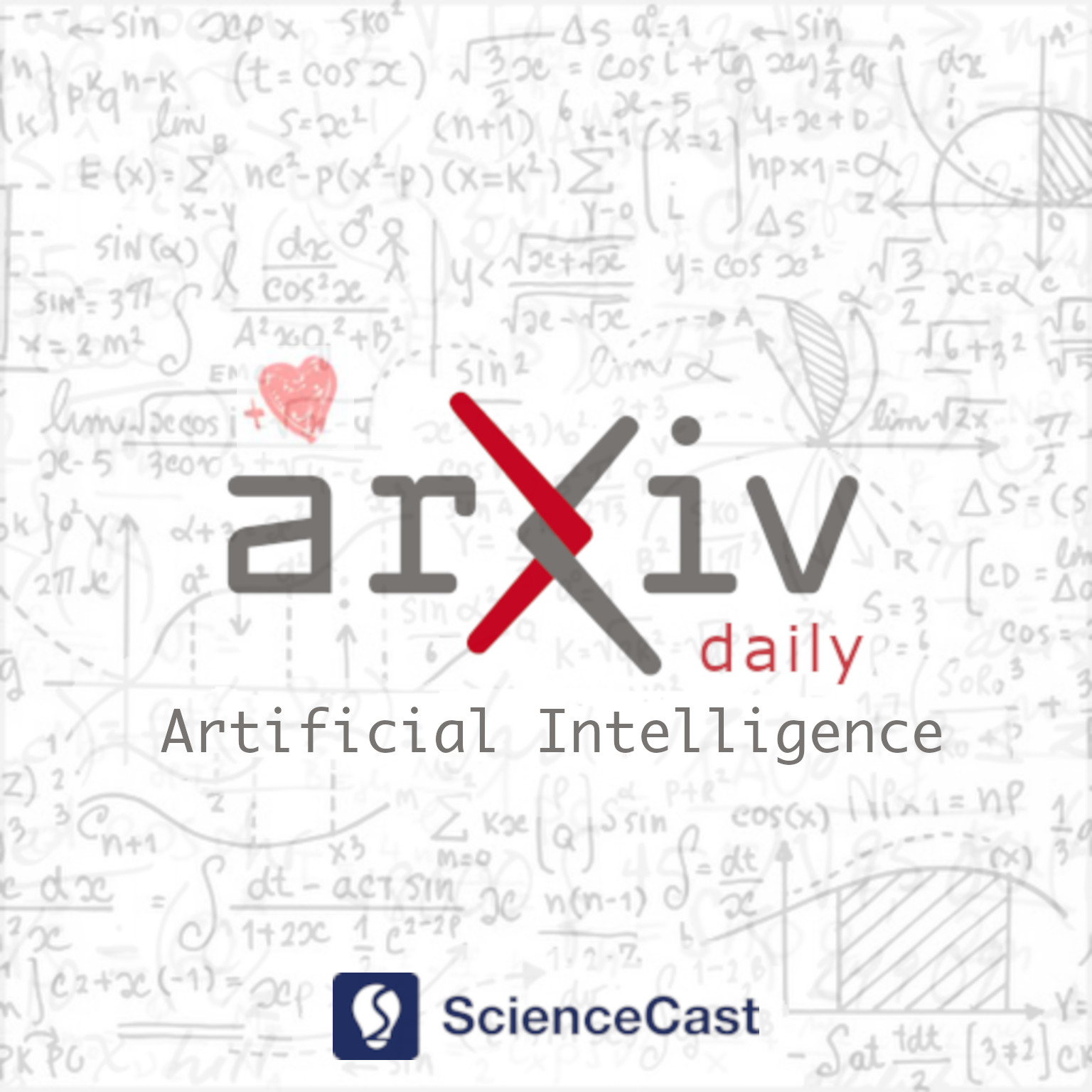
Artificial Intelligence (cs.AI)
Wed, 12 Apr 2023
1.Multi-agent Policy Reciprocity with Theoretical Guarantee
Authors:Haozhi Wang, Yinchuan Li, Qing Wang, Yunfeng Shao, Jianye Hao
Abstract: Modern multi-agent reinforcement learning (RL) algorithms hold great potential for solving a variety of real-world problems. However, they do not fully exploit cross-agent knowledge to reduce sample complexity and improve performance. Although transfer RL supports knowledge sharing, it is hyperparameter sensitive and complex. To solve this problem, we propose a novel multi-agent policy reciprocity (PR) framework, where each agent can fully exploit cross-agent policies even in mismatched states. We then define an adjacency space for mismatched states and design a plug-and-play module for value iteration, which enables agents to infer more precise returns. To improve the scalability of PR, deep PR is proposed for continuous control tasks. Moreover, theoretical analysis shows that agents can asymptotically reach consensus through individual perceived rewards and converge to an optimal value function, which implies the stability and effectiveness of PR, respectively. Experimental results on discrete and continuous environments demonstrate that PR outperforms various existing RL and transfer RL methods.
2.Learning to Communicate and Collaborate in a Competitive Multi-Agent Setup to Clean the Ocean from Macroplastics
Authors:Philipp Dominic Siedler
Abstract: Finding a balance between collaboration and competition is crucial for artificial agents in many real-world applications. We investigate this using a Multi-Agent Reinforcement Learning (MARL) setup on the back of a high-impact problem. The accumulation and yearly growth of plastic in the ocean cause irreparable damage to many aspects of oceanic health and the marina system. To prevent further damage, we need to find ways to reduce macroplastics from known plastic patches in the ocean. Here we propose a Graph Neural Network (GNN) based communication mechanism that increases the agents' observation space. In our custom environment, agents control a plastic collecting vessel. The communication mechanism enables agents to develop a communication protocol using a binary signal. While the goal of the agent collective is to clean up as much as possible, agents are rewarded for the individual amount of macroplastics collected. Hence agents have to learn to communicate effectively while maintaining high individual performance. We compare our proposed communication mechanism with a multi-agent baseline without the ability to communicate. Results show communication enables collaboration and increases collective performance significantly. This means agents have learned the importance of communication and found a balance between collaboration and competition.