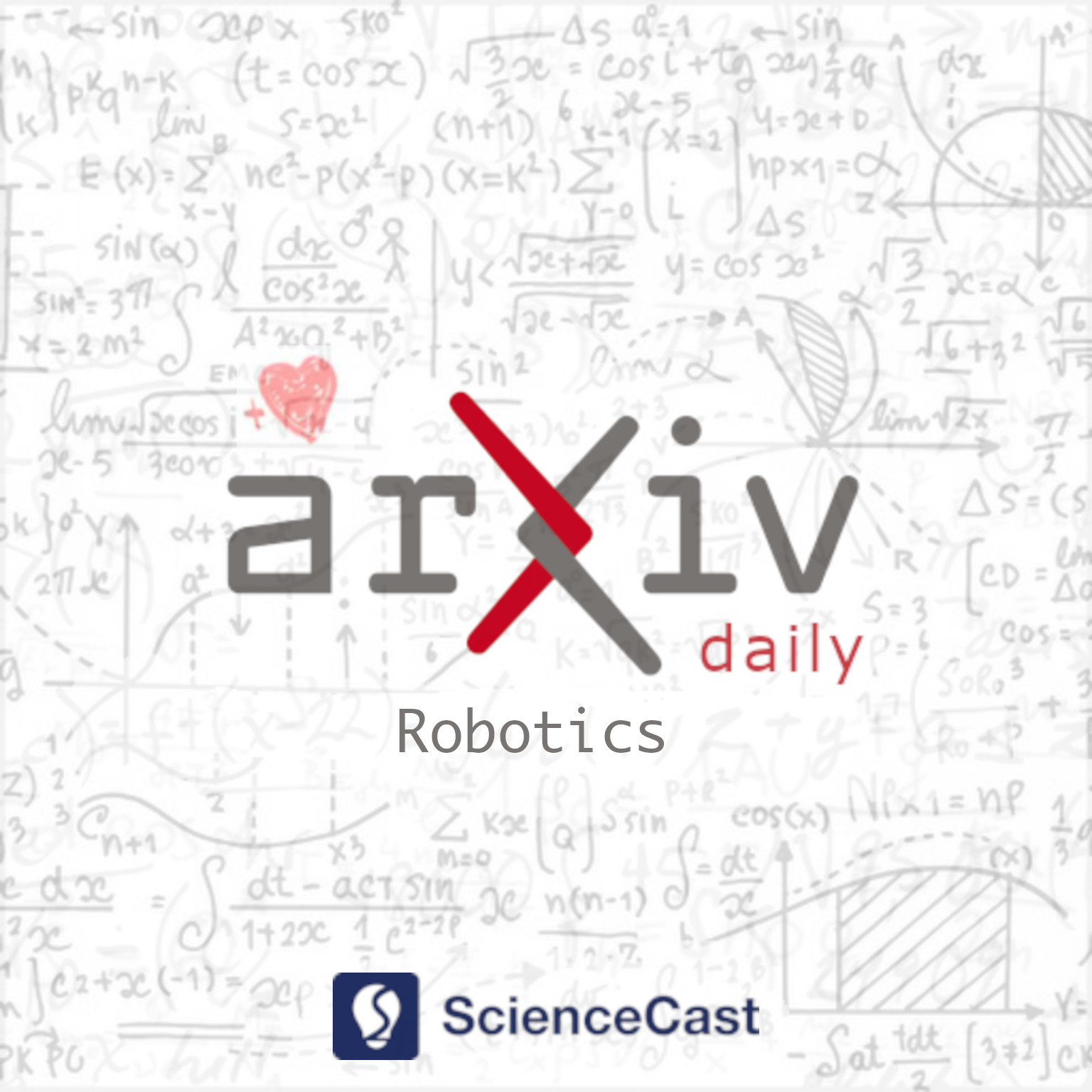
Robotics (cs.RO)
Fri, 30 Jun 2023
1.Decentralized Motor Skill Learning for Complex Robotic Systems
Authors:Yanjiang Guo, Zheyuan Jiang, Yen-Jen Wang, Jingyue Gao, Jianyu Chen
Abstract: Reinforcement learning (RL) has achieved remarkable success in complex robotic systems (eg. quadruped locomotion). In previous works, the RL-based controller was typically implemented as a single neural network with concatenated observation input. However, the corresponding learned policy is highly task-specific. Since all motors are controlled in a centralized way, out-of-distribution local observations can impact global motors through the single coupled neural network policy. In contrast, animals and humans can control their limbs separately. Inspired by this biological phenomenon, we propose a Decentralized motor skill (DEMOS) learning algorithm to automatically discover motor groups that can be decoupled from each other while preserving essential connections and then learn a decentralized motor control policy. Our method improves the robustness and generalization of the policy without sacrificing performance. Experiments on quadruped and humanoid robots demonstrate that the learned policy is robust against local motor malfunctions and can be transferred to new tasks.
2.Micromanipulation in Surgery: Autonomous Needle Insertion Inside the Eye for Targeted Drug Delivery
Authors:Ji Woong Kim, Peiyao Zhang, Peter Gehlbach, Iulian Iordachita, Marin Kobilarov
Abstract: We consider a micromanipulation problem in eye surgery, specifically retinal vein cannulation (RVC). RVC involves inserting a microneedle into a retinal vein for the purpose of targeted drug delivery. The procedure requires accurately guiding a needle to a target vein and inserting it while avoiding damage to the surrounding tissues. RVC can be considered similar to the reach or push task studied in robotics manipulation, but with additional constraints related to precision and safety while interacting with soft tissues. Prior works have mainly focused developing robotic hardware and sensors to enhance the surgeons' accuracy, leaving the automation of RVC largely unexplored. In this paper, we present the first autonomous strategy for RVC while relying on a minimal setup: a robotic arm, a needle, and monocular images. Our system exclusively relies on monocular vision to achieve precise navigation, gentle placement on the target vein, and safe insertion without causing tissue damage. Throughout the procedure, we employ machine learning for perception and to identify key surgical events such as needle-vein contact and vein punctures. Detecting these events guides our task and motion planning framework, which generates safe trajectories using model predictive control to complete the procedure. We validate our system through 24 successful autonomous trials on 4 cadaveric pig eyes. We show that our system can navigate to target veins within 22 micrometers of XY accuracy and under 35 seconds, and consistently puncture the target vein without causing tissue damage. Preliminary comparison to a human demonstrates the superior accuracy and reliability of our system.
3.Modeling and parametric optimization of 3D tendon-sheath actuator system for upper limb soft exosuit
Authors:Amit Yadav, Nitesh Kumar, Shaurya Surana, Lalan Kumar, Suriya Prakash Muthukrishnan, Shubhendu Bhasin, Sitikantha Roy
Abstract: This paper presents an analysis of parametric characterization of a motor driven tendon-sheath actuator system for use in upper limb augmentation for applications such as rehabilitation, therapy, and industrial automation. The double tendon sheath system, which uses two sets of cables (agonist and antagonist side) guided through a sheath, is considered to produce smooth and natural-looking movements of the arm. The exoskeleton is equipped with a single motor capable of controlling both the flexion and extension motions. One of the key challenges in the implementation of a double tendon sheath system is the possibility of slack in the tendon, which can impact the overall performance of the system. To address this issue, a robust mathematical model is developed and a comprehensive parametric study is carried out to determine the most effective strategies for overcoming the problem of slack and improving the transmission. The study suggests that incorporating a series spring into the system's tendon leads to a universally applicable design, eliminating the need for individual customization. The results also show that the slack in the tendon can be effectively controlled by changing the pretension, spring constant, and size and geometry of spool mounted on the axle of motor.
4.LIO-GVM: an Accurate, Tightly-Coupled Lidar-Inertial Odometry with Gaussian Voxel Map
Authors:Xingyu Ji, Shenghai Yuan, Pengyu Yin, Lihua Xie
Abstract: This letter presents an accurate and robust Lidar Inertial Odometry framework. We fuse LiDAR scans with IMU data using a tightly-coupled iterative error state Kalman filter for robust and fast localization. To achieve robust correspondence matching, we represent the points as a set of Gaussian distributions and evaluate the divergence in variance for outlier rejection. Based on the fitted distributions, a new residual metric is proposed for the filter-based Lidar inertial odometry, which demonstrates an improvement from merely quantifying distance to incorporating variance disparity, further enriching the comprehensiveness and accuracy of the residual metric. Due to the strategic design of the residual metric, we propose a simple yet effective voxel-solely mapping scheme, which only necessities the maintenance of one centroid and one covariance matrix for each voxel. Experiments on different datasets demonstrate the robustness and accuracy of our framework for various data inputs and environments. To the benefit of the robotics society, we open source the code at https://github.com/Ji1Xingyu/lio_gvm.
5.Human-like Decision-making at Unsignalized Intersection using Social Value Orientation
Authors:Yan Tong, Licheng Wen, Pinlong Cai, Daocheng Fu, Song Mao, Yikang Li
Abstract: With the commercial application of automated vehicles (AVs), the sharing of roads between AVs and human-driven vehicles (HVs) becomes a common occurrence in the future. While research has focused on improving the safety and reliability of autonomous driving, it's also crucial to consider collaboration between AVs and HVs. Human-like interaction is a required capability for AVs, especially at common unsignalized intersections, as human drivers of HVs expect to maintain their driving habits for inter-vehicle interactions. This paper uses the social value orientation (SVO) in the decision-making of vehicles to describe the social interaction among multiple vehicles. Specifically, we define the quantitative calculation of the conflict-involved SVO at unsignalized intersections to enhance decision-making based on the reinforcement learning method. We use naturalistic driving scenarios with highly interactive motions for performance evaluation of the proposed method. Experimental results show that SVO is more effective in characterizing inter-vehicle interactions than conventional motion state parameters like velocity, and the proposed method can accurately reproduce naturalistic driving trajectories compared to behavior cloning.
6.Locking On: Leveraging Dynamic Vehicle-Imposed Motion Constraints to Improve Visual Localization
Authors:Stephen Hausler, Sourav Garg, Punarjay Chakravarty, Shubham Shrivastava, Ankit Vora, Michael Milford
Abstract: Most 6-DoF localization and SLAM systems use static landmarks but ignore dynamic objects because they cannot be usefully incorporated into a typical pipeline. Where dynamic objects have been incorporated, typical approaches have attempted relatively sophisticated identification and localization of these objects, limiting their robustness or general utility. In this research, we propose a middle ground, demonstrated in the context of autonomous vehicles, using dynamic vehicles to provide limited pose constraint information in a 6-DoF frame-by-frame PnP-RANSAC localization pipeline. We refine initial pose estimates with a motion model and propose a method for calculating the predicted quality of future pose estimates, triggered based on whether or not the autonomous vehicle's motion is constrained by the relative frame-to-frame location of dynamic vehicles in the environment. Our approach detects and identifies suitable dynamic vehicles to define these pose constraints to modify a pose filter, resulting in improved recall across a range of localization tolerances from $0.25m$ to $5m$, compared to a state-of-the-art baseline single image PnP method and its vanilla pose filtering. Our constraint detection system is active for approximately $35\%$ of the time on the Ford AV dataset and localization is particularly improved when the constraint detection is active.
7.Fusion of Visual-Inertial Odometry with LiDAR Relative Localization for Cooperative Guidance of a Micro-Scale Aerial Vehicle
Authors:Václav Pritzl, Matouš Vrba, Petr Štěpán, Martin Saska
Abstract: A novel relative localization approach for cooperative guidance of a micro-scale Unmanned Aerial Vehicle (UAV) fusing Visual-Inertial Odometry (VIO) with Light Detection and Ranging (LiDAR) is proposed in this paper. LiDAR-based localization is accurate and robust to challenging environmental conditions, but 3D LiDARs are relatively heavy and require large UAV platforms. Visual cameras are cheap and lightweight. However, visual-based self-localization methods exhibit lower accuracy and can suffer from significant drift with respect to the global reference frame. We focus on cooperative navigation in a heterogeneous team of a primary LiDAR-equipped UAV and secondary camera-equipped UAV. We propose a novel cooperative approach combining LiDAR relative localization data with VIO output on board the primary UAV to obtain an accurate pose of the secondary UAV. The pose estimate is used to guide the secondary UAV along trajectories defined in the primary UAV reference frame. The experimental evaluation has shown the superior accuracy of our method to the raw VIO output and demonstrated its capability to guide the secondary UAV along desired trajectories while mitigating VIO drift.
8.Role of single particle motility statistics on efficiency of targeted delivery of micro-robot swarms
Authors:Akshatha Jagadish, Manoj Varma
Abstract: The study of dynamics of single active particles plays an important role in the development of artificial or hybrid micro-systems for bio-medical and other applications at micro-scale. Here, we utilize the results of these studies to better understand their implications for the specific application of drug delivery. We analyze the variations in the capture efficiency for different types of motion dynamics without inter-particle interactions and compare the results. We also discuss the reasons for the same and describe the specific parameters that affect the capture efficiency, which in turn helps in both hardware and control design of a micro-bot swarm system for drug delivery.
9.Unscented Optimal Control for 3D Coverage Planning with an Autonomous UAV Agent
Authors:Savvas Papaioannou, Panayiotis Kolios, Theocharis Theocharides, Christos G. Panayiotou, Marios M. Polycarpou
Abstract: We propose a novel probabilistically robust controller for the guidance of an unmanned aerial vehicle (UAV) in coverage planning missions, which can simultaneously optimize both the UAV's motion, and camera control inputs for the 3D coverage of a given object of interest. Specifically, the coverage planning problem is formulated in this work as an optimal control problem with logical constraints to enable the UAV agent to jointly: a) select a series of discrete camera field-of-view states which satisfy a set of coverage constraints, and b) optimize its motion control inputs according to a specified mission objective. We show how this hybrid optimal control problem can be solved with standard optimization tools by converting the logical expressions in the constraints into equality/inequality constraints involving only continuous variables. Finally, probabilistic robustness is achieved by integrating the unscented transformation to the proposed controller, thus enabling the design of robust open-loop coverage plans which take into account the future posterior distribution of the UAV's state inside the planning horizon.
10.Navigation of micro-robot swarms for targeted delivery using reinforcement learning
Authors:Akshatha Jagadish, Manoj Varma
Abstract: Micro robotics is quickly emerging to be a promising technological solution to many medical treatments with focus on targeted drug delivery. They are effective when working in swarms whose individual control is mostly infeasible owing to their minute size. Controlling a number of robots with a single controller is thus important and artificial intelligence can help us perform this task successfully. In this work, we use the Reinforcement Learning (RL) algorithms Proximal Policy Optimization (PPO) and Robust Policy Optimization (RPO) to navigate a swarm of 4, 9 and 16 microswimmers under hydrodynamic effects, controlled by their orientation, towards a circular absorbing target. We look at both PPO and RPO performances with limited state information scenarios and also test their robustness for random target location and size. We use curriculum learning to improve upon the performance and demonstrate the same in learning to navigate a swarm of 25 swimmers and steering the swarm to exemplify the manoeuvring capabilities of the RL model.
11.Mixed Integer Programming for Time-Optimal Multi-Robot Coverage Path Planning with Efficient Heuristics
Authors:Jingtao Tang, Hang Ma
Abstract: We investigate time-optimal Multi-Robot Coverage Path Planning (MCPP) for both unweighted and weighted terrains, which aims to minimize the coverage time, defined as the maximum travel time of all robots. Specifically, we focus on a reduction from MCPP to Rooted Min-Max Tree Cover (RMMTC). For the first time, we propose a Mixed Integer Programming (MIP) model to optimally solve RMMTC, resulting in an MCPP solution with a coverage time that is provably at most four times the optimal. Moreover, we propose two suboptimal yet effective heuristics that reduce the number of variables in the MIP model, thus improving its efficiency for large-scale MCPP instances. We show that both heuristics result in reduced-size MIP models that remain complete (i.e., guarantee to find a solution if one exists) for all RMMTC instances. Additionally, we explore the use of model optimization warm-startup to further improve the efficiency of both the original MIP model and the reduced-size MIP models. We validate the effectiveness of our MIP-based MCPP planner through experiments that compare it with two state-of-the-art MCPP planners on various instances, demonstrating a reduction in the coverage time by an average of 42.42% and 39.16% over them, respectively.
12.Projection-based first-order constrained optimization solver for robotics
Authors:Hakan Girgin, Tobias Löw, Teng Xue, Sylvain Calinon
Abstract: Robot programming tools ranging from inverse kinematics (IK) to model predictive control (MPC) are most often described as constrained optimization problems. Even though there are currently many commercially-available second-order solvers, robotics literature recently focused on efficient implementations and improvements over these solvers for real-time robotic applications. However, most often, these implementations stay problem-specific and are not easy to access or implement, or do not exploit the geometric aspect of the robotics problems. In this work, we propose to solve these problems using a fast, easy-to-implement first-order method that fully exploits the geometric constraints via Euclidean projections, called Augmented Lagrangian Spectral Projected Gradient Descent (ALSPG). We show that 1. using projections instead of full constraints and gradients improves the performance of the solver and 2. ALSPG stays competitive to the standard second-order methods such as iLQR in the unconstrained case. We showcase these results with IK and motion planning problems on simulated examples and with an MPC problem on a 7-axis manipulator experiment.
13.An Integrated FPGA Accelerator for Deep Learning-based 2D/3D Path Planning
Authors:Keisuke Sugiura, Hiroki Matsutani
Abstract: Path planning is a crucial component for realizing the autonomy of mobile robots. However, due to limited computational resources on mobile robots, it remains challenging to deploy state-of-the-art methods and achieve real-time performance. To address this, we propose P3Net (PointNet-based Path Planning Networks), a lightweight deep-learning-based method for 2D/3D path planning, and design an IP core (P3NetCore) targeting FPGA SoCs (Xilinx ZCU104). P3Net improves the algorithm and model architecture of the recently-proposed MPNet. P3Net employs an encoder with a PointNet backbone and a lightweight planning network in order to extract robust point cloud features and sample path points from a promising region. P3NetCore is comprised of the fully-pipelined point cloud encoder, batched bidirectional path planner, and parallel collision checker, to cover most part of the algorithm. On the 2D (3D) datasets, P3Net with the IP core runs 24.54-149.57x and 6.19-115.25x (10.03-59.47x and 3.38-28.76x) faster than ARM Cortex CPU and Nvidia Jetson while only consuming 0.255W (0.809W), and is up to 1049.42x (133.84x) power-efficient than the workstation. P3Net improves the success rate by up to 28.2% and plans a near-optimal path, leading to a significantly better tradeoff between computation and solution quality than MPNet and the state-of-the-art sampling-based methods.
14.Evaluation of the Benefits of Zero Velocity Update in Decentralized EKF-Based Cooperative Localization Algorithms for GNSS-Denied Multi-Robot Systems
Authors:Cagri Kilic, Eduardo Gutierrez, Jason N. Gross
Abstract: This paper proposes the cooperative use of zero velocity update (ZU) in a decentralized extended Kalman filter (DEKF) based localization algorithm for multi-robot systems. The filter utilizes inertial measurement unit (IMU), ultra-wideband (UWB), and odometry velocity measurements to improve the localization performance of the system in the presence of a GNSS-denied environment. The contribution of this work is to evaluate the benefits of using ZU in a DEKF-based localization algorithm. The algorithm is tested with real hardware in a video motion capture facility and a Robot Operating System (ROS) based simulation environment for unmanned ground vehicles (UGV). Both simulation and real-world experiments are performed to show the effectiveness of using ZU in one robot to reinstate the localization of other robots in a multi-robot system. Experimental results from GNSS-denied simulation and real-world environments show that using ZU with simple heuristics in the DEKF significantly improves the 3D localization accuracy.
15.The Bridge between Xsens Motion-Capture and Robot Operating System (ROS): Enabling Robots with Online 3D Human Motion Tracking
Authors:Mattia Leonori Human-Robot Interfaces and Interaction, Istituto Italiano di Tecnologia, Marta Lorenzini Human-Robot Interfaces and Interaction, Istituto Italiano di Tecnologia, Luca Fortini Human-Robot Interfaces and Interaction, Istituto Italiano di Tecnologia, Juan M. Gandarias Human-Robot Interfaces and Interaction, Istituto Italiano di Tecnologia, Arash Ajoudani Human-Robot Interfaces and Interaction, Istituto Italiano di Tecnologia
Abstract: This document introduces the bridge between the leading inertial motion-capture systems for 3D human tracking and the most used robotics software framework. 3D kinematic data provided by Xsens are translated into ROS messages to make them usable by robots and a Unified Robotics Description Format (URDF) model of the human kinematics is generated, which can be run and displayed in ROS 3D visualizer, RViz. The code to implement the to-ROS-bridge is a ROS package called xsens_mvn_ros and is available on GitHub at https://github.com/hrii-iit/xsens_mvn_ros The main documentation can be found at https://hrii-iit.github.io/xsens_mvn_ros/index.html
16.Zespol: A Lightweight Environment for Training Swarming Agents
Authors:Shay Snyder George Mason University, Kevin Zhu George Mason University, Ricardo Vega George Mason University, Cameron Nowzari George Mason University, Maryam Parsa George Mason University
Abstract: Agent-based modeling (ABM) and simulation have emerged as important tools for studying emergent behaviors, especially in the context of swarming algorithms for robotic systems. Despite significant research in this area, there is a lack of standardized simulation environments, which hinders the development and deployment of real-world robotic swarms. To address this issue, we present Zespol, a modular, Python-based simulation environment that enables the development and testing of multi-agent control algorithms. Zespol provides a flexible and extensible sandbox for initial research, with the potential for scaling to real-world applications. We provide a topological overview of the system and detailed descriptions of its plug-and-play elements. We demonstrate the fidelity of Zespol in simulated and real-word robotics by replicating existing works highlighting the simulation to real gap with the milling behavior. We plan to leverage Zespol's plug-and-play feature for neuromorphic computing in swarming scenarios, which involves using the modules in Zespol to simulate the behavior of neurons and their connections as synapses. This will enable optimizing and studying the emergent behavior of swarm systems in complex environments. Our goal is to gain a better understanding of the interplay between environmental factors and neural-like computations in swarming systems.
17.Act3D: Infinite Resolution Action Detection Transformer for Robotic Manipulation
Authors:Theophile Gervet, Zhou Xian, Nikolaos Gkanatsios, Katerina Fragkiadaki
Abstract: 3D perceptual representations are well suited for robot manipulation as they easily encode occlusions and simplify spatial reasoning. Many manipulation tasks require high spatial precision in end-effector pose prediction, typically demanding high-resolution 3D perceptual grids that are computationally expensive to process. As a result, most manipulation policies operate directly in 2D, foregoing 3D inductive biases. In this paper, we propose Act3D, a manipulation policy Transformer that casts 6-DoF keypose prediction as 3D detection with adaptive spatial computation. It takes as input 3D feature clouds unprojected from one or more camera views, iteratively samples 3D point grids in free space in a coarse-to-fine manner, featurizes them using relative spatial attention to the physical feature cloud, and selects the best feature point for end-effector pose prediction. Act3D sets a new state-of-the-art in RLbench, an established manipulation benchmark. Our model achieves 10% absolute improvement over the previous SOTA 2D multi-view policy on 74 RLbench tasks and 22% absolute improvement with 3x less compute over the previous SOTA 3D policy. In thorough ablations, we show the importance of relative spatial attention, large-scale vision-language pre-trained 2D backbones, and weight tying across coarse-to-fine attentions. Code and videos are available at our project site: https://act3d.github.io/.
18.Learning Evacuee Models from Robot-Guided Emergency Evacuation Experiments
Authors:Mollik Nayyar, Ghanghoon Paik, Zhenyuan Yuan, Tongjia Zheng, Minghui Zhu, Hai Lin, Alan R. Wagner
Abstract: Recent research has examined the possibility of using robots to guide evacuees to safe exits during emergencies. Yet, there are many factors that can impact a person's decision to follow a robot. Being able to model how an evacuee follows an emergency robot guide could be crucial for designing robots that effectively guide evacuees during an emergency. This paper presents a method for developing realistic and predictive human evacuee models from physical human evacuation experiments. The paper analyzes the behavior of 14 human subjects during physical robot-guided evacuation. We then use the video data to create evacuee motion models that predict the person's future positions during the emergency. Finally, we validate the resulting models by running a k-fold cross-validation on the data collected during physical human subject experiments. We also present performance results of the model using data from a similar simulated emergency evacuation experiment demonstrating that these models can serve as a tool to predict evacuee behavior in novel evacuation simulations.
19.Statler: State-Maintaining Language Models for Embodied Reasoning
Authors:Takuma Yoneda, Jiading Fang, Peng Li, Huanyu Zhang, Tianchong Jiang, Shengjie Lin, Ben Picker, David Yunis, Hongyuan Mei, Matthew R. Walter
Abstract: Large language models (LLMs) provide a promising tool that enable robots to perform complex robot reasoning tasks. However, the limited context window of contemporary LLMs makes reasoning over long time horizons difficult. Embodied tasks such as those that one might expect a household robot to perform typically require that the planner consider information acquired a long time ago (e.g., properties of the many objects that the robot previously encountered in the environment). Attempts to capture the world state using an LLM's implicit internal representation is complicated by the paucity of task- and environment-relevant information available in a robot's action history, while methods that rely on the ability to convey information via the prompt to the LLM are subject to its limited context window. In this paper, we propose Statler, a framework that endows LLMs with an explicit representation of the world state as a form of ``memory'' that is maintained over time. Integral to Statler is its use of two instances of general LLMs -- a world-model reader and a world-model writer -- that interface with and maintain the world state. By providing access to this world state ``memory'', Statler improves the ability of existing LLMs to reason over longer time horizons without the constraint of context length. We evaluate the effectiveness of our approach on three simulated table-top manipulation domains and a real robot domain, and show that it improves the state-of-the-art in LLM-based robot reasoning. Project website: https://statler-lm.github.io/
20.GIRA: Gaussian Mixture Models for Inference and Robot Autonomy
Authors:Kshitij Goel, Wennie Tabib
Abstract: Large-scale deployments of robot teams are challenged by the need to share high-resolution perceptual information over low-bandwidth communication channels. Individual size, weight, and power constrained robots rely on environment models to assess navigability and safely traverse unstructured and complex environments. State of the art perception frameworks construct these models via multiple disparate pipelines that reuse the same underlying sensor data, which leads to increased computation, redundancy, and complexity. To bridge this gap, this paper introduces GIRA -- an open-source framework for compact, high-resolution environment modeling using Gaussian mixture models (GMMs). GIRA provides fundamental robotics capabilities such as high-fidelity reconstruction, pose estimation, and occupancy modeling in a single continuous representation.
21.Parallel Self-assembly for a Multi-USV System on Water Surface with Obstacles
Authors:Lianxin Zhang, Yihan Huang, Zhongzhong Cao, Yang Jiao, Huihuan Qian
Abstract: Parallel self-assembly is an efficient approach to accelerate the assembly process for modular robots. However, these approaches cannot accommodate complicated environments with obstacles, which restricts their applications. This paper considers the surrounding stationary obstacles and proposes a parallel self-assembly planning algorithm named SAPOA. With this algorithm, modular robots can avoid immovable obstacles when performing docking actions, which adapts the parallel self-assembly process to complex scenes. To validate the efficiency and scalability, we have designed 25 distinct grid maps with different obstacle configurations to simulate the algorithm. From the results compared to the existing parallel self-assembly algorithms, our algorithm shows a significantly higher success rate, which is more than 80%. For verification in real-world applications, a multi-agent hardware testbed system is developed. The algorithm is successfully deployed on four omnidirectional unmanned surface vehicles, CuBoats. The navigation strategy that translates the discrete planner, SAPOA, to the continuous controller on the CuBoats is presented. The algorithm's feasibility and flexibility were demonstrated through successful self-assembly experiments on 5 maps with varying obstacle configurations.
22.Collapse of Straight Soft Growing Inflated Beam Robots Under Their Own Weight
Authors:Ciera McFarland, Margaret M. Coad
Abstract: Soft, growing inflated beam robots, also known as everting vine robots, have previously been shown to navigate confined spaces with ease. Less is known about their ability to navigate three-dimensional open spaces where they have the potential to collapse under their own weight as they attempt to move through a space. Previous work has studied collapse of inflated beams and vine robots due to purely transverse or purely axial external loads. Here, we extend previous models to predict the length at which straight vine robots will collapse under their own weight at arbitrary launch angle relative to gravity, inflated diameter, and internal pressure. Our model successfully predicts the general trends of collapse behavior of straight vine robots. We find that collapse length increases non-linearly with the robot's launch angle magnitude, linearly with the robot's diameter, and with the square root of the robot's internal pressure. We also demonstrate the use of our model to determine the robot parameters required to grow a vine robot across a gap in the floor. This work forms the foundation of an approach for modeling the collapse of vine robots and inflated beams in arbitrary shapes.
23.A Personalized Household Assistive Robot that Learns and Creates New Breakfast Options through Human-Robot Interaction
Authors:Ali Ayub, Chrystopher L. Nehaniv, Kerstin Dautenhahn
Abstract: For robots to assist users with household tasks, they must first learn about the tasks from the users. Further, performing the same task every day, in the same way, can become boring for the robot's user(s), therefore, assistive robots must find creative ways to perform tasks in the household. In this paper, we present a cognitive architecture for a household assistive robot that can learn personalized breakfast options from its users and then use the learned knowledge to set up a table for breakfast. The architecture can also use the learned knowledge to create new breakfast options over a longer period of time. The proposed cognitive architecture combines state-of-the-art perceptual learning algorithms, computational implementation of cognitive models of memory encoding and learning, a task planner for picking and placing objects in the household, a graphical user interface (GUI) to interact with the user and a novel approach for creating new breakfast options using the learned knowledge. The architecture is integrated with the Fetch mobile manipulator robot and validated, as a proof-of-concept system evaluation in a large indoor environment with multiple kitchen objects. Experimental results demonstrate the effectiveness of our architecture to learn personalized breakfast options from the user and generate new breakfast options never learned by the robot.
24.Goal Representations for Instruction Following: A Semi-Supervised Language Interface to Control
Authors:Vivek Myers, Andre He, Kuan Fang, Homer Walke, Philippe Hansen-Estruch, Ching-An Cheng, Mihai Jalobeanu, Andrey Kolobov, Anca Dragan, Sergey Levine
Abstract: Our goal is for robots to follow natural language instructions like "put the towel next to the microwave." But getting large amounts of labeled data, i.e. data that contains demonstrations of tasks labeled with the language instruction, is prohibitive. In contrast, obtaining policies that respond to image goals is much easier, because any autonomous trial or demonstration can be labeled in hindsight with its final state as the goal. In this work, we contribute a method that taps into joint image- and goal- conditioned policies with language using only a small amount of language data. Prior work has made progress on this using vision-language models or by jointly training language-goal-conditioned policies, but so far neither method has scaled effectively to real-world robot tasks without significant human annotation. Our method achieves robust performance in the real world by learning an embedding from the labeled data that aligns language not to the goal image, but rather to the desired change between the start and goal images that the instruction corresponds to. We then train a policy on this embedding: the policy benefits from all the unlabeled data, but the aligned embedding provides an interface for language to steer the policy. We show instruction following across a variety of manipulation tasks in different scenes, with generalization to language instructions outside of the labeled data. Videos and code for our approach can be found on our website: http://tiny.cc/grif .
25.How Do Human Users Teach a Continual Learning Robot in Repeated Interactions?
Authors:Ali Ayub, Jainish Mehta, Zachary De Francesco, Patrick Holthaus, Kerstin Dautenhahn, Chrystopher L. Nehaniv
Abstract: Continual learning (CL) has emerged as an important avenue of research in recent years, at the intersection of Machine Learning (ML) and Human-Robot Interaction (HRI), to allow robots to continually learn in their environments over long-term interactions with humans. Most research in continual learning, however, has been robot-centered to develop continual learning algorithms that can quickly learn new information on static datasets. In this paper, we take a human-centered approach to continual learning, to understand how humans teach continual learning robots over the long term and if there are variations in their teaching styles. We conducted an in-person study with 40 participants that interacted with a continual learning robot in 200 sessions. In this between-participant study, we used two different CL models deployed on a Fetch mobile manipulator robot. An extensive qualitative and quantitative analysis of the data collected in the study shows that there is significant variation among the teaching styles of individual users indicating the need for personalized adaptation to their distinct teaching styles. The results also show that although there is a difference in the teaching styles between expert and non-expert users, the style does not have an effect on the performance of the continual learning robot. Finally, our analysis shows that the constrained experimental setups that have been widely used to test most continual learning techniques are not adequate, as real users interact with and teach continual learning robots in a variety of ways. Our code is available at https://github.com/aliayub7/cl_hri.
26.RObotic MAnipulation Network (ROMAN) $\unicode{x2013}$ Hybrid Hierarchical Learning for Solving Complex Sequential Tasks
Authors:Eleftherios Triantafyllidis, Fernando Acero, Zhaocheng Liu, Zhibin Li
Abstract: Solving long sequential tasks poses a significant challenge in embodied artificial intelligence. Enabling a robotic system to perform diverse sequential tasks with a broad range of manipulation skills is an active area of research. In this work, we present a Hybrid Hierarchical Learning framework, the Robotic Manipulation Network (ROMAN), to address the challenge of solving multiple complex tasks over long time horizons in robotic manipulation. ROMAN achieves task versatility and robust failure recovery by integrating behavioural cloning, imitation learning, and reinforcement learning. It consists of a central manipulation network that coordinates an ensemble of various neural networks, each specialising in distinct re-combinable sub-tasks to generate their correct in-sequence actions for solving complex long-horizon manipulation tasks. Experimental results show that by orchestrating and activating these specialised manipulation experts, ROMAN generates correct sequential activations for accomplishing long sequences of sophisticated manipulation tasks and achieving adaptive behaviours beyond demonstrations, while exhibiting robustness to various sensory noises. These results demonstrate the significance and versatility of ROMAN's dynamic adaptability featuring autonomous failure recovery capabilities, and highlight its potential for various autonomous manipulation tasks that demand adaptive motor skills.
27.Vision-based Oxy-fuel Torch Control for Robotic Metal Cutting
Authors:James Akl, Yash Patil, Chinmay Todankar, Berk Calli
Abstract: The automation of key processes in metal cutting would substantially benefit many industries such as manufacturing and metal recycling. We present a vision-based control scheme for automated metal cutting with oxy-fuel torches, an established cutting medium in industry. The system consists of a robot equipped with a cutting torch and an eye-in-hand camera observing the scene behind a tinted visor. We develop a vision-based control algorithm to servo the torch's motion by visually observing its effects on the metal surface. As such, the vision system processes the metal surface's heat pool and computes its associated features, specifically pool convexity and intensity, which are then used for control. The operating conditions of the control problem are defined within which the stability is proven. In addition, metal cutting experiments are performed using a physical 1-DOF robot and oxy-fuel cutting equipment. Our results demonstrate the successful cutting of metal plates across three different plate thicknesses, relying purely on visual information without a priori knowledge of the thicknesses.
28.Modeling, Characterization, and Control of Bacteria-inspired Bi-flagellated Mechanism with Tumbling
Authors:Zhuonan Hao, Sangmin Lim, M. Khalid Jawed
Abstract: Multi-flagellated bacteria utilize the hydrodynamic interaction between their filamentary tails, known as flagella, to swim and change their swimming direction in low Reynolds number flow. This interaction, referred to as bundling and tumbling, is often overlooked in simplified hydrodynamic models such as Resistive Force Theories (RFT). However, for the development of efficient and steerable robots inspired by bacteria, it becomes crucial to exploit this interaction. In this paper, we present the construction of a macroscopic bio-inspired robot featuring two rigid flagella arranged as right-handed helices, along with a cylindrical head. By rotating the flagella in opposite directions, the robot's body can reorient itself through repeatable and controllable tumbling. To accurately model this bi-flagellated mechanism in low Reynolds flow, we employ a coupling of rigid body dynamics and the method of Regularized Stokeslet Segments (RSS). Unlike RFT, RSS takes into account the hydrodynamic interaction between distant filamentary structures. Furthermore, we delve into the exploration of the parameter space to optimize the propulsion and torque of the system. To achieve the desired reorientation of the robot, we propose a tumble control scheme that involves modulating the rotation direction and speed of the two flagella. By implementing this scheme, the robot can effectively reorient itself to attain the desired attitude. Notably, the overall scheme boasts a simplified design and control as it only requires two control inputs. With our macroscopic framework serving as a foundation, we envision the eventual miniaturization of this technology to construct mobile and controllable micro-scale bacterial robots.