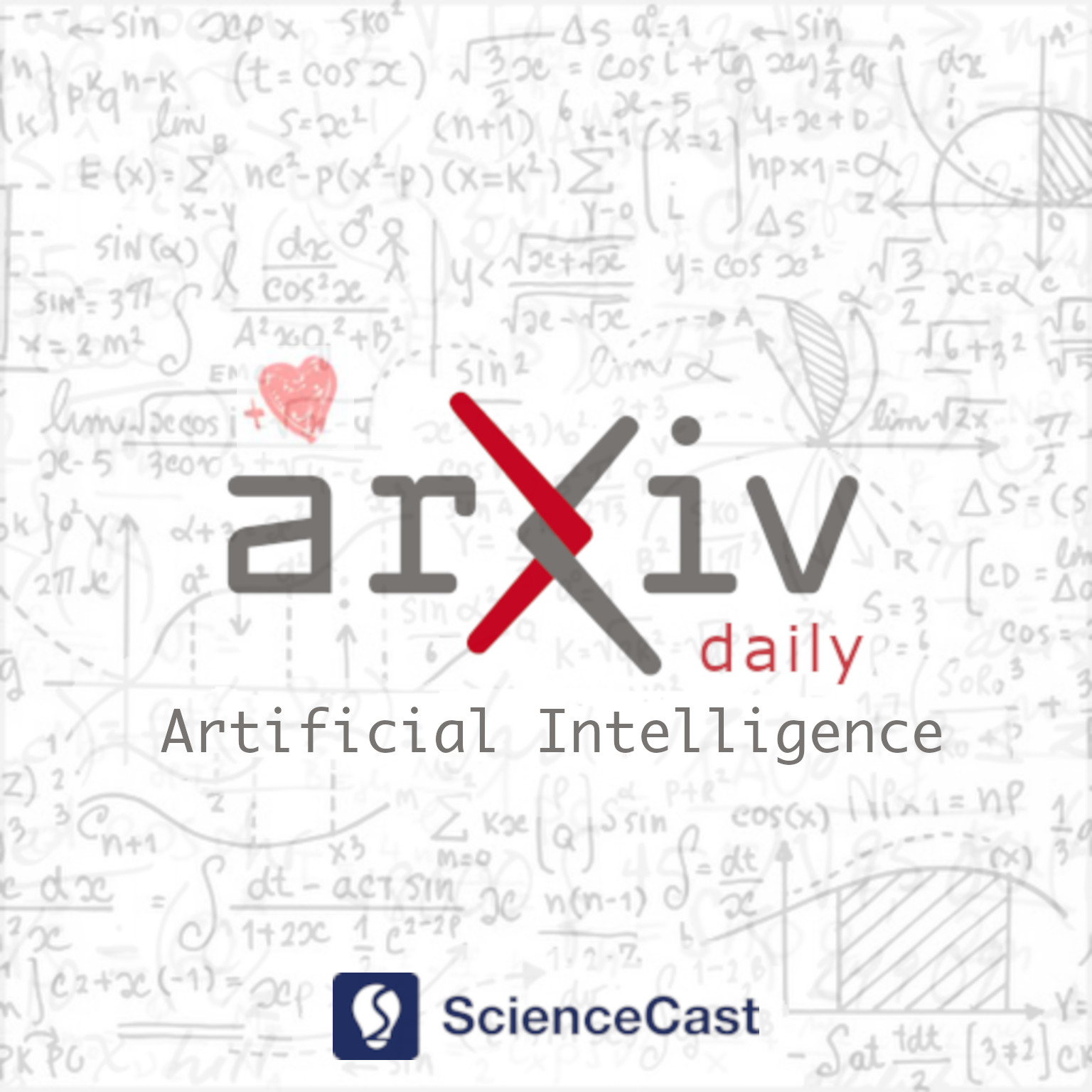
Artificial Intelligence (cs.AI)
Wed, 26 Jul 2023
1.Revisiting the Performance-Explainability Trade-Off in Explainable Artificial Intelligence (XAI)
Authors:Barnaby Crook, Maximilian Schlüter, Timo Speith
Abstract: Within the field of Requirements Engineering (RE), the increasing significance of Explainable Artificial Intelligence (XAI) in aligning AI-supported systems with user needs, societal expectations, and regulatory standards has garnered recognition. In general, explainability has emerged as an important non-functional requirement that impacts system quality. However, the supposed trade-off between explainability and performance challenges the presumed positive influence of explainability. If meeting the requirement of explainability entails a reduction in system performance, then careful consideration must be given to which of these quality aspects takes precedence and how to compromise between them. In this paper, we critically examine the alleged trade-off. We argue that it is best approached in a nuanced way that incorporates resource availability, domain characteristics, and considerations of risk. By providing a foundation for future research and best practices, this work aims to advance the field of RE for AI.
2.A New Perspective on Evaluation Methods for Explainable Artificial Intelligence (XAI)
Authors:Timo Speith, Markus Langer
Abstract: Within the field of Requirements Engineering (RE), the increasing significance of Explainable Artificial Intelligence (XAI) in aligning AI-supported systems with user needs, societal expectations, and regulatory standards has garnered recognition. In general, explainability has emerged as an important non-functional requirement that impacts system quality. However, the supposed trade-off between explainability and performance challenges the presumed positive influence of explainability. If meeting the requirement of explainability entails a reduction in system performance, then careful consideration must be given to which of these quality aspects takes precedence and how to compromise between them. In this paper, we critically examine the alleged trade-off. We argue that it is best approached in a nuanced way that incorporates resource availability, domain characteristics, and considerations of risk. By providing a foundation for future research and best practices, this work aims to advance the field of RE for AI.
3.General Purpose Artificial Intelligence Systems (GPAIS): Properties, Definition, Taxonomy, Open Challenges and Implications
Authors:Isaac Triguero, Daniel Molina, Javier Poyatos, Javier Del Ser, Francisco Herrera
Abstract: Most applications of Artificial Intelligence (AI) are designed for a confined and specific task. However, there are many scenarios that call for a more general AI, capable of solving a wide array of tasks without being specifically designed for them. The term General-Purpose Artificial Intelligence Systems (GPAIS) has been defined to refer to these AI systems. To date, the possibility of an Artificial General Intelligence, powerful enough to perform any intellectual task as if it were human, or even improve it, has remained an aspiration, fiction, and considered a risk for our society. Whilst we might still be far from achieving that, GPAIS is a reality and sitting at the forefront of AI research. This work discusses existing definitions for GPAIS and proposes a new definition that allows for a gradual differentiation among types of GPAIS according to their properties and limitations. We distinguish between closed-world and open-world GPAIS, characterising their degree of autonomy and ability based on several factors such as adaptation to new tasks, competence in domains not intentionally trained for, ability to learn from few data, or proactive acknowledgment of their own limitations. We then propose a taxonomy of approaches to realise GPAIS, describing research trends such as the use of AI techniques to improve another AI or foundation models. As a prime example, we delve into generative AI, aligning them with the terms and concepts presented in the taxonomy. Through the proposed definition and taxonomy, our aim is to facilitate research collaboration across different areas that are tackling general-purpose tasks, as they share many common aspects. Finally, we discuss the current state of GPAIS, its challenges and prospects, implications for our society, and the need for responsible and trustworthy AI systems and regulation, with the goal of providing a holistic view of GPAIS.