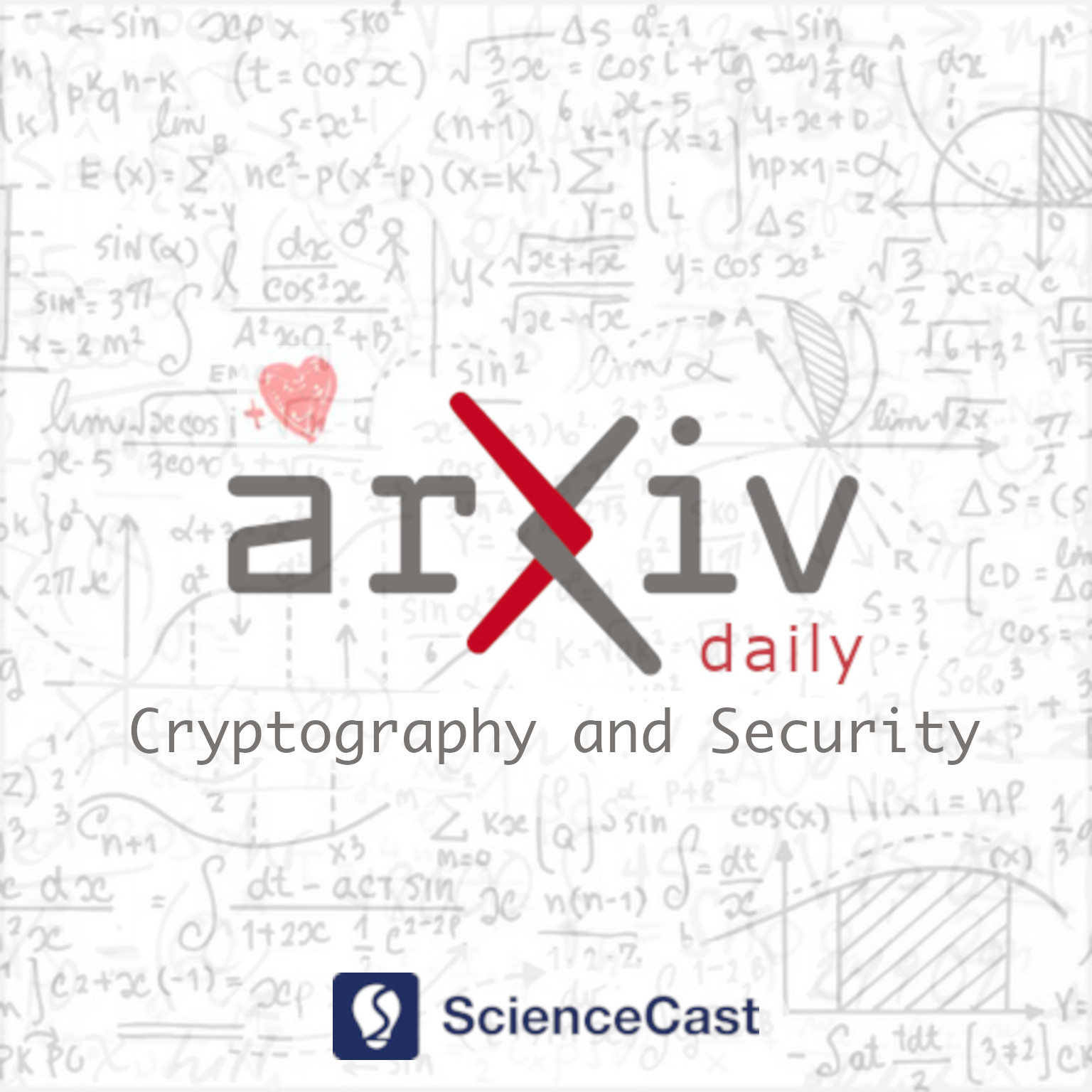
Cryptography and Security (cs.CR)
Tue, 11 Jul 2023
1.A Blockchain-based two Factor Honeytoken Authentication System
Authors:Vasilis Papaspirou, Leandros Maglaras, Ioanna Kantzavelou, Naghemeh Moradpoor, Sokratis Katsikas
Abstract: This paper extends and advances our recently introduced two-factor Honeytoken authentication method by incorporating blockchain technology. This novel approach strengthens the authentication method to prevent many attacks including tampering attacks. Evaluation results show that integrating blockchain into the Honeytoken method could improve performance and operational efficiency.
2.ATWM: Defense against adversarial malware based on adversarial training
Authors:Kun Li, Fan Zhang, Wei Guo
Abstract: Deep learning technology has made great achievements in the field of image. In order to defend against malware attacks, researchers have proposed many Windows malware detection models based on deep learning. However, deep learning models are vulnerable to adversarial example attacks. Malware can generate adversarial malware with the same malicious function to attack the malware detection model and evade detection of the model. Currently, many adversarial defense studies have been proposed, but existing adversarial defense studies are based on image sample and cannot be directly applied to malware sample. Therefore, this paper proposes an adversarial malware defense method based on adversarial training. This method uses preprocessing to defend simple adversarial examples to reduce the difficulty of adversarial training. Moreover, this method improves the adversarial defense capability of the model through adversarial training. We experimented with three attack methods in two sets of datasets, and the results show that the method in this paper can improve the adversarial defense capability of the model without reducing the accuracy of the model.
3.SecFlow: Adaptive Security-Aware Workflow Management System in Multi-Cloud Environments
Authors:Nafiseh Soveizi, Fatih Turkmen
Abstract: In this paper, we propose an architecture for a security-aware workflow management system (WfMS) we call SecFlow in answer to the recent developments of combining workflow management systems with Cloud environments and the still lacking abilities of such systems to ensure the security and privacy of cloud-based workflows. The SecFlow architecture focuses on full workflow life cycle coverage as, in addition to the existing approaches to design security-aware processes, there is a need to fill in the gap of maintaining security properties of workflows during their execution phase. To address this gap, we derive the requirements for such a security-aware WfMS and design a system architecture that meets these requirements. SecFlow integrates key functional components such as secure model construction, security-aware service selection, security violation detection, and adaptive response mechanisms while considering all potential malicious parties in multi-tenant and cloud-based WfMS.
4.Application-aware Energy Attack Mitigation in the Battery-less Internet of Things
Authors:Chetna Singhal, Thiemo Voigt, Luca Mottola
Abstract: We study how to mitigate the effects of energy attacks in the batteryless Internet of Things (IoT). Battery-less IoT devices live and die with ambient energy, as they use energy harvesting to power their operation. They are employed in a multitude of applications, including safety-critical ones such as biomedical implants. Due to scarce energy intakes and limited energy buffers, their executions become intermittent, alternating periods of active operation with periods of recharging their energy buffers. Experimental evidence exists that shows how controlling ambient energy allows an attacker to steer a device execution in unintended ways: energy provisioning effectively becomes an attack vector. We design, implement, and evaluate a mitigation system for energy attacks. By taking into account the specific application requirements and the output of an attack detection module, we tune task execution rates and optimize energy management. This ensures continued application execution in the event of an energy attack. When a device is under attack, our solution ensures the execution of 23.3% additional application cycles compared to the baselines we consider and increases task schedulability by at least 21%, while enabling a 34% higher peripheral availability.
5.Smart Environment for Adaptive Learning of Cybersecurity Skills
Authors:Jan Vykopal, Pavel Seda, Valdemar Švábenský, Pavel Čeleda
Abstract: Hands-on computing education requires a realistic learning environment that enables students to gain and deepen their skills. Available learning environments, including virtual and physical labs, provide students with real-world computer systems but rarely adapt the learning environment to individual students of various proficiency and background. We designed a unique and novel smart environment for adaptive training of cybersecurity skills. The environment collects a variety of student data to assign a suitable learning path through the training. To enable such adaptiveness, we proposed, developed, and deployed a new tutor model and a training format. We evaluated the learning environment using two different adaptive trainings attended by 114 students of various proficiency. The results show students were assigned tasks with a more appropriate difficulty, which enabled them to successfully complete the training. Students reported that they enjoyed the training, felt the training difficulty was appropriately designed, and would attend more training sessions like these. Instructors can use the environment for teaching any topic involving real-world computer networks and systems because it is not tailored to particular training. We freely released the software along with exemplary training so that other instructors can adopt the innovations in their teaching practice.
6.Differential Analysis of Triggers and Benign Features for Black-Box DNN Backdoor Detection
Authors:Hao Fu, Prashanth Krishnamurthy, Siddharth Garg, Farshad Khorrami
Abstract: This paper proposes a data-efficient detection method for deep neural networks against backdoor attacks under a black-box scenario. The proposed approach is motivated by the intuition that features corresponding to triggers have a higher influence in determining the backdoored network output than any other benign features. To quantitatively measure the effects of triggers and benign features on determining the backdoored network output, we introduce five metrics. To calculate the five-metric values for a given input, we first generate several synthetic samples by injecting the input's partial contents into clean validation samples. Then, the five metrics are computed by using the output labels of the corresponding synthetic samples. One contribution of this work is the use of a tiny clean validation dataset. Having the computed five metrics, five novelty detectors are trained from the validation dataset. A meta novelty detector fuses the output of the five trained novelty detectors to generate a meta confidence score. During online testing, our method determines if online samples are poisoned or not via assessing their meta confidence scores output by the meta novelty detector. We show the efficacy of our methodology through a broad range of backdoor attacks, including ablation studies and comparison to existing approaches. Our methodology is promising since the proposed five metrics quantify the inherent differences between clean and poisoned samples. Additionally, our detection method can be incrementally improved by appending more metrics that may be proposed to address future advanced attacks.
7.Let's shake on it: Extracting secure shared keys from Wi-Fi CSI
Authors:Tomer Avrahami, Ofer Amrani, Avishai Wool
Abstract: A shared secret key is necessary for encrypted communications. Since Wi-Fi relies on OFDM, we suggest a method to generate such a key by utilizing Wi-Fi's channel state information (CSI). CSI is typically reciprocal but very sensitive to location: While the legitimate Alice and Bob observe the same CSI, an eavesdropper Eve observes an uncorrelated CSI when positioned over 0.5 wavelength away. We show that if endpoint Bob is shaken, sufficient diversity is induced in the CSI so that it can serve as a source of true randomness. Then we show that the CSI among neighboring sub-carriers is correlated, so we select a small set of judiciously-spaced sub-carriers, and use a majority rule around each. We demonstrate that Alice and Bob observe a 5-15\% bit mismatch rate (BMR) in the extracted bitstream while Eve observes a BMR of around 50\% even when placed within 10cm of Alice. We employ the cryptography-oriented definition of min-entropy to estimate the number of secure bits within the bitstream, and use the Cascade algorithm of quantum-key-distribution to reconcile Alice and Bob's bitstreams, while quantifying the number of bits leaked by the algorithm. Accounting for both the min-entropy and the cascade leakage we quantify the Secured Bit Generation Rate of our method. We conducted extensive tests in an indoor environment. Our system exhibits a secure bit generation rate of 1.2--1.6 %secure bits per packet, at distances ranging from 0.5m--9m, and can generate a secure shared 128-bit key with 20sec of device shaking.
8.Improving the Security of Smartwatch Payment with Deep Learning
Authors:George Webber
Abstract: Making contactless payments using a smartwatch is increasingly popular, but this payment medium lacks traditional biometric security measures such as facial or fingerprint recognition. In 2022, Sturgess et al. proposed WatchAuth, a system for authenticating smartwatch payments using the physical gesture of reaching towards a payment terminal. While effective, the system requires the user to undergo a burdensome enrolment period to achieve acceptable error levels. In this dissertation, we explore whether applications of deep learning can reduce the number of gestures a user must provide to enrol into an authentication system for smartwatch payment. We firstly construct a deep-learned authentication system that outperforms the current state-of-the-art, including in a scenario where the target user has provided a limited number of gestures. We then develop a regularised autoencoder model for generating synthetic user-specific gestures. We show that using these gestures in training improves classification ability for an authentication system. Through this technique we can reduce the number of gestures required to enrol a user into a WatchAuth-like system without negatively impacting its error rates.