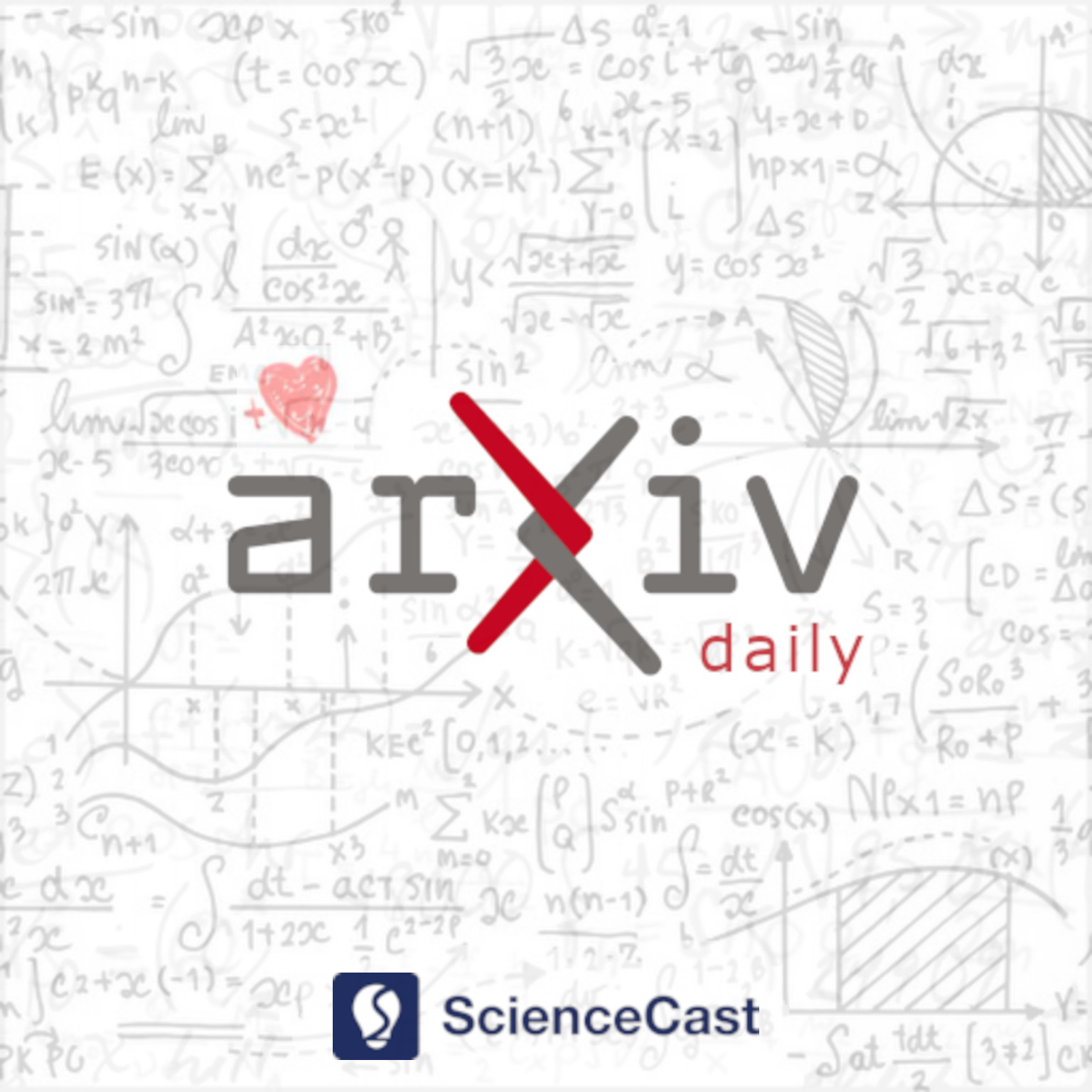
Image and Video Processing (eess.IV)
Wed, 28 Jun 2023
1.A Cascaded Approach for ultraly High Performance Lesion Detection and False Positive Removal in Liver CT Scans
Authors:Fakai Wang, Chi-Tung Cheng, Chien-Wei Peng, Ke Yan, Min Wu, Le Lu, Chien-Hung Liao, Ling Zhang
Abstract: Liver cancer has high morbidity and mortality rates in the world. Multi-phase CT is a main medical imaging modality for detecting/identifying and diagnosing liver tumors. Automatically detecting and classifying liver lesions in CT images have the potential to improve the clinical workflow. This task remains challenging due to liver lesions' large variations in size, appearance, image contrast, and the complexities of tumor types or subtypes. In this work, we customize a multi-object labeling tool for multi-phase CT images, which is used to curate a large-scale dataset containing 1,631 patients with four-phase CT images, multi-organ masks, and multi-lesion (six major types of liver lesions confirmed by pathology) masks. We develop a two-stage liver lesion detection pipeline, where the high-sensitivity detecting algorithms in the first stage discover as many lesion proposals as possible, and the lesion-reclassification algorithms in the second stage remove as many false alarms as possible. The multi-sensitivity lesion detection algorithm maximizes the information utilization of the individual probability maps of segmentation, and the lesion-shuffle augmentation effectively explores the texture contrast between lesions and the liver. Independently tested on 331 patient cases, the proposed model achieves high sensitivity and specificity for malignancy classification in the multi-phase contrast-enhanced CT (99.2%, 97.1%, diagnosis setting) and in the noncontrast CT (97.3%, 95.7%, screening setting).
2.Chan-Vese Attention U-Net: An attention mechanism for robust segmentation
Authors:Nicolas Makaroff, Laurent D. Cohen
Abstract: When studying the results of a segmentation algorithm using convolutional neural networks, one wonders about the reliability and consistency of the results. This leads to questioning the possibility of using such an algorithm in applications where there is little room for doubt. We propose in this paper a new attention gate based on the use of Chan-Vese energy minimization to control more precisely the segmentation masks given by a standard CNN architecture such as the U-Net model. This mechanism allows to obtain a constraint on the segmentation based on the resolution of a PDE. The study of the results allows us to observe the spatial information retained by the neural network on the region of interest and obtains competitive results on the binary segmentation. We illustrate the efficiency of this approach for medical image segmentation on a database of MRI brain images.
3.1M parameters are enough? A lightweight CNN-based model for medical image segmentation
Authors:Binh-Duong Dinh, Thanh-Thu Nguyen, Thi-Thao Tran, Van-Truong Pham
Abstract: Convolutional neural networks (CNNs) and Transformer-based models are being widely applied in medical image segmentation thanks to their ability to extract high-level features and capture important aspects of the image. However, there is often a trade-off between the need for high accuracy and the desire for low computational cost. A model with higher parameters can theoretically achieve better performance but also result in more computational complexity and higher memory usage, and thus is not practical to implement. In this paper, we look for a lightweight U-Net-based model which can remain the same or even achieve better performance, namely U-Lite. We design U-Lite based on the principle of Depthwise Separable Convolution so that the model can both leverage the strength of CNNs and reduce a remarkable number of computing parameters. Specifically, we propose Axial Depthwise Convolutions with kernels 7x7 in both the encoder and decoder to enlarge the model receptive field. To further improve the performance, we use several Axial Dilated Depthwise Convolutions with filters 3x3 for the bottleneck as one of our branches. Overall, U-Lite contains only 878K parameters, 35 times less than the traditional U-Net, and much more times less than other modern Transformer-based models. The proposed model cuts down a large amount of computational complexity while attaining an impressive performance on medical segmentation tasks compared to other state-of-the-art architectures. The code will be available at: https://github.com/duong-db/U-Lite.
4.Generalizing Surgical Instruments Segmentation to Unseen Domains with One-to-Many Synthesis
Authors:An Wang, Mobarakol Islam, Mengya Xu, Hongliang Ren
Abstract: Despite their impressive performance in various surgical scene understanding tasks, deep learning-based methods are frequently hindered from deploying to real-world surgical applications for various causes. Particularly, data collection, annotation, and domain shift in-between sites and patients are the most common obstacles. In this work, we mitigate data-related issues by efficiently leveraging minimal source images to generate synthetic surgical instrument segmentation datasets and achieve outstanding generalization performance on unseen real domains. Specifically, in our framework, only one background tissue image and at most three images of each foreground instrument are taken as the seed images. These source images are extensively transformed and employed to build up the foreground and background image pools, from which randomly sampled tissue and instrument images are composed with multiple blending techniques to generate new surgical scene images. Besides, we introduce hybrid training-time augmentations to diversify the training data further. Extensive evaluation on three real-world datasets, i.e., Endo2017, Endo2018, and RoboTool, demonstrates that our one-to-many synthetic surgical instruments datasets generation and segmentation framework can achieve encouraging performance compared with training with real data. Notably, on the RoboTool dataset, where a more significant domain gap exists, our framework shows its superiority of generalization by a considerable margin. We expect that our inspiring results will attract research attention to improving model generalization with data synthesizing.
5.DoseDiff: Distance-aware Diffusion Model for Dose Prediction in Radiotherapy
Authors:Yiwen Zhang, Chuanpu Li, Liming Zhong, Zeli Chen, Wei Yang, Xuetao Wang
Abstract: Treatment planning is a critical component of the radiotherapy workflow, typically carried out by a medical physicist using a time-consuming trial-and-error manner. Previous studies have proposed knowledge-based or deep learning-based methods for predicting dose distribution maps to assist medical physicists in improving the efficiency of treatment planning. However, these dose prediction methods usuallylack the effective utilization of distance information between surrounding tissues andtargets or organs-at-risk (OARs). Moreover, they are poor in maintaining the distribution characteristics of ray paths in the predicted dose distribution maps, resulting in a loss of valuable information obtained by medical physicists. In this paper, we propose a distance-aware diffusion model (DoseDiff) for precise prediction of dose distribution. We define dose prediction as a sequence of denoising steps, wherein the predicted dose distribution map is generated with the conditions of the CT image and signed distance maps (SDMs). The SDMs are obtained by a distance transformation from the masks of targets or OARs, which provide the distance information from each pixel in the image to the outline of the targets or OARs. Besides, we propose a multiencoder and multi-scale fusion network (MMFNet) that incorporates a multi-scale fusion and a transformer-based fusion module to enhance information fusion between the CT image and SDMs at the feature level. Our model was evaluated on two datasets collected from patients with breast cancer and nasopharyngeal cancer, respectively. The results demonstrate that our DoseDiff outperforms the state-of-the-art dose prediction methods in terms of both quantitative and visual quality.