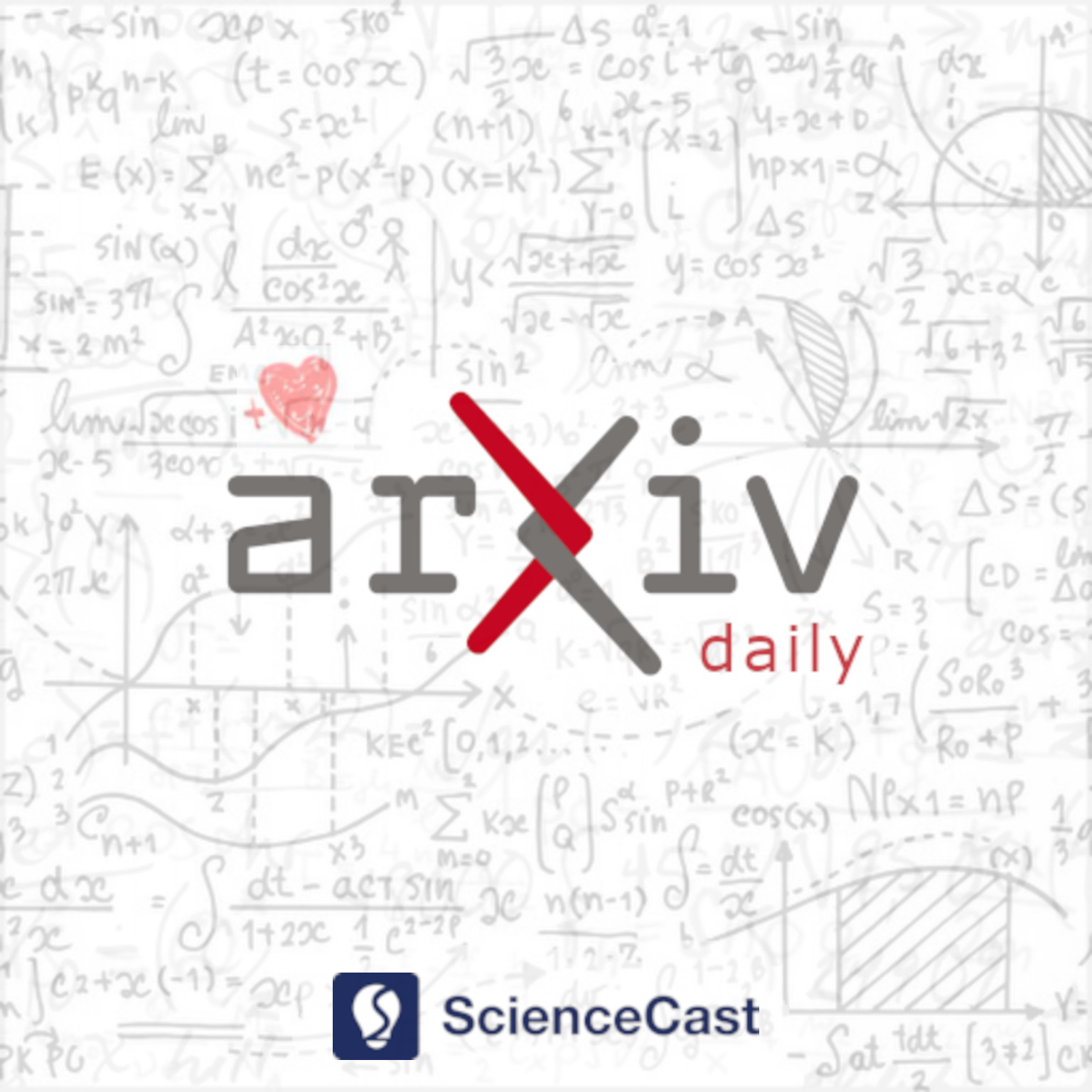
Image and Video Processing (eess.IV)
Thu, 29 Jun 2023
1.SimPLe: Similarity-Aware Propagation Learning for Weakly-Supervised Breast Cancer Segmentation in DCE-MRI
Authors:Yuming Zhong, Yi Wang
Abstract: Breast dynamic contrast-enhanced magnetic resonance imaging (DCE-MRI) plays an important role in the screening and prognosis assessment of high-risk breast cancer. The segmentation of cancerous regions is essential useful for the subsequent analysis of breast MRI. To alleviate the annotation effort to train the segmentation networks, we propose a weakly-supervised strategy using extreme points as annotations for breast cancer segmentation. Without using any bells and whistles, our strategy focuses on fully exploiting the learning capability of the routine training procedure, i.e., the train - fine-tune - retrain process. The network first utilizes the pseudo-masks generated using the extreme points to train itself, by minimizing a contrastive loss, which encourages the network to learn more representative features for cancerous voxels. Then the trained network fine-tunes itself by using a similarity-aware propagation learning (SimPLe) strategy, which leverages feature similarity between unlabeled and positive voxels to propagate labels. Finally the network retrains itself by employing the pseudo-masks generated using previous fine-tuned network. The proposed method is evaluated on our collected DCE-MRI dataset containing 206 patients with biopsy-proven breast cancers. Experimental results demonstrate our method effectively fine-tunes the network by using the SimPLe strategy, and achieves a mean Dice value of 81%.
2.Processing Energy Modeling for Neural Network Based Image Compression
Authors:Christian Herglotz, Fabian Brand, Andy Regensky, Felix Rievel, André Kaup
Abstract: Nowadays, the compression performance of neural-networkbased image compression algorithms outperforms state-of-the-art compression approaches such as JPEG or HEIC-based image compression. Unfortunately, most neural-network based compression methods are executed on GPUs and consume a high amount of energy during execution. Therefore, this paper performs an in-depth analysis on the energy consumption of state-of-the-art neural-network based compression methods on a GPU and show that the energy consumption of compression networks can be estimated using the image size with mean estimation errors of less than 7%. Finally, using a correlation analysis, we find that the number of operations per pixel is the main driving force for energy consumption and deduce that the network layers up to the second downsampling step are consuming most energy.
3.PCDAL: A Perturbation Consistency-Driven Active Learning Approach for Medical Image Segmentation and Classification
Authors:Tao Wang, Xinlin Zhang, Yuanbo Zhou, Junlin Lan, Tao Tan, Min Du, Qinquan Gao, Tong Tong
Abstract: In recent years, deep learning has become a breakthrough technique in assisting medical image diagnosis. Supervised learning using convolutional neural networks (CNN) provides state-of-the-art performance and has served as a benchmark for various medical image segmentation and classification. However, supervised learning deeply relies on large-scale annotated data, which is expensive, time-consuming, and even impractical to acquire in medical imaging applications. Active Learning (AL) methods have been widely applied in natural image classification tasks to reduce annotation costs by selecting more valuable examples from the unlabeled data pool. However, their application in medical image segmentation tasks is limited, and there is currently no effective and universal AL-based method specifically designed for 3D medical image segmentation. To address this limitation, we propose an AL-based method that can be simultaneously applied to 2D medical image classification, segmentation, and 3D medical image segmentation tasks. We extensively validated our proposed active learning method on three publicly available and challenging medical image datasets, Kvasir Dataset, COVID-19 Infection Segmentation Dataset, and BraTS2019 Dataset. The experimental results demonstrate that our PCDAL can achieve significantly improved performance with fewer annotations in 2D classification and segmentation and 3D segmentation tasks. The codes of this study are available at https://github.com/ortonwang/PCDAL.
4.MLA-BIN: Model-level Attention and Batch-instance Style Normalization for Domain Generalization of Federated Learning on Medical Image Segmentation
Authors:Fubao Zhu, Yanhui Tian, Chuang Han, Yanting Li, Jiaofen Nan, Ni Yao, Weihua Zhou
Abstract: The privacy protection mechanism of federated learning (FL) offers an effective solution for cross-center medical collaboration and data sharing. In multi-site medical image segmentation, each medical site serves as a client of FL, and its data naturally forms a domain. FL supplies the possibility to improve the performance of seen domains model. However, there is a problem of domain generalization (DG) in the actual de-ployment, that is, the performance of the model trained by FL in unseen domains will decrease. Hence, MLA-BIN is proposed to solve the DG of FL in this study. Specifically, the model-level attention module (MLA) and batch-instance style normalization (BIN) block were designed. The MLA represents the unseen domain as a linear combination of seen domain models. The atten-tion mechanism is introduced for the weighting coefficient to obtain the optimal coefficient ac-cording to the similarity of inter-domain data features. MLA enables the global model to gen-eralize to unseen domain. In the BIN block, batch normalization (BN) and instance normalization (IN) are combined to perform the shallow layers of the segmentation network for style normali-zation, solving the influence of inter-domain image style differences on DG. The extensive experimental results of two medical image seg-mentation tasks demonstrate that the proposed MLA-BIN outperforms state-of-the-art methods.
5.Histopathology Slide Indexing and Search: Are We There Yet?
Authors:Helen H. Shang, Mohammad Sadegh Nasr, Jai Prakash Veerla, Parisa Boodaghi Malidarreh, MD Jillur Rahman Saurav, Amir Hajighasemi, Manfred Huber, Chace Moleta, Jitin Makker, Jacob M. Luber
Abstract: The search and retrieval of digital histopathology slides is an important task that has yet to be solved. In this case study, we investigate the clinical readiness of three state-of-the-art histopathology slide search engines, Yottixel, SISH, and RetCCL, on three patients with solid tumors. We provide a qualitative assessment of each model's performance in providing retrieval results that are reliable and useful to pathologists. We found that all three image search engines fail to produce consistently reliable results and have difficulties in capturing granular and subtle features of malignancy, limiting their diagnostic accuracy. Based on our findings, we also propose a minimal set of requirements to further advance the development of accurate and reliable histopathology image search engines for successful clinical adoption.
6.Twice Binnable Color Filter Arrays
Authors:Mritunjay Singh, Tripurari Singh
Abstract: Pixel binning enables high speed, low power readout in low resolution modes, and more importantly, a reduction of read noise via floating diffusion binning. New, high resolution CMOS image sensors for mobile phones have moved beyond the once-binnable Quad Bayer and RGBW-Kodak patterns to the twice binnable Hexadeca Bayer pattern featuring 4x4 tiles of like colored pixels.Pixel binning enables high speed, low power readout in low resolution modes, and more importantly, a reduction of read noise via floating diffusion binning. New, high resolution CMOS image sensors for mobile phones have moved beyond the once-binnable Quad Bayer and RGBW-Kodak patterns to the twice binnable Hexadeca Bayer pattern featuring 4x4 tiles of like colored pixels. In this paper we present the non-intuitive result that Nona and Hexadeca Bayer can be superior to Quad Bayer in demosaicking quality due to degeneracies in the latter's spectrum. Hexadeca Bayer, nevertheless, suffers from the weakness of generating Quad Bayer after one round of binning. We present a novel twice binnable RGBW CFA, composed of 2x2 tiles capable of 4:1 floating diffusion binning, that is free from spectral degeneracies and thus demosaick well in full resolution and both binned modes. It also has a 4 dB low light SNR advantage over Quad and Hexadeca Bayer in the full resolution mode, and 6 dB SNR advantage in both the binned modes.