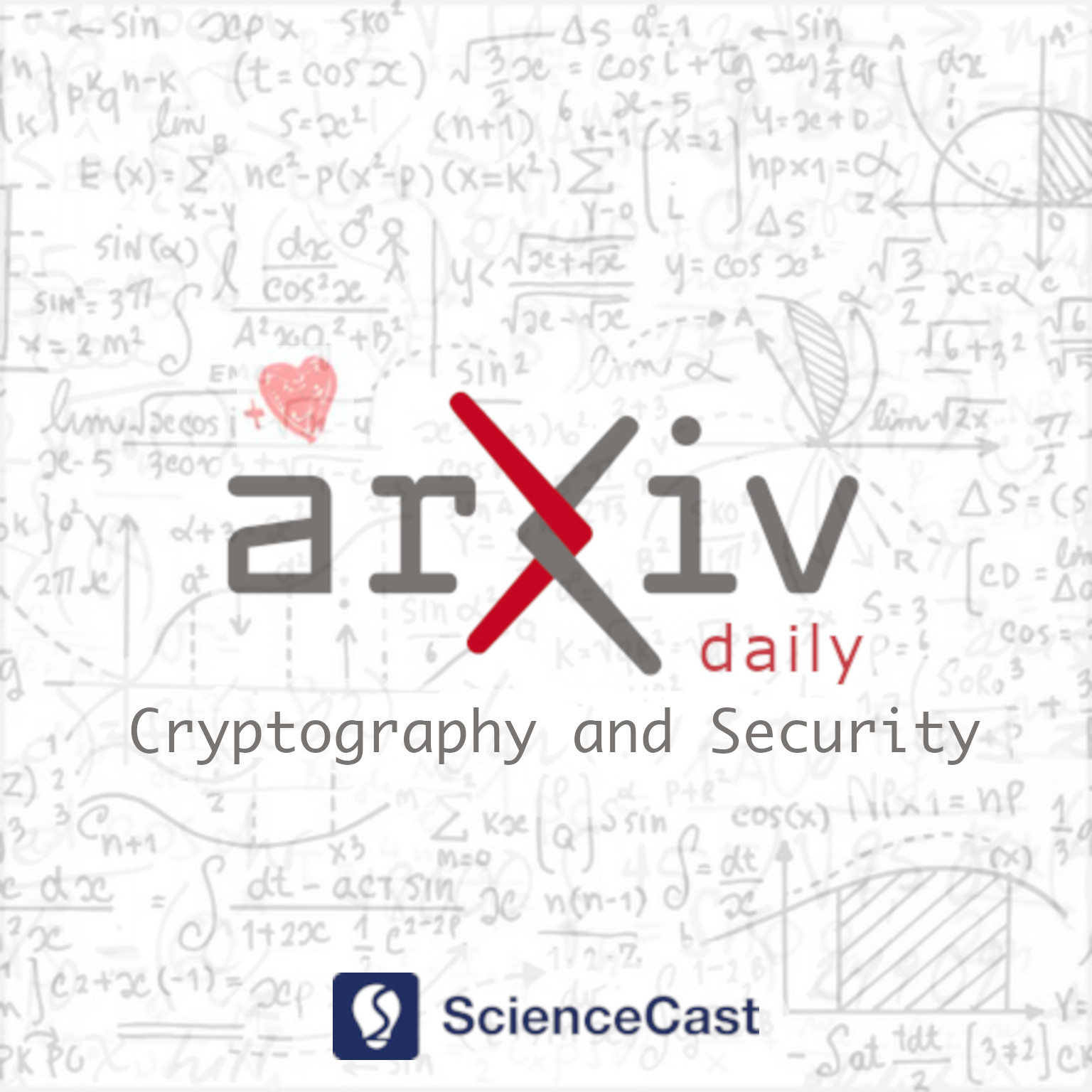
Cryptography and Security (cs.CR)
Tue, 04 Jul 2023
1.Machine Learning-Based Intrusion Detection: Feature Selection versus Feature Extraction
Authors:Vu-Duc Ngo, Tuan-Cuong Vuong, Thien Van Luong, Hung Tran
Abstract: Internet of things (IoT) has been playing an important role in many sectors, such as smart cities, smart agriculture, smart healthcare, and smart manufacturing. However, IoT devices are highly vulnerable to cyber-attacks, which may result in security breaches and data leakages. To effectively prevent these attacks, a variety of machine learning-based network intrusion detection methods for IoT networks have been developed, which often rely on either feature extraction or feature selection techniques for reducing the dimension of input data before being fed into machine learning models. This aims to make the detection complexity low enough for real-time operations, which is particularly vital in any intrusion detection systems. This paper provides a comprehensive comparison between these two feature reduction methods of intrusion detection in terms of various performance metrics, namely, precision rate, recall rate, detection accuracy, as well as runtime complexity, in the presence of the modern UNSW-NB15 dataset as well as both binary and multiclass classification. For example, in general, the feature selection method not only provides better detection performance but also lower training and inference time compared to its feature extraction counterpart, especially when the number of reduced features K increases. However, the feature extraction method is much more reliable than its selection counterpart, particularly when K is very small, such as K = 4. Additionally, feature extraction is less sensitive to changing the number of reduced features K than feature selection, and this holds true for both binary and multiclass classifications. Based on this comparison, we provide a useful guideline for selecting a suitable intrusion detection type for each specific scenario, as detailed in Tab. 14 at the end of Section IV.
2.With Trail to Follow: Measurements of Real-world Non-fungible Token Phishing Attacks on Ethereum
Authors:Jingjing Yang, Jieli Liu, Jiajing Wu
Abstract: With the popularity of Non-Fungible Tokens (NFTs), NFTs have become a new target of phishing attacks, posing a significant threat to the NFT trading ecosystem. There has been growing anecdotal evidence that new means of NFT phishing attacks have emerged in Ethereum ecosystem. Most of the existing research focus on detecting phishing scam accounts for native cryptocurrency on the blockchain, but there is a lack of research in the area of phishing attacks of emerging NFTs. Although a few studies have recently started to focus on the analysis and detection of NFT phishing attacks, NFT phishing attack means are diverse and little has been done to understand these various types of NFT phishing attacks. To the best of our knowledge, we are the first to conduct case retrospective analysis and measurement study of real-world historical NFT phishing attacks on Ethereum. By manually analyzing the existing scams reported by Chainabuse, we classify NFT phishing attacks into four patterns. For each pattern, we further investigate the tricks and working principles of them. Based on 469 NFT phishing accounts collected up until October 2022 from multiple channels, we perform a measurement study of on-chain transaction data crawled from Etherscan to characterizing NFT phishing scams by analyzing the modus operandi and preferences of NFT phishing scammers, as well as economic impacts and whereabouts of stolen NFTs. We classify NFT phishing transactions into one of the four patterns by log parsing and transaction record parsing. We find these phishing accounts stole 19,514 NFTs for a total profit of 8,858.431 ETH (around 18.57 million dollars). We also observe that scammers remain highly active in the last two years and favor certain categories and series of NFTs, accompanied with signs of gang theft.
3.Overconfidence is a Dangerous Thing: Mitigating Membership Inference Attacks by Enforcing Less Confident Prediction
Authors:Zitao Chen, Karthik Pattabiraman
Abstract: Machine learning (ML) models are vulnerable to membership inference attacks (MIAs), which determine whether a given input is used for training the target model. While there have been many efforts to mitigate MIAs, they often suffer from limited privacy protection, large accuracy drop, and/or requiring additional data that may be difficult to acquire. This work proposes a defense technique, HAMP that can achieve both strong membership privacy and high accuracy, without requiring extra data. To mitigate MIAs in different forms, we observe that they can be unified as they all exploit the ML model's overconfidence in predicting training samples through different proxies. This motivates our design to enforce less confident prediction by the model, hence forcing the model to behave similarly on the training and testing samples. HAMP consists of a novel training framework with high-entropy soft labels and an entropy-based regularizer to constrain the model's prediction while still achieving high accuracy. To further reduce privacy risk, HAMP uniformly modifies all the prediction outputs to become low-confidence outputs while preserving the accuracy, which effectively obscures the differences between the prediction on members and non-members. We conduct extensive evaluation on five benchmark datasets, and show that HAMP provides consistently high accuracy and strong membership privacy. Our comparison with seven state-of-the-art defenses shows that HAMP achieves a superior privacy-utility trade off than those techniques.
4.SeePrivacy: Automated Contextual Privacy Policy Generation for Mobile Applications
Authors:Shidong Pan, Zhen Tao, Thong Hoang, Dawen Zhang, Zhenchang Xing, Xiwei Xu, Mark Staples, David Lo
Abstract: Privacy policies have become the most critical approach to safeguarding individuals' privacy and digital security. To enhance their presentation and readability, researchers propose the concept of contextual privacy policies (CPPs), aiming to fragment policies into shorter snippets and display them only in corresponding contexts. In this paper, we propose a novel multi-modal framework, namely SeePrivacy, designed to automatically generate contextual privacy policies for mobile apps. Our method synergistically combines mobile GUI understanding and privacy policy document analysis, yielding an impressive overall 83.6% coverage rate for privacy-related context detection and an accuracy of 0.92 in extracting corresponding policy segments. Remarkably, 96% of the retrieved policy segments can be correctly matched with their contexts. The user study shows SeePrivacy demonstrates excellent functionality and usability (4.5/5). Specifically, participants exhibit a greater willingness to read CPPs (4.1/5) compared to original privacy policies (2/5). Our solution effectively assists users in comprehending privacy notices, and this research establishes a solid foundation for further advancements and exploration.
5.Synthetic is all you need: removing the auxiliary data assumption for membership inference attacks against synthetic data
Authors:Florent Guépin, Matthieu Meeus, Ana-Maria Cretu, Yves-Alexandre de Montjoye
Abstract: Synthetic data is emerging as the most promising solution to share individual-level data while safeguarding privacy. Membership inference attacks (MIAs), based on shadow modeling, have become the standard to evaluate the privacy of synthetic data. These attacks, however, currently assume the attacker to have access to an auxiliary dataset sampled from a similar distribution as the training dataset. This often is a very strong assumption that would make an attack unlikely to happen in practice. We here show how this assumption can be removed and how MIAs can be performed using only the synthetic data. More specifically, in three different attack scenarios using only synthetic data, our results demonstrate that MIAs are still successful, across two real-world datasets and two synthetic data generators. These results show how the strong hypothesis made when auditing synthetic data releases - access to an auxiliary dataset - can be relaxed to perform an actual attack.
6.The Path to Fault- and Intrusion-Resilient Manycore Systems on a Chip
Authors:Ali Shoker, Paulo Esteves Verissimo, Marcus Völp
Abstract: The hardware computing landscape is changing. What used to be distributed systems can now be found on a chip with highly configurable, diverse, specialized and general purpose units. Such Systems-on-a-Chip (SoC) are used to control today's cyber-physical systems, being the building blocks of critical infrastructures. They are deployed in harsh environments and are connected to the cyberspace, which makes them exposed to both accidental faults and targeted cyberattacks. This is in addition to the changing fault landscape that continued technology scaling, emerging devices and novel application scenarios will bring. In this paper, we discuss how the very features, distributed, parallelized, reconfigurable, heterogeneous, that cause many of the imminent and emerging security and resilience challenges, also open avenues for their cure though SoC replication, diversity, rejuvenation, adaptation, and hybridization. We show how to leverage these techniques at different levels across the entire SoC hardware/software stack, calling for more research on the topic.
7.Digital Sovereignty Strategies for Every Nation
Authors:Ali Shoker
Abstract: Digital Sovereignty must be on the agenda of every modern nation. Digital technology is becoming part of our life details, from the vital essentials, like food and water management, to transcendence in the Metaverse and Space. Protecting these digital assets will, therefore, be inevitable for a modern country to live, excel and lead. Digital Sovereignty is a strategic necessity to protect these digital assets from the monopoly of friendly rational states, and the threats of unfriendly Malicious states and behaviors. In this work, we revisit the definition and scope of digital sovereignty through extending it to cover the entire value chain of using, owning, and producing digital assets. We emphasize the importance of protecting the operational resources, both raw materials and human expertise, in addition to research and innovation necessary to achieve sustainable sovereignty. We also show that digital sovereignty by autonomy is often impossible, and by mutual cooperation is not always sustainable. To this end, we propose implementing digital sovereignty using Nash Equilibrium, often studied in Game Theory, to govern the relation with Rational states. Finally, we propose a digital sovereignty agenda for different country's digital profiles, based on their status quo, priorities, and capabilities. We survey state-of-the-art digital technology that is useful to make the current digital assets sovereign. Additionally, we propose a roadmap that aims to develop a sovereign digital nation, as close as possible to autonomy. Finally, we draw attention to the need of more research to better understand and implement digital sovereignty from different perspectives: technological, economic, and geopolitical.
8.ProPILE: Probing Privacy Leakage in Large Language Models
Authors:Siwon Kim, Sangdoo Yun, Hwaran Lee, Martin Gubri, Sungroh Yoon, Seong Joon Oh
Abstract: The rapid advancement and widespread use of large language models (LLMs) have raised significant concerns regarding the potential leakage of personally identifiable information (PII). These models are often trained on vast quantities of web-collected data, which may inadvertently include sensitive personal data. This paper presents ProPILE, a novel probing tool designed to empower data subjects, or the owners of the PII, with awareness of potential PII leakage in LLM-based services. ProPILE lets data subjects formulate prompts based on their own PII to evaluate the level of privacy intrusion in LLMs. We demonstrate its application on the OPT-1.3B model trained on the publicly available Pile dataset. We show how hypothetical data subjects may assess the likelihood of their PII being included in the Pile dataset being revealed. ProPILE can also be leveraged by LLM service providers to effectively evaluate their own levels of PII leakage with more powerful prompts specifically tuned for their in-house models. This tool represents a pioneering step towards empowering the data subjects for their awareness and control over their own data on the web.
9.An Algorithm for Persistent Homology Computation Using Homomorphic Encryption
Authors:Dominic Gold, Koray Karabina, Francis C. Motta
Abstract: Topological Data Analysis (TDA) offers a suite of computational tools that provide quantified shape features in high dimensional data that can be used by modern statistical and predictive machine learning (ML) models. In particular, persistent homology (PH) takes in data (e.g., point clouds, images, time series) and derives compact representations of latent topological structures, known as persistence diagrams (PDs). Because PDs enjoy inherent noise tolerance, are interpretable and provide a solid basis for data analysis, and can be made compatible with the expansive set of well-established ML model architectures, PH has been widely adopted for model development including on sensitive data, such as genomic, cancer, sensor network, and financial data. Thus, TDA should be incorporated into secure end-to-end data analysis pipelines. In this paper, we take the first step to address this challenge and develop a version of the fundamental algorithm to compute PH on encrypted data using homomorphic encryption (HE).