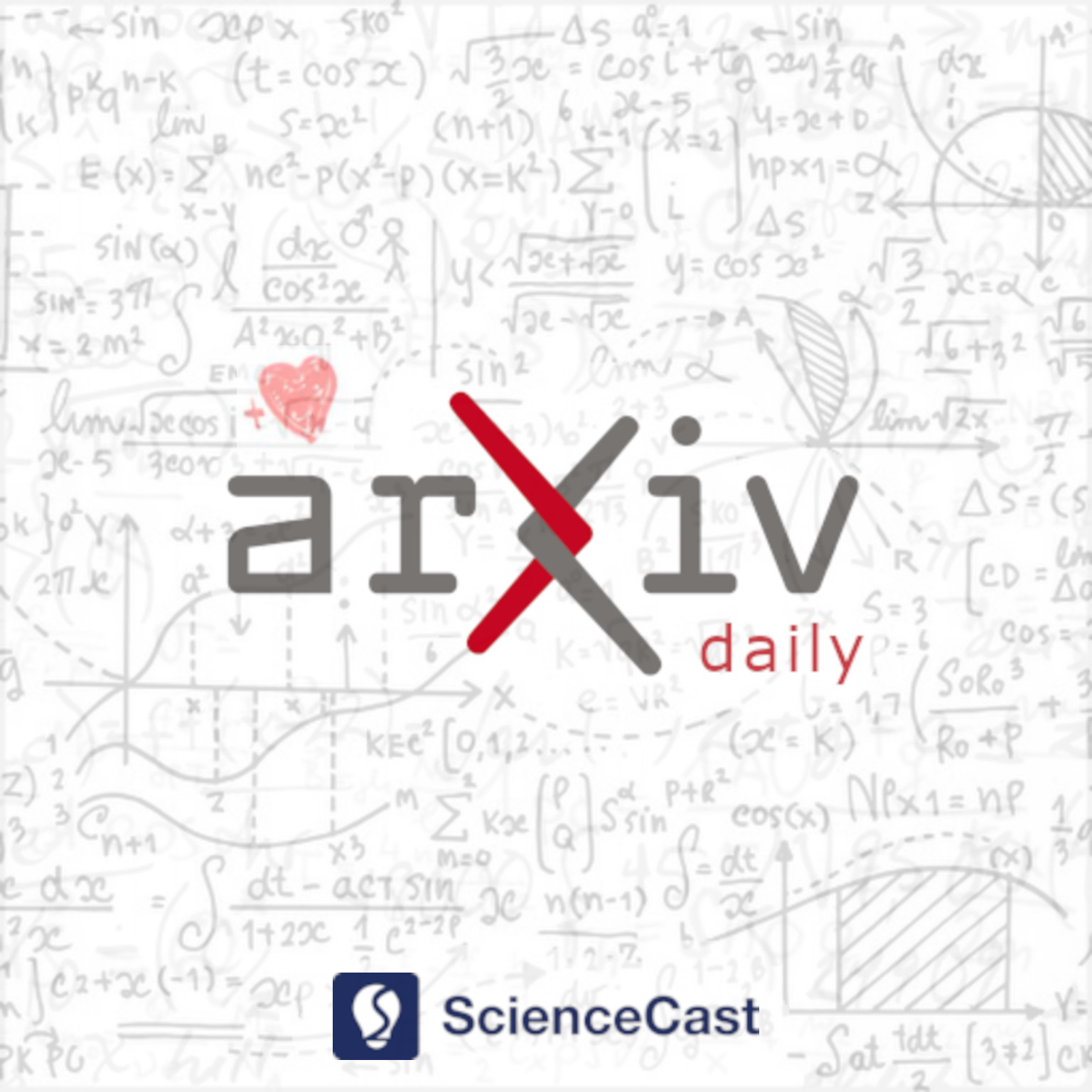
Image and Video Processing (eess.IV)
Wed, 30 Aug 2023
1.ACNPU: A 4.75TOPS/W 1080P@30FPS Super Resolution Accelerator with Decoupled Asymmetric Convolution
Authors:Tun-Hao Yang, Tian-Sheuan Chang
Abstract: Deep learning-driven superresolution (SR) outperforms traditional techniques but also faces the challenge of high complexity and memory bandwidth. This challenge leads many accelerators to opt for simpler and shallow models like FSRCNN, compromising performance for real-time needs, especially for resource-limited edge devices. This paper proposes an energy-efficient SR accelerator, ACNPU, to tackle this challenge. The ACNPU enhances image quality by 0.34dB with a 27-layer model, but needs 36\% less complexity than FSRCNN, while maintaining a similar model size, with the \textit{decoupled asymmetric convolution and split-bypass structure}. The hardware-friendly 17K-parameter model enables \textit{holistic model fusion} instead of localized layer fusion to remove external DRAM access of intermediate feature maps. The on-chip memory bandwidth is further reduced with the \textit{input stationary flow} and \textit{parallel-layer execution} to reduce power consumption. Hardware is regular and easy to control to support different layers by \textit{processing elements (PEs) clusters with reconfigurable input and uniform data flow}. The implementation in the 40 nm CMOS process consumes 2333 K gate counts and 198KB SRAMs. The ACNPU achieves 31.7 FPS and 124.4 FPS for x2 and x4 scales Full-HD generation, respectively, which attains 4.75 TOPS/W energy efficiency.
2.AMDNet23: A combined deep Contour-based Convolutional Neural Network and Long Short Term Memory system to diagnose Age-related Macular Degeneration
Authors:Md. Aiyub Ali, Md. Shakhawat Hossain, Md. Kawar Hossain, Subhadra Soumi Sikder, Sharun Akter Khushbu, Mirajul Islam
Abstract: In light of the expanding population, an automated framework of disease detection can assist doctors in the diagnosis of ocular diseases, yields accurate, stable, rapid outcomes, and improves the success rate of early detection. The work initially intended the enhancing the quality of fundus images by employing an adaptive contrast enhancement algorithm (CLAHE) and Gamma correction. In the preprocessing techniques, CLAHE elevates the local contrast of the fundus image and gamma correction increases the intensity of relevant features. This study operates on a AMDNet23 system of deep learning that combined the neural networks made up of convolutions (CNN) and short-term and long-term memory (LSTM) to automatically detect aged macular degeneration (AMD) disease from fundus ophthalmology. In this mechanism, CNN is utilized for extracting features and LSTM is utilized to detect the extracted features. The dataset of this research is collected from multiple sources and afterward applied quality assessment techniques, 2000 experimental fundus images encompass four distinct classes equitably. The proposed hybrid deep AMDNet23 model demonstrates to detection of AMD ocular disease and the experimental result achieved an accuracy 96.50%, specificity 99.32%, sensitivity 96.5%, and F1-score 96.49.0%. The system achieves state-of-the-art findings on fundus imagery datasets to diagnose AMD ocular disease and findings effectively potential of our method.
3.Research on Image Stitching Based on Invariant Features of Reconstructed Plane
Authors:Qi Liu, Xiyu Tang, Ju Huo
Abstract: Generating high-quality stitched images is a challenging task in computer vision. The existing feature-based image stitching methods commonly only focus on point and line features, neglecting the crucial role of higher-level planar features in image stitching. This paper proposes an image stitching method based on invariant planar features, which uses planar features as constraints to improve the overall effect of natural image stitching. Initially, our approach expands the quantity of matched feature points and lines through straight-line procedures, advancing alignment quality and reducing artifacts in overlapping areas. Then, uncertain planes are described by known matching points and matching lines, and plane features are introduced to preserve energy items, which improves the overall appearance of stitched images while reducing distortion and guarantees a more natural stitched image. Furthermore, to demonstrate the superiority of our approach, we also propose several evaluation indexes related to planar features to quantify the detailed changes of planar features. An extensive set of experiments validates the effectiveness of our approach in stitching natural images with a larger field of view. Compared with the most advanced methods, our method retains the texture and structure of the image better, and produces less unnatural distortion. Multiple quantitative evaluations illustrate that our approach outperforms existing methods with significant improvements, further validating the effectiveness and superiority of our proposed method.
4.Interpretability-guided Data Augmentation for Robust Segmentation in Multi-centre Colonoscopy Data
Authors:Valentina Corbetta, Regina Beets-Tan, Wilson Silva
Abstract: Multi-centre colonoscopy images from various medical centres exhibit distinct complicating factors and overlays that impact the image content, contingent on the specific acquisition centre. Existing Deep Segmentation networks struggle to achieve adequate generalizability in such data sets, and the currently available data augmentation methods do not effectively address these sources of data variability. As a solution, we introduce an innovative data augmentation approach centred on interpretability saliency maps, aimed at enhancing the generalizability of Deep Learning models within the realm of multi-centre colonoscopy image segmentation. The proposed augmentation technique demonstrates increased robustness across different segmentation models and domains. Thorough testing on a publicly available multi-centre dataset for polyp detection demonstrates the effectiveness and versatility of our approach, which is observed both in quantitative and qualitative results. The code is publicly available at: https://github.com/nki-radiology/interpretability_augmentation
5.Attention-based CT Scan Interpolation for Lesion Segmentation of Colorectal Liver Metastases
Authors:Mohammad Hamghalam, Richard K. G. Do, Amber L. Simpson
Abstract: Small liver lesions common to colorectal liver metastases (CRLMs) are challenging for convolutional neural network (CNN) segmentation models, especially when we have a wide range of slice thicknesses in the computed tomography (CT) scans. Slice thickness of CT images may vary by clinical indication. For example, thinner slices are used for presurgical planning when fine anatomic details of small vessels are required. While keeping the effective radiation dose in patients as low as possible, various slice thicknesses are employed in CRLMs due to their limitations. However, differences in slice thickness across CTs lead to significant performance degradation in CT segmentation models based on CNNs. This paper proposes a novel unsupervised attention-based interpolation model to generate intermediate slices from consecutive triplet slices in CT scans. We integrate segmentation loss during the interpolation model's training to leverage segmentation labels in existing slices to generate middle ones. Unlike common interpolation techniques in CT volumes, our model highlights the regions of interest (liver and lesions) inside the abdominal CT scans in the interpolated slice. Moreover, our model's outputs are consistent with the original input slices while increasing the segmentation performance in two cutting-edge 3D segmentation pipelines. We tested the proposed model on the CRLM dataset to upsample subjects with thick slices and create isotropic volume for our segmentation model. The produced isotropic dataset increases the Dice score in the segmentation of lesions and outperforms other interpolation approaches in terms of interpolation metrics.
6.Stage-by-stage Wavelet Optimization Refinement Diffusion Model for Sparse-View CT Reconstruction
Authors:Kai Xu, Shiyu Lu, Bin Huang, Weiwen Wu, Qiegen Liu
Abstract: Diffusion models have emerged as potential tools to tackle the challenge of sparse-view CT reconstruction, displaying superior performance compared to conventional methods. Nevertheless, these prevailing diffusion models predominantly focus on the sinogram or image domains, which can lead to instability during model training, potentially culminating in convergence towards local minimal solutions. The wavelet trans-form serves to disentangle image contents and features into distinct frequency-component bands at varying scales, adeptly capturing diverse directional structures. Employing the Wavelet transform as a guiding sparsity prior significantly enhances the robustness of diffusion models. In this study, we present an innovative approach named the Stage-by-stage Wavelet Optimization Refinement Diffusion (SWORD) model for sparse-view CT reconstruction. Specifically, we establish a unified mathematical model integrating low-frequency and high-frequency generative models, achieving the solution with optimization procedure. Furthermore, we perform the low-frequency and high-frequency generative models on wavelet's decomposed components rather than sinogram or image domains, ensuring the stability of model training. Our method rooted in established optimization theory, comprising three distinct stages, including low-frequency generation, high-frequency refinement and domain transform. Our experimental results demonstrate that the proposed method outperforms existing state-of-the-art methods both quantitatively and qualitatively.
7.Modality Cycles with Masked Conditional Diffusion for Unsupervised Anomaly Segmentation in MRI
Authors:Ziyun Liang, Harry Anthony, Felix Wagner, Konstantinos Kamnitsas
Abstract: Unsupervised anomaly segmentation aims to detect patterns that are distinct from any patterns processed during training, commonly called abnormal or out-of-distribution patterns, without providing any associated manual segmentations. Since anomalies during deployment can lead to model failure, detecting the anomaly can enhance the reliability of models, which is valuable in high-risk domains like medical imaging. This paper introduces Masked Modality Cycles with Conditional Diffusion (MMCCD), a method that enables segmentation of anomalies across diverse patterns in multimodal MRI. The method is based on two fundamental ideas. First, we propose the use of cyclic modality translation as a mechanism for enabling abnormality detection. Image-translation models learn tissue-specific modality mappings, which are characteristic of tissue physiology. Thus, these learned mappings fail to translate tissues or image patterns that have never been encountered during training, and the error enables their segmentation. Furthermore, we combine image translation with a masked conditional diffusion model, which attempts to `imagine' what tissue exists under a masked area, further exposing unknown patterns as the generative model fails to recreate them. We evaluate our method on a proxy task by training on healthy-looking slices of BraTS2021 multi-modality MRIs and testing on slices with tumors. We show that our method compares favorably to previous unsupervised approaches based on image reconstruction and denoising with autoencoders and diffusion models.