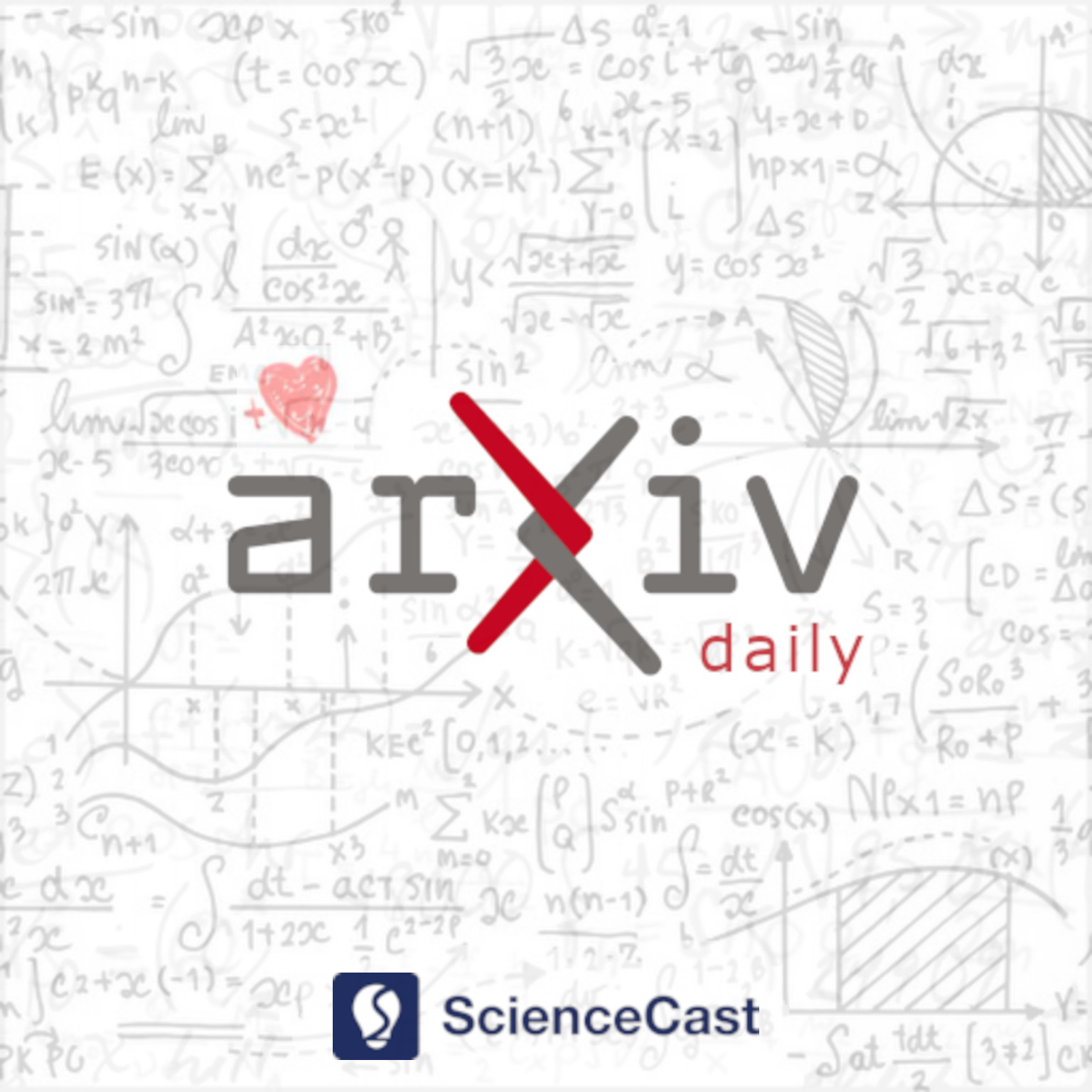
Image and Video Processing (eess.IV)
Mon, 11 Sep 2023
1.AutoFuse: Automatic Fusion Networks for Deformable Medical Image Registration
Authors:Mingyuan Meng, Michael Fulham, Dagan Feng, Lei Bi, Jinman Kim
Abstract: Deformable image registration aims to find a dense non-linear spatial correspondence between a pair of images, which is a crucial step for many medical tasks such as tumor growth monitoring and population analysis. Recently, Deep Neural Networks (DNNs) have been widely recognized for their ability to perform fast end-to-end registration. However, DNN-based registration needs to explore the spatial information of each image and fuse this information to characterize spatial correspondence. This raises an essential question: what is the optimal fusion strategy to characterize spatial correspondence? Existing fusion strategies (e.g., early fusion, late fusion) were empirically designed to fuse information by manually defined prior knowledge, which inevitably constrains the registration performance within the limits of empirical designs. In this study, we depart from existing empirically-designed fusion strategies and develop a data-driven fusion strategy for deformable image registration. To achieve this, we propose an Automatic Fusion network (AutoFuse) that provides flexibility to fuse information at many potential locations within the network. A Fusion Gate (FG) module is also proposed to control how to fuse information at each potential network location based on training data. Our AutoFuse can automatically optimize its fusion strategy during training and can be generalizable to both unsupervised registration (without any labels) and semi-supervised registration (with weak labels provided for partial training data). Extensive experiments on two well-benchmarked medical registration tasks (inter- and intra-patient registration) with eight public datasets show that our AutoFuse outperforms state-of-the-art unsupervised and semi-supervised registration methods.
2.Two-Stage Hybrid Supervision Framework for Fast, Low-resource, and Accurate Organ and Pan-cancer Segmentation in Abdomen CT
Authors:Wentao Liu, Tong Tian, Weijin Xu, Lemeng Wang, Haoyuan Li, Huihua Yang
Abstract: Abdominal organ and tumour segmentation has many important clinical applications, such as organ quantification, surgical planning, and disease diagnosis. However, manual assessment is inherently subjective with considerable inter- and intra-expert variability. In the paper, we propose a hybrid supervised framework, StMt, that integrates self-training and mean teacher for the segmentation of abdominal organs and tumors using partially labeled and unlabeled data. We introduce a two-stage segmentation pipeline and whole-volume-based input strategy to maximize segmentation accuracy while meeting the requirements of inference time and GPU memory usage. Experiments on the validation set of FLARE2023 demonstrate that our method achieves excellent segmentation performance as well as fast and low-resource model inference. Our method achieved an average DSC score of 89.79\% and 45.55 \% for the organs and lesions on the validation set and the average running time and area under GPU memory-time cure are 11.25s and 9627.82MB, respectively.
3.Treatment-aware Diffusion Probabilistic Model for Longitudinal MRI Generation and Diffuse Glioma Growth Prediction
Authors:Qinghui Liu, Elies Fuster-Garcia, Ivar Thokle Hovden, Donatas Sederevicius, Karoline Skogen, Bradley J MacIntosh, Edvard Grødem, Till Schellhorn, Petter Brandal, Atle Bjørnerud, Kyrre Eeg Emblem
Abstract: Diffuse gliomas are malignant brain tumors that grow widespread through the brain. The complex interactions between neoplastic cells and normal tissue, as well as the treatment-induced changes often encountered, make glioma tumor growth modeling challenging. In this paper, we present a novel end-to-end network capable of generating future tumor masks and realistic MRIs of how the tumor will look at any future time points for different treatment plans. Our model is built upon cutting-edge diffusion probabilistic models and deep-segmentation neural networks. We extended a diffusion model to include sequential multi-parametric MRI and treatment information as conditioning input to guide the generative diffusion process. This allows us to estimate tumor growth at any given time point. We trained the model using real-world postoperative longitudinal MRI data with glioma tumor growth trajectories represented as tumor segmentation maps over time. The model has demonstrated promising performance across a range of tasks, including the generation of high-quality synthetic MRIs with tumor masks, time-series tumor segmentations, and uncertainty estimation. Combined with the treatment-aware generated MRIs, the tumor growth predictions with uncertainty estimates can provide useful information for clinical decision-making.
4.A Localization-to-Segmentation Framework for Automatic Tumor Segmentation in Whole-Body PET/CT Images
Authors:Linghan Cai, Jianhao Huang, Zihang Zhu, Jinpeng Lu, Yongbing Zhang
Abstract: Fluorodeoxyglucose (FDG) positron emission tomography(PET) combined with computed tomography (CT) is considered the primary solution for detecting some cancers, such as lung cancer and melanoma. Automatic segmentation of tumors in PET/CT images can help reduce doctors' workload, thereby improving diagnostic quality. However, precise tumor segmentation is challenging due to the small size of many tumors and the similarity of high-uptake normal areas to the tumor regions. To address these issues, this paper proposes a localization-to-segmentation framework (L2SNet) for precise tumor segmentation. L2SNet first localizes the possible lesions in the lesion localization phase and then uses the location cues to shape the segmentation results in the lesion segmentation phase. To further improve the segmentation performance of L2SNet, we design an adaptive threshold scheme that takes the segmentation results of the two phases into consideration. The experiments with the MICCAI 2023 Automated Lesion Segmentation in Whole-Body FDG-PET/CT challenge dataset show that our method achieved a competitive result and was ranked in the top 7 methods on the preliminary test set. Our work is available at: https://github.com/MedCAI/L2SNet.