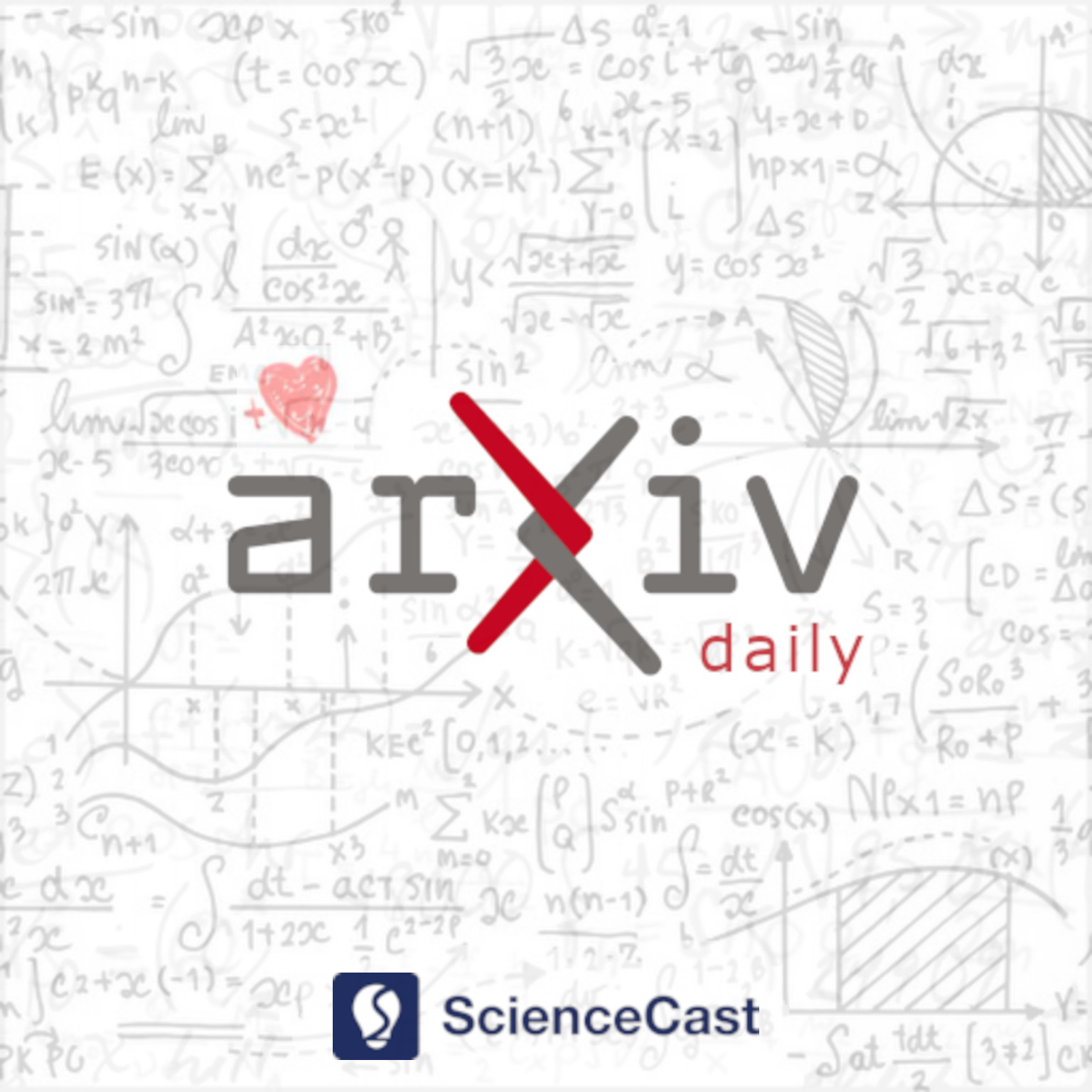
Image and Video Processing (eess.IV)
Wed, 12 Jul 2023
1.Unified Medical Image-Text-Label Contrastive Learning With Continuous Prompt
Authors:Yuhao Wang
Abstract: Contrastive language-image Pre-training (CLIP) [13] can leverage large datasets of unlabeled Image-Text pairs, which have demonstrated impressive performance in various downstream tasks. Given that annotating medical data is time-consuming and laborious, Image-Text Pre-training has promising applications in exploiting large-scale medical image and radiology report datasets. However, medical Image-Text Pre-training faces several challenges, as follows: (1) Due to privacy concerns, the amount of available medical data is relatively small compared to natural data, leading to weaker generalization ability of the model. (2) Medical images are highly similar with only fine-grained differences in subtleties, resulting in a large number of false-negative sample pairs in comparison learning. (3) The hand-crafted Prompt usually differs from the natural medical image report, Subtle changes in wording can lead to significant differences in performance. In this paper, we propose a unified Image-Text-Label contrastive learning framework based on continuous prompts, with three main contributions. First, We unified the data of images, text, and labels, which greatly expanded the training data that the model could utilize. Second, we address the issue of data diversity and the impact of hand-crafted prompts on model performance by introducing continuous implicit prompts. Lastly, we propose a ImageText-Label contrastive Training to mitigate the problem of too many false-negative samples. We demonstrate through sufficient experiments that the Unified Medical Contrastive Learning (UMCL) framework exhibits excellent performance on several downstream tasks.
2.Spatially-Adaptive Learning-Based Image Compression with Hierarchical Multi-Scale Latent Spaces
Authors:Fabian Brand, Alexander Kopte, Kristian Fischer, André Kaup
Abstract: Adaptive block partitioning is responsible for large gains in current image and video compression systems. This method is able to compress large stationary image areas with only a few symbols, while maintaining a high level of quality in more detailed areas. Current state-of-the-art neural-network-based image compression systems however use only one scale to transmit the latent space. In previous publications, we proposed RDONet, a scheme to transmit the latent space in multiple spatial resolutions. Following this principle, we extend a state-of-the-art compression network by a second hierarchical latent-space level to enable multi-scale processing. We extend the existing rate variability capabilities of RDONet by a gain unit. With that we are able to outperform an equivalent traditional autoencoder by 7% rate savings. Furthermore, we show that even though we add an additional latent space, the complexity only increases marginally and the decoding time can potentially even be decreased.
3.Local Conditional Neural Fields for Versatile and Generalizable Large-Scale Reconstructions in Computational Imaging
Authors:Hao Wang, Lei Tian
Abstract: Deep learning has transformed computational imaging, but traditional pixel-based representations limit their ability to capture continuous, multiscale details of objects. Here we introduce a novel Local Conditional Neural Fields (LCNF) framework, leveraging a continuous implicit neural representation to address this limitation. LCNF enables flexible object representation and facilitates the reconstruction of multiscale information. We demonstrate the capabilities of LCNF in solving the highly ill-posed inverse problem in Fourier ptychographic microscopy (FPM) with multiplexed measurements, achieving robust, scalable, and generalizable large-scale phase retrieval. Unlike traditional neural fields frameworks, LCNF incorporates a local conditional representation that promotes model generalization, learning multiscale information, and efficient processing of large-scale imaging data. By combining an encoder and a decoder conditioned on a learned latent vector, LCNF achieves versatile continuous-domain super-resolution image reconstruction. We demonstrate accurate reconstruction of wide field-of-view, high-resolution phase images using only a few multiplexed measurements. LCNF robustly captures the continuous object priors and eliminates various phase artifacts, even when it is trained on imperfect datasets. The framework exhibits strong generalization, reconstructing diverse objects even with limited training data. Furthermore, LCNF can be trained on a physics simulator using natural images and successfully applied to experimental measurements on biological samples. Our results highlight the potential of LCNF for solving large-scale inverse problems in computational imaging, with broad applicability in various deep-learning-based techniques.
4.On the Importance of Denoising when Learning to Compress Images
Authors:Benoit Brummer, Christophe De Vleeschouwer
Abstract: Image noise is ubiquitous in photography. However, image noise is not compressible nor desirable, thus attempting to convey the noise in compressed image bitstreams yields sub-par results in both rate and distortion. We propose to explicitly learn the image denoising task when training a codec. Therefore, we leverage the Natural Image Noise Dataset, which offers a wide variety of scenes captured with various ISO numbers, leading to different noise levels, including insignificant ones. Given this training set, we supervise the codec with noisy-clean image pairs, and show that a single model trained based on a mixture of images with variable noise levels appears to yield best-in-class results with both noisy and clean images, achieving better rate-distortion than a compression-only model or even than a pair of denoising-then-compression models with almost one order of magnitude fewer GMac operations.