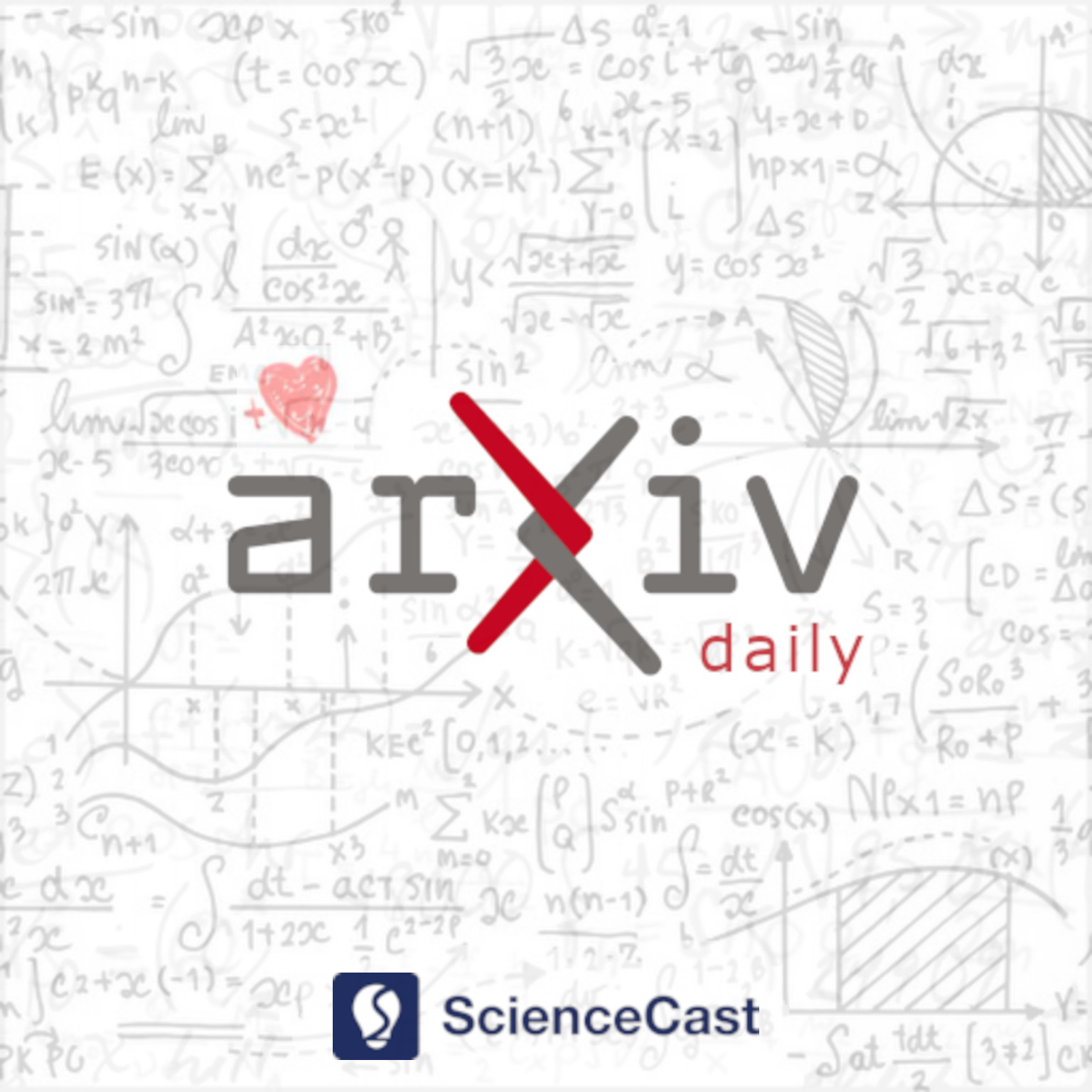
Optics (physics.optics)
Mon, 17 Jul 2023
1.Narrow-linewidth 852-nm DBR-LD with self-injection lock based on high-fineness optical cavity filtering
Authors:Lili Hao, Rui Chang, Xiaokai Hou, Jun He, Junmin Wang
Abstract: Narrow-linewidth lasers have high spectral purity, long coherent length and low phase noise, so they have important applications in cold atom physics, quantum communication, quantum information processing and optical precision measurement. We inject transmitted laser from a narrow-linewidth (15 kHz) flat-concave Fabry-Perot (F-P) cavity made of ultra-low expansion (ULE) optical glass into 852-nm distributed-Bragg-reflector type laser diode (DBR-LD), of which the comprehensive linewidth of 1.67 MHz for the free running case. With the increase of self-injection power, the laser linewidth is gradually narrowed, and the inject-locking current range is gradually increased. The narrowest linewidth measured by the delayed frequency-shifted self-heterodyne (DFSSH) method is 263 Hz. Moreover, to characterize the laser phase noise, we use a detuned F-P cavity to measure the conversion signal from laser phase noise to intensity noise for both the free running case and self-injection lock case. Laser phase noise for the self-injection lock case is significantly suppressed in the analysis frequency range of 0.1-10 MHz compared to the free running case. Especially, the phase noise is suppressed by more than 30dB at the analysis frequency of 100 kHz.
2.Towards mid-infrared computational temporal ghost imaging
Authors:Han Wu, Bo Hu, Fei Peng, Zinan Wang, Goëry Genty, Houkun Liang
Abstract: Ghost imaging in the time domain opens new possibilities to reconstruct an unknown temporal object by correlating the multiple probing temporal intensity patterns with the integrated signal measured after transmission through the temporal object. By using the pre-programmed temporal patterns as the probe, computational temporal ghost imaging (TGI) can reconstruct the fast temporal object with only one slow photodetector and significantly reduce the number of measurement realizations. However, direct implementation of computational TGI requires the use of suitable instrumentation such as, e.g., ultrafast modulators to pre-program temporal patterns at the source wavelength, which are not necessarily available in all wavelength regions, such as mid-infrared region. Here, we experimentally demonstrated MIR computational TGI for the first time, to the best of our knowledge, based on the frequency down-conversion scheme. Instead of directly pre-programming temporal patterns at MIR light, in the frequency down-conversion temporal ghost imaging, the pre-programmed temporal patterns are modulated at 1.5 um light and then the pre-programmed 1.5 um light is interacted with another 1 um continuous-wave light to realize difference-frequency generation (DFG) process in periodically poled lithium niobate crystal. In this way, the pre-programmed temporal patterns can be transferred from 1.5 um light to the generated DFG light source at 3.4 um, and computational TGI could be performed to image a fast temporal object in MIR with a MIR slow detector. With the use of near-infrared optical modulator and wavelength-versatile near-infrared fiber lasers, the concept of frequency down-conversion based ghost imaging unlocks new possibilities to realized computational imaging in the spectral region where preprogrammed modulation is difficult to applied, such as the mid-infrared and THz regions.
3.Manipulate Quantum Emission by Interface States between Multi-component Moiré Lattice and Metasurface
Authors:Z. N. Liu, X. Q. Zhao, Y. L. Zhao, S. N. Zhu, H. Liu
Abstract: In recent years, moir\'e lattice has become a hot topic and inspired the research upsurge of moir\'e lattice. In this work, we propose a method of constructing a multi-composite moir\'e lattice, which is composed of over three periodic component structures. Moreover, we propose the moir\'e lattice-metasurface structure, which can realize the multi-wavelength interface states between these kinds of moir\'e lattices and metasurfaces. The wavelength, polarization, and number of moir\'e interface states can be manipulated flexibly, with anisotropic metasurfaces. These multi-wavelength interface states are employed to enhance quantum emission (QE) and over 20 times QE efficiency can be obtained.
4.Nonlinear Processing with Linear Optics
Authors:Mustafa Yildirim, Niyazi Ulas Dinc, Ilker Oguz, Demetri Psaltis, Christophe Moser
Abstract: Deep neural networks have achieved remarkable breakthroughs by leveraging multiple layers of data processing to extract hidden representations, albeit at the cost of large electronic computing power. To enhance energy efficiency and speed, the optical implementation of neural networks aims to harness the advantages of optical bandwidth and the energy efficiency of optical interconnections. In the absence of low-power optical nonlinearities, the challenge in the implementation of multilayer optical networks lies in realizing multiple optical layers without resorting to electronic components. In this study, we present a novel framework that uses multiple scattering that is capable of synthesizing programmable linear and nonlinear transformations concurrently at low optical power by leveraging the nonlinear relationship between the scattering potential, represented by data, and the scattered field. Theoretical and experimental investigations show that repeating the data by multiple scattering enables non-linear optical computing at low power continuous wave light.
5.Deep Learning with Passive Optical Nonlinear Mapping
Authors:Fei Xia, Kyungduk Kim, Yaniv Eliezer, Liam Shaughnessy, Sylvain Gigan, Hui Cao
Abstract: Deep learning has fundamentally transformed artificial intelligence, but the ever-increasing complexity in deep learning models calls for specialized hardware accelerators. Optical accelerators can potentially offer enhanced performance, scalability, and energy efficiency. However, achieving nonlinear mapping, a critical component of neural networks, remains challenging optically. Here, we introduce a design that leverages multiple scattering in a reverberating cavity to passively induce optical nonlinear random mapping, without the need for additional laser power. A key advantage emerging from our work is that we show we can perform optical data compression, facilitated by multiple scattering in the cavity, to efficiently compress and retain vital information while also decreasing data dimensionality. This allows rapid optical information processing and generation of low dimensional mixtures of highly nonlinear features. These are particularly useful for applications demanding high-speed analysis and responses such as in edge computing devices. Utilizing rapid optical information processing capabilities, our optical platforms could potentially offer more efficient and real-time processing solutions for a broad range of applications. We demonstrate the efficacy of our design in improving computational performance across tasks, including classification, image reconstruction, key-point detection, and object detection, all achieved through optical data compression combined with a digital decoder. Notably, we observed high performance, at an extreme compression ratio, for real-time pedestrian detection. Our findings pave the way for novel algorithms and architectural designs for optical computing.
6.A newcomer's guide to deep learning for inverse design in nano-photonics
Authors:Abdourahman Khaireh-Walieh, Denis Langevin, Pauline Bennet, Olivier Teytaud, Antoine Moreau, Peter R. Wiecha
Abstract: Nanophotonic devices manipulate light at sub-wavelength scales, enabling tasks such as light concentration, routing, and filtering. Designing these devices is a challenging task. Traditionally, solving this problem has relied on computationally expensive, iterative methods. In recent years, deep learning techniques have emerged as promising tools for tackling the inverse design of nanophotonic devices. While several review articles have provided an overview of the progress in this rapidly evolving field, there is a need for a comprehensive tutorial that specifically targets newcomers without prior experience in deep learning. Our goal is to address this gap and provide practical guidance for applying deep learning to individual scientific problems. We introduce the fundamental concepts of deep learning and critically discuss the potential benefits it offers for various inverse design problems in nanophotonics. We present a suggested workflow and detailed, practical design guidelines to help newcomers navigate the challenges they may encounter. By following our guide, newcomers can avoid frustrating roadblocks commonly experienced when venturing into deep learning for the first time. In a second part, we explore different iterative and direct deep learning-based techniques for inverse design, and evaluate their respective advantages and limitations. To enhance understanding and facilitate implementation, we supplement the manuscript with detailed Python notebook examples, illustrating each step of the discussed processes. While our tutorial primarily focuses on researchers in (nano-)photonics, it is also relevant for those working with deep learning in other research domains. We aim at providing a solid starting point to empower researchers to leverage the potential of deep learning in their scientific pursuits.