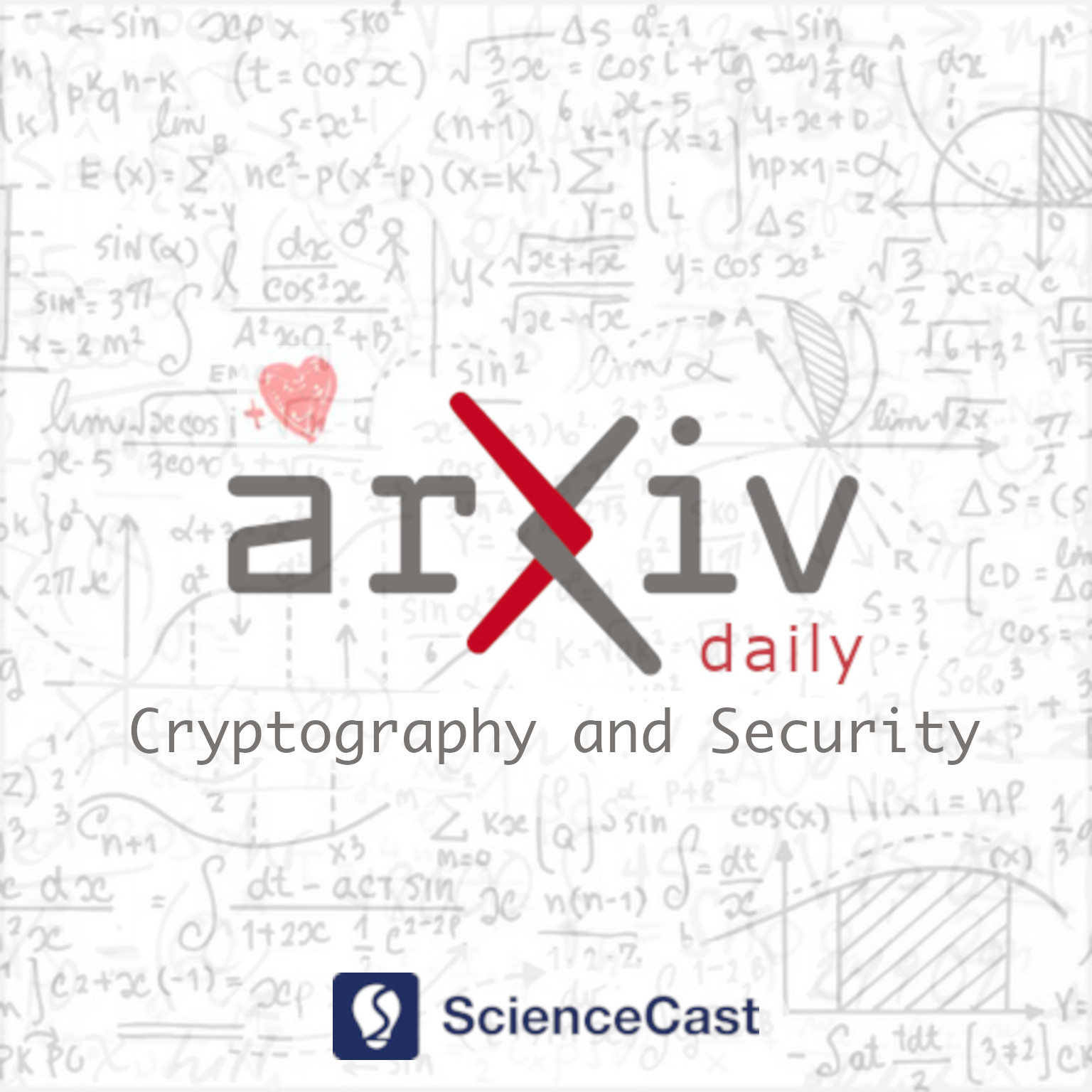
Cryptography and Security (cs.CR)
Tue, 27 Jun 2023
1.Errorless Robust JPEG Steganography Using Steganographic Polar Codes
Authors:Jimin Zhang, Xianfeng Zhao, Xiaolei He
Abstract: Recently, a robust steganographic algorithm that achieves errorless robustness against JPEG recompression is proposed. The method evaluates the behavior of DCT coefficients after recompression using the local JPEG encoder to select robust coefficients and sets the other coefficients as wet cost. Combining the lattice embedding scheme, the method is errorless by construction. However, the authors only concern with the success rate under theoretical embedding, while the success rate of the implementation with practical steganographic codes is not verified. In this letter, we implement the method with two steganographic codes, i.e., steganographic polar code and syndrome-trellis code. By analyzing the possibility of success embedding of two steganographic codes under wet paper embedding, we discover that steganographic polar code achieves success embedding with a larger number of wet coefficients compared with syndrome-trellis code, which makes steganographic polar code more suitable under the errorless robust embedding paradigm. The experimental results show that the combination of steganographic polar code and errorless robust embedding achieves a higher success rate compared with the implementation with syndrome-trellis code under close security performance.
2.Catch Me If You Can: A New Low-Rate DDoS Attack Strategy Disguised by Feint
Authors:Tianyang Cai, Yuqi Li, Tao Jia, Leo Yu Zhang, Zheng Yang
Abstract: While collaborative systems provide convenience to our lives, they also face many security threats. One of them is the Low-rate Distributed Denial-of-Service (LDDoS) attack, which is a worthy concern. Unlike volumetric DDoS attacks that continuously send large volumes of traffic, LDDoS attacks are more stealthy and difficult to be detected owing to their low-volume feature. Due to its stealthiness and harmfulness, LDDoS has become one of the most destructive attacks in cloud computing. Although a few LDDoS attack detection and defense methods have been proposed, we observe that sophisticated LDDoS attacks (being more stealthy) can bypass some of the existing LDDoS defense methods. To verify our security observation, we proposed a new Feint-based LDDoS (F-LDDoS) attack strategy. In this strategy, we divide a Pulse Interval into a Feinting Interval and an Attack Interval. Unlike the previous LDDoS attacks, the bots also send traffic randomly in the Feinting Interval, thus disguise themselves as benign users during the F-LDDoS attack. In this way, although the victim detects that it is under an LDDoS attack, it is difficult to locate the attack sources and apply mitigation solutions. Experimental results show that F-LDDoS attack can degrade TCP bandwidth 6.7%-14% more than the baseline LDDoS attack. Besides, F-LDDoS also reduces the similarities between bot traffic and aggregated attack traffic, and increases the uncertainty of packet arrival. These results mean that the proposed F-LDDoS is more effective and more stealthy than normal LDDoS attacks. Finally, we discuss the countermeasures of F-LDDoS to draw the attention of defenders and improve the defense methods.
3.A Highly Accurate Query-Recovery Attack against Searchable Encryption using Non-Indexed Documents
Authors:Marc Damie, Florian Hahn, Andreas Peter
Abstract: Cloud data storage solutions offer customers cost-effective and reduced data management. While attractive, data security issues remain to be a core concern. Traditional encryption protects stored documents, but hinders simple functionalities such as keyword search. Therefore, searchable encryption schemes have been proposed to allow for the search on encrypted data. Efficient schemes leak at least the access pattern (the accessed documents per keyword search), which is known to be exploitable in query recovery attacks assuming the attacker has a significant amount of background knowledge on the stored documents. Existing attacks can only achieve decent results with strong adversary models (e.g. at least 20% of previously known documents or require additional knowledge such as on query frequencies) and they give no metric to evaluate the certainty of recovered queries. This hampers their practical utility and questions their relevance in the real-world. We propose a refined score attack which achieves query recovery rates of around 85% without requiring exact background knowledge on stored documents; a distributionally similar, but otherwise different (i.e., non-indexed), dataset suffices. The attack starts with very few known queries (around 10 known queries in our experiments over different datasets of varying size) and then iteratively recovers further queries with confidence scores by adding previously recovered queries that had high confidence scores to the set of known queries. Additional to high recovery rates, our approach yields interpretable results in terms of confidence scores.
4.Your Attack Is Too DUMB: Formalizing Attacker Scenarios for Adversarial Transferability
Authors:Marco Alecci, Mauro Conti, Francesco Marchiori, Luca Martinelli, Luca Pajola
Abstract: Evasion attacks are a threat to machine learning models, where adversaries attempt to affect classifiers by injecting malicious samples. An alarming side-effect of evasion attacks is their ability to transfer among different models: this property is called transferability. Therefore, an attacker can produce adversarial samples on a custom model (surrogate) to conduct the attack on a victim's organization later. Although literature widely discusses how adversaries can transfer their attacks, their experimental settings are limited and far from reality. For instance, many experiments consider both attacker and defender sharing the same dataset, balance level (i.e., how the ground truth is distributed), and model architecture. In this work, we propose the DUMB attacker model. This framework allows analyzing if evasion attacks fail to transfer when the training conditions of surrogate and victim models differ. DUMB considers the following conditions: Dataset soUrces, Model architecture, and the Balance of the ground truth. We then propose a novel testbed to evaluate many state-of-the-art evasion attacks with DUMB; the testbed consists of three computer vision tasks with two distinct datasets each, four types of balance levels, and three model architectures. Our analysis, which generated 13K tests over 14 distinct attacks, led to numerous novel findings in the scope of transferable attacks with surrogate models. In particular, mismatches between attackers and victims in terms of dataset source, balance levels, and model architecture lead to non-negligible loss of attack performance.
5.A New Mathematical Optimization-Based Method for the m-invariance Problem
Authors:Adrian Tobar, Jordi Castro, Claudio Gentile
Abstract: The issue of ensuring privacy for users who share their personal information has been a growing priority in a business and scientific environment where the use of different types of data and the laws that protect it have increased in tandem. Different technologies have been widely developed for static publications, i.e., where the information is published only once, such as k-anonymity and {\epsilon}-differential privacy. In the case where microdata information is published dynamically, although established notions such as m-invariance and {\tau}-safety already exist, developments for improving utility remain superficial. We propose a new heuristic approach for the NP-hard combinatorial problem of m-invariance and {\tau}-safety, which is based on a mathematical optimization column generation scheme. The quality of a solution to m-invariance and {\tau}-safety can be measured by the Information Loss (IL), a value in [0,100], the closer to 0 the better. We show that our approach improves by far current heuristics, providing in some instances solutions with ILs of 1.87, 8.5 and 1.93, while the state-of-the art methods reported ILs of 39.03, 51.84 and 57.97, respectively.
6.Identifying Practical Challenges in the Implementation of Technical Measures for Data Privacy Compliance
Authors:Oleksandra Klymenko, Stephen Meisenbacher, Florian Matthes
Abstract: Modern privacy regulations provide a strict mandate for data processing entities to implement appropriate technical measures to demonstrate compliance. In practice, determining what measures are indeed "appropriate" is not trivial, particularly in light of vague guidelines provided by privacy regulations. To exacerbate the issue, challenges arise not only in the implementation of the technical measures themselves, but also in a variety of factors involving the roles, processes, decisions, and culture surrounding the pursuit of privacy compliance. In this paper, we present 33 challenges faced in the implementation of technical measures for privacy compliance, derived from a qualitative analysis of 16 interviews with privacy professionals. In addition, we evaluate the interview findings in a survey study, which gives way to a discussion of the identified challenges and their implications.
7.PASNet: Polynomial Architecture Search Framework for Two-party Computation-based Secure Neural Network Deployment
Authors:Hongwu Peng, Shanglin Zhou, Yukui Luo, Nuo Xu, Shijin Duan, Ran Ran, Jiahui Zhao, Chenghong Wang, Tong Geng, Wujie Wen, Xiaolin Xu, Caiwen Ding
Abstract: Two-party computation (2PC) is promising to enable privacy-preserving deep learning (DL). However, the 2PC-based privacy-preserving DL implementation comes with high comparison protocol overhead from the non-linear operators. This work presents PASNet, a novel systematic framework that enables low latency, high energy efficiency & accuracy, and security-guaranteed 2PC-DL by integrating the hardware latency of the cryptographic building block into the neural architecture search loss function. We develop a cryptographic hardware scheduler and the corresponding performance model for Field Programmable Gate Arrays (FPGA) as a case study. The experimental results demonstrate that our light-weighted model PASNet-A and heavily-weighted model PASNet-B achieve 63 ms and 228 ms latency on private inference on ImageNet, which are 147 and 40 times faster than the SOTA CryptGPU system, and achieve 70.54% & 78.79% accuracy and more than 1000 times higher energy efficiency.
8.RansomAI: AI-powered Ransomware for Stealthy Encryption
Authors:Jan von der Assen, Alberto Huertas Celdrán, Janik Luechinger, Pedro Miguel Sánchez Sánchez, Gérôme Bovet, Gregorio Martínez Pérez, Burkhard Stiller
Abstract: Cybersecurity solutions have shown promising performance when detecting ransomware samples that use fixed algorithms and encryption rates. However, due to the current explosion of Artificial Intelligence (AI), sooner than later, ransomware (and malware in general) will incorporate AI techniques to intelligently and dynamically adapt its encryption behavior to be undetected. It might result in ineffective and obsolete cybersecurity solutions, but the literature lacks AI-powered ransomware to verify it. Thus, this work proposes RansomAI, a Reinforcement Learning-based framework that can be integrated into existing ransomware samples to adapt their encryption behavior and stay stealthy while encrypting files. RansomAI presents an agent that learns the best encryption algorithm, rate, and duration that minimizes its detection (using a reward mechanism and a fingerprinting intelligent detection system) while maximizing its damage function. The proposed framework was validated in a ransomware, Ransomware-PoC, that infected a Raspberry Pi 4, acting as a crowdsensor. A pool of experiments with Deep Q-Learning and Isolation Forest (deployed on the agent and detection system, respectively) has demonstrated that RansomAI evades the detection of Ransomware-PoC affecting the Raspberry Pi 4 in a few minutes with >90% accuracy.
9.MTFS: a Moving Target Defense-Enabled File System for Malware Mitigation
Authors:Jan von der Assen, Alberto Huertas Celdrán, Rinor Sefa, Gérôme Bovet, Burkhard Stiller
Abstract: Ransomware has remained one of the most notorious threats in the cybersecurity field. Moving Target Defense (MTD) has been proposed as a novel paradigm for proactive defense. Although various approaches leverage MTD, few of them rely on the operating system and, specifically, the file system, thereby making them dependent on other computing devices. Furthermore, existing ransomware defense techniques merely replicate or detect attacks, without preventing them. Thus, this paper introduces the MTFS overlay file system and the design and implementation of three novel MTD techniques implemented on top of it. One delaying attackers, one trapping recursive directory traversal, and another one hiding file types. The effectiveness of the techniques are shown in two experiments. First, it is shown that the techniques can delay and mitigate ransomware on real IoT devices. Secondly, in a broader scope, the solution was confronted with 14 ransomware samples, highlighting that it can save 97% of the files.
10.Developing and Deploying Security Applications for In-Vehicle Networks
Authors:Samuel C Hollifield, Pablo Moriano, William L Lambert, Joel Asiamah, Isaac Sikkema, Michael D Iannacone
Abstract: Radiological material transportation is primarily facilitated by heavy-duty on-road vehicles. Modern vehicles have dozens of electronic control units or ECUs, which are small, embedded computers that communicate with sensors and each other for vehicle functionality. ECUs use a standardized network architecture--Controller Area Network or CAN--which presents grave security concerns that have been exploited by researchers and hackers alike. For instance, ECUs can be impersonated by adversaries who have infiltrated an automotive CAN and disable or invoke unintended vehicle functions such as brakes, acceleration, or safety mechanisms. Further, the quality of security approaches varies wildly between manufacturers. Thus, research and development of after-market security solutions have grown remarkably in recent years. Many researchers are exploring deployable intrusion detection and prevention mechanisms using machine learning and data science techniques. However, there is a gap between developing security system algorithms and deploying prototype security appliances in-vehicle. In this paper, we, a research team at Oak Ridge National Laboratory working in this space, highlight challenges in the development pipeline, and provide techniques to standardize methodology and overcome technological hurdles.
11.Automated Fuzzing Harness Generation for Library APIs and Binary Protocol Parsers
Authors:Chaitanya Rahalkar
Abstract: Fuzzing is a widely used software security testing technique that is designed to identify vulnerabilities in systems by providing invalid or unexpected input. Continuous fuzzing systems like OSS-FUZZ have been successful in finding security bugs in many different software systems. The typical process of finding security bugs using fuzzing involves several steps: first, the "fuzz-worthy" functions that are likely to contain vulnerabilities must be identified; second, the setup requirements for the API must be understood before it can be called; third, a fuzzing harness must be written and bound to a coverage-guided fuzzer like LLVM's LibFuzzer; and finally, the security bugs discovered by the fuzzing harness must be triaged and checked for reproducibility. This project focuses on automating the first two steps in this process. In particular, we present an automated system that can generate fuzzing harnesses for library APIs and binary protocol parsers by analyzing unit tests. This allows for the scaling of the fuzzing infrastructure in proportion to the growth of the codebase, without the need for manual coding of harnesses. Additionally, we develop a metric to assess the "fuzz-worthiness" of an API, enabling us to prioritize the most promising targets for testing.