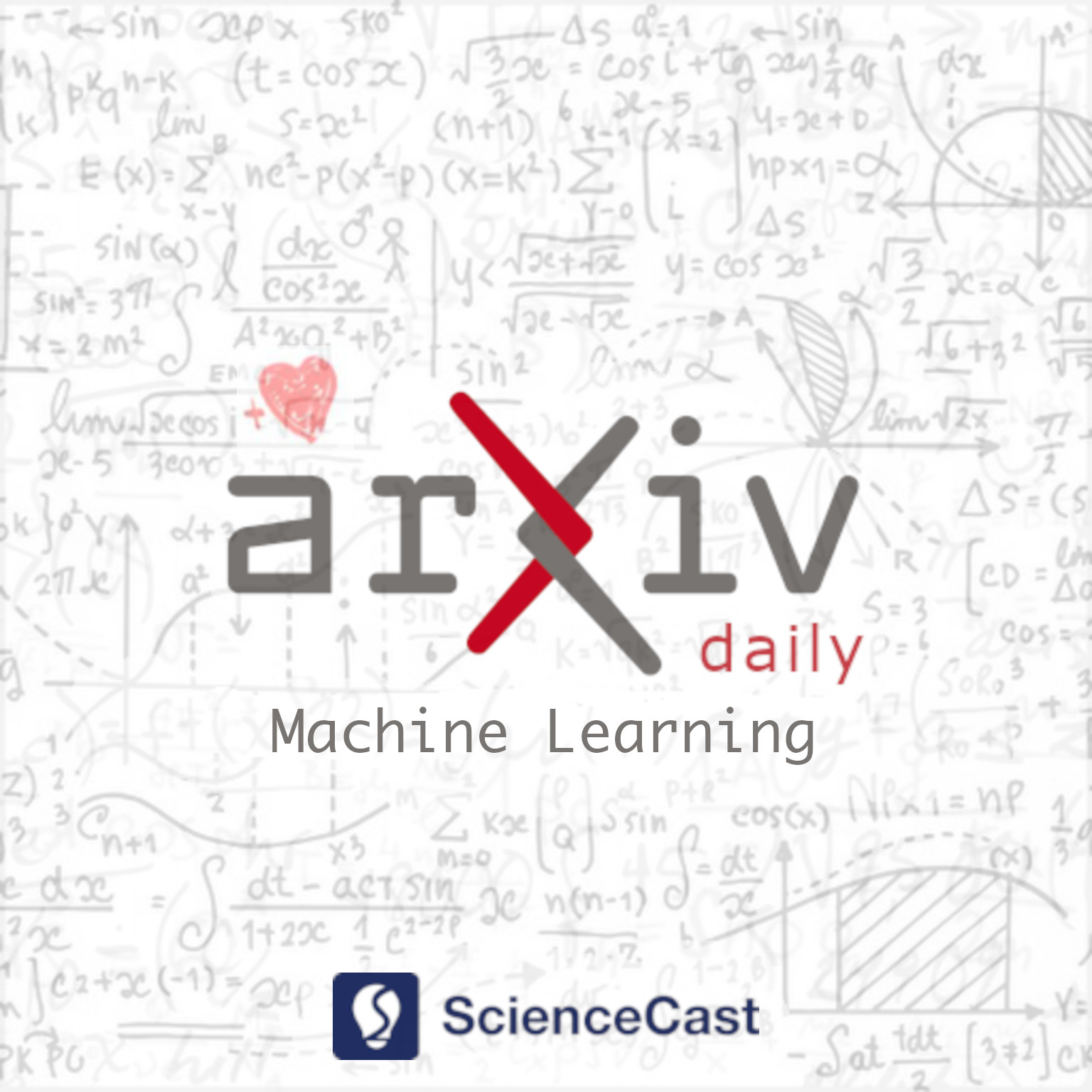
Machine Learning (stat.ML)
Wed, 10 May 2023
1.Fair principal component analysis (PCA): minorization-maximization algorithms for Fair PCA, Fair Robust PCA and Fair Sparse PCA
Authors:Prabhu Babu, Petre Stoica
Abstract: In this paper we propose a new iterative algorithm to solve the fair PCA (FPCA) problem. We start with the max-min fair PCA formulation originally proposed in [1] and derive a simple and efficient iterative algorithm which is based on the minorization-maximization (MM) approach. The proposed algorithm relies on the relaxation of a semi-orthogonality constraint which is proved to be tight at every iteration of the algorithm. The vanilla version of the proposed algorithm requires solving a semi-definite program (SDP) at every iteration, which can be further simplified to a quadratic program by formulating the dual of the surrogate maximization problem. We also propose two important reformulations of the fair PCA problem: a) fair robust PCA -- which can handle outliers in the data, and b) fair sparse PCA -- which can enforce sparsity on the estimated fair principal components. The proposed algorithms are computationally efficient and monotonically increase their respective design objectives at every iteration. An added feature of the proposed algorithms is that they do not require the selection of any hyperparameter (except for the fair sparse PCA case where a penalty parameter that controls the sparsity has to be chosen by the user). We numerically compare the performance of the proposed methods with two of the state-of-the-art approaches on synthetic data sets and a real-life data set.
2.Penalized deep neural networks estimator with general loss functions under weak dependence
Authors:William Kengne, Modou Wade
Abstract: This paper carries out sparse-penalized deep neural networks predictors for learning weakly dependent processes, with a broad class of loss functions. We deal with a general framework that includes, regression estimation, classification, times series prediction, $\cdots$ The $\psi$-weak dependence structure is considered, and for the specific case of bounded observations, $\theta_\infty$-coefficients are also used. In this case of $\theta_\infty$-weakly dependent, a non asymptotic generalization bound within the class of deep neural networks predictors is provided. For learning both $\psi$ and $\theta_\infty$-weakly dependent processes, oracle inequalities for the excess risk of the sparse-penalized deep neural networks estimators are established. When the target function is sufficiently smooth, the convergence rate of these excess risk is close to $\mathcal{O}(n^{-1/3})$. Some simulation results are provided, and application to the forecast of the particulate matter in the Vit\'{o}ria metropolitan area is also considered.