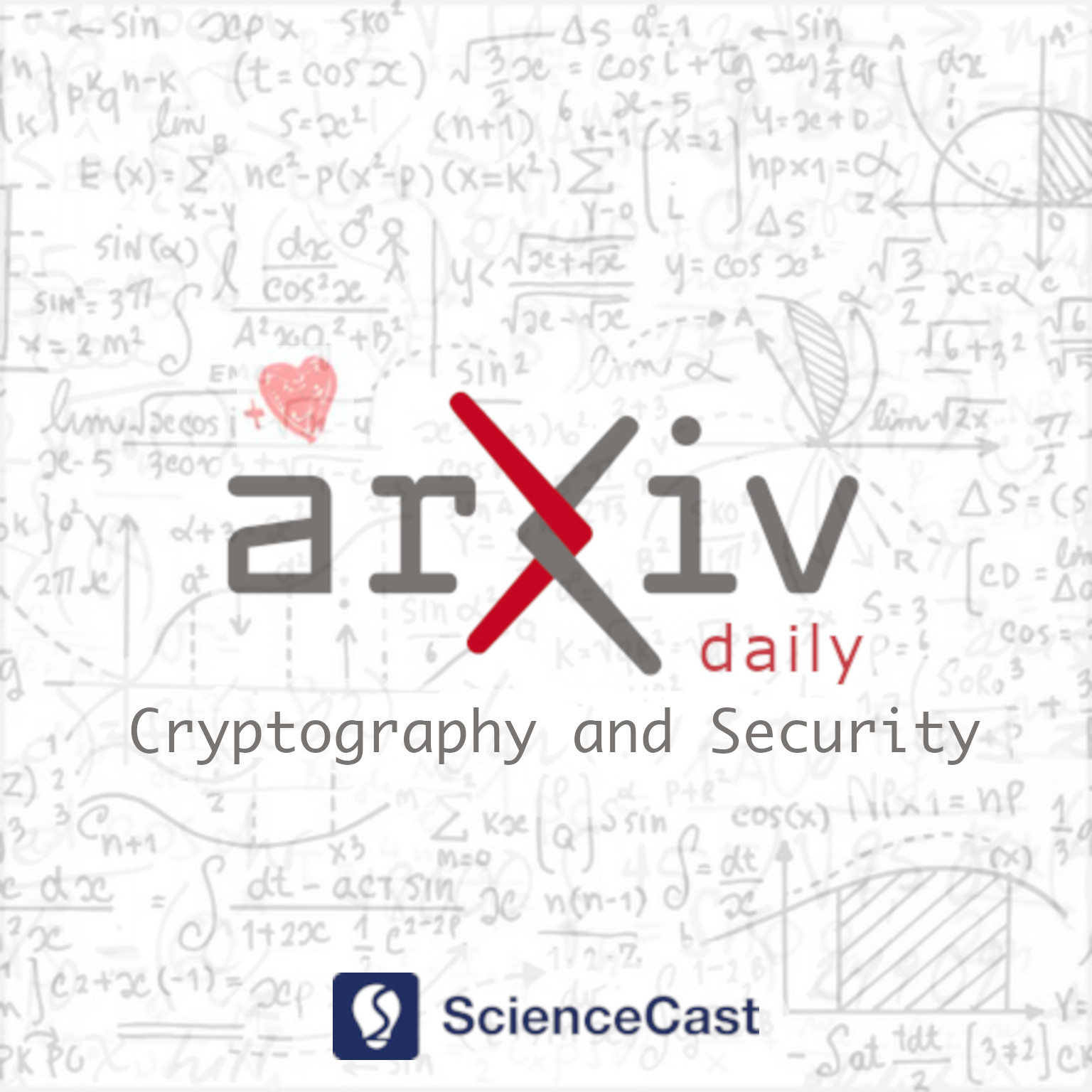
Cryptography and Security (cs.CR)
Tue, 12 Sep 2023
1.CToMP: A Cycle-task-oriented Memory Protection Scheme for Unmanned Systems
Authors:Chengyan Ma, Ning Xi, Di Lu, Yebo Feng, Jianfeng Ma
Abstract: Memory corruption attacks (MCAs) refer to malicious behaviors of system intruders that modify the contents of a memory location to disrupt the normal operation of computing systems, causing leakage of sensitive data or perturbations to ongoing processes. Unlike general-purpose systems, unmanned systems cannot deploy complete security protection schemes, due to their limitations in size, cost and performance. MCAs in unmanned systems are particularly difficult to defend against. Furthermore, MCAs have diverse and unpredictable attack interfaces in unmanned systems, severely impacting digital and physical sectors. In this paper, we first generalize, model and taxonomize MCAs found in unmanned systems currently, laying the foundation for designing a portable and general defense approach. According to different attack mechanisms, we found that MCAs are mainly categorized into two types--return2libc and return2shellcode. To tackle return2libc attacks, we model the erratic operation of unmanned systems with cycles and then propose a cycle-task-oriented memory protection (CToMP) approach to protect control flows from tampering. To defend against return2shellcode attacks, we introduce a secure process stack with a randomized memory address by leveraging the memory pool to prevent Shellcode from being executed. Moreover, we discuss the mechanism by which CToMP resists the ROP attack, a novel variant of return2libc attacks. Finally, we implement CToMP on CUAV V5+ with Ardupilot and Crazyflie. The evaluation and security analysis results demonstrate that the proposed approach CToMP is resilient to various MCAs in unmanned systems with low footprints and system overhead.
2.Backdoor Attacks and Countermeasures in Natural Language Processing Models: A Comprehensive Security Review
Authors:Pengzhou Cheng, Zongru Wu, Wei Du, Gongshen Liu
Abstract: Deep Neural Networks (DNNs) have led to unprecedented progress in various natural language processing (NLP) tasks. Owing to limited data and computation resources, using third-party data and models has become a new paradigm for adapting various tasks. However, research shows that it has some potential security vulnerabilities because attackers can manipulate the training process and data source. Such a way can set specific triggers, making the model exhibit expected behaviors that have little inferior influence on the model's performance for primitive tasks, called backdoor attacks. Hence, it could have dire consequences, especially considering that the backdoor attack surfaces are broad. To get a precise grasp and understanding of this problem, a systematic and comprehensive review is required to confront various security challenges from different phases and attack purposes. Additionally, there is a dearth of analysis and comparison of the various emerging backdoor countermeasures in this situation.In this paper, we conduct a timely review of backdoor attacks and countermeasures to sound the red alarm for the NLP security community. According to the affected stage of the machine learning pipeline, the attack surfaces are recognized to be wide and then formalized into three categorizations: attacking pre-trained model with fine-tuning (APMF) or prompt-tuning (APMP), and attacking final model with training (AFMT), where AFMT can be subdivided into different attack aims. Thus, attacks under each categorization are combed. The countermeasures are categorized into two general classes: sample inspection and model inspection. Overall, the research on the defense side is far behind the attack side, and there is no single defense that can prevent all types of backdoor attacks. An attacker can intelligently bypass existing defenses with a more invisible attack. ......
3.Verifiable Fairness: Privacy-preserving Computation of Fairness for Machine Learning Systems
Authors:Ehsan Toreini, Maryam Mehrnezhad, Aad van Moorsel
Abstract: Fair machine learning is a thriving and vibrant research topic. In this paper, we propose Fairness as a Service (FaaS), a secure, verifiable and privacy-preserving protocol to computes and verify the fairness of any machine learning (ML) model. In the deisgn of FaaS, the data and outcomes are represented through cryptograms to ensure privacy. Also, zero knowledge proofs guarantee the well-formedness of the cryptograms and underlying data. FaaS is model--agnostic and can support various fairness metrics; hence, it can be used as a service to audit the fairness of any ML model. Our solution requires no trusted third party or private channels for the computation of the fairness metric. The security guarantees and commitments are implemented in a way that every step is securely transparent and verifiable from the start to the end of the process. The cryptograms of all input data are publicly available for everyone, e.g., auditors, social activists and experts, to verify the correctness of the process. We implemented FaaS to investigate performance and demonstrate the successful use of FaaS for a publicly available data set with thousands of entries.
4.HoneyEVSE: An Honeypot to emulate Electric Vehicle Supply Equipments
Authors:Massimiliano Baldo, Tommaso Bianchi, Mauro Conti, Alessio Trevisan, Federico Turrin
Abstract: To fight climate change, new "green" technology are emerging, most of them using electricity as a power source. Among the solutions, Electric Vehicles (EVs) represent a central asset in the future transport system. EVs require a complex infrastructure to enable the so-called Vehicle-to-Grid (V2G) paradigm to manage the charging process between the smart grid and the EV. In this paradigm, the Electric Vehicle Supply Equipment (EVSE), or charging station, is the end device that authenticates the vehicle and delivers the power to charge it. However, since an EVSE is publicly exposed and connected to the Internet, recent works show how an attacker with physical tampering and remote access can target an EVSE, exposing the security of the entire infrastructure and the final user. For this reason, it is important to develop novel strategies to secure such infrastructures. In this paper we present HoneyEVSE, the first honeypot conceived to simulate an EVSE. HoneyEVSE can simulate with high fidelity the EV charging process and, at the same time, enables a user to interact with it through a dashboard. Furthermore, based on other charging columns exposed on the Internet, we emulate the login and device information pages to increase user engagement. We exposed HoneyEVSE for 30 days to the Internet to assess its capability and measured the interaction received with its Shodan Honeyscore. Results show that HoneyEVSE can successfully evade the Shodan honeyscore metric while attracting a high number of interactions on the exposed services.
5.Unveiling Signle-Bit-Flip Attacks on DNN Executables
Authors:Yanzuo Chen The Hong Kong University of Science and Technology, Zhibo Liu The Hong Kong University of Science and Technology, Yuanyuan Yuan The Hong Kong University of Science and Technology, Sihang Hu Huawei Technologies, Tianxiang Li Huawei Technologies, Shuai Wang The Hong Kong University of Science and Technology
Abstract: Recent research has shown that bit-flip attacks (BFAs) can manipulate deep neural networks (DNNs) via DRAM Rowhammer exploitations. Existing attacks are primarily launched over high-level DNN frameworks like PyTorch and flip bits in model weight files. Nevertheless, DNNs are frequently compiled into low-level executables by deep learning (DL) compilers to fully leverage low-level hardware primitives. The compiled code is usually high-speed and manifests dramatically distinct execution paradigms from high-level DNN frameworks. In this paper, we launch the first systematic study on the attack surface of BFA specifically for DNN executables compiled by DL compilers. We design an automated search tool to identify vulnerable bits in DNN executables and identify practical attack vectors that exploit the model structure in DNN executables with BFAs (whereas prior works make likely strong assumptions to attack model weights). DNN executables appear more "opaque" than models in high-level DNN frameworks. Nevertheless, we find that DNN executables contain extensive, severe (e.g., single-bit flip), and transferrable attack surfaces that are not present in high-level DNN models and can be exploited to deplete full model intelligence and control output labels. Our finding calls for incorporating security mechanisms in future DNN compilation toolchains.
6.Systematic Evaluation of Geolocation Privacy Mechanisms
Authors:Alban Héon, Ryan Sheatsley, Quinn Burke, Blaine Hoak, Eric Pauley, Yohan Beugin, Patrick McDaniel
Abstract: Location data privacy has become a serious concern for users as Location Based Services (LBSs) have become an important part of their life. It is possible for malicious parties having access to geolocation data to learn sensitive information about the user such as religion or political views. Location Privacy Preserving Mechanisms (LPPMs) have been proposed by previous works to ensure the privacy of the shared data while allowing the users to use LBSs. But there is no clear view of which mechanism to use according to the scenario in which the user makes use of a LBS. The scenario is the way the user is using a LBS (frequency of reports, number of reports). In this paper, we study the sensitivity of LPPMs on the scenario on which they are used. We propose a framework to systematically evaluate LPPMs by considering an exhaustive combination of LPPMs, attacks and metrics. Using our framework we compare a selection of LPPMs including an improved mechanism that we introduce. By evaluating over a variety of scenarios, we find that the efficacy (privacy, utility, and robustness) of the studied mechanisms is dependent on the scenario: for example the privacy of Planar Laplace geo-indistinguishability is greatly reduced in a continuous scenario. We show that the scenario is essential to consider when choosing an obfuscation mechanism for a given application.