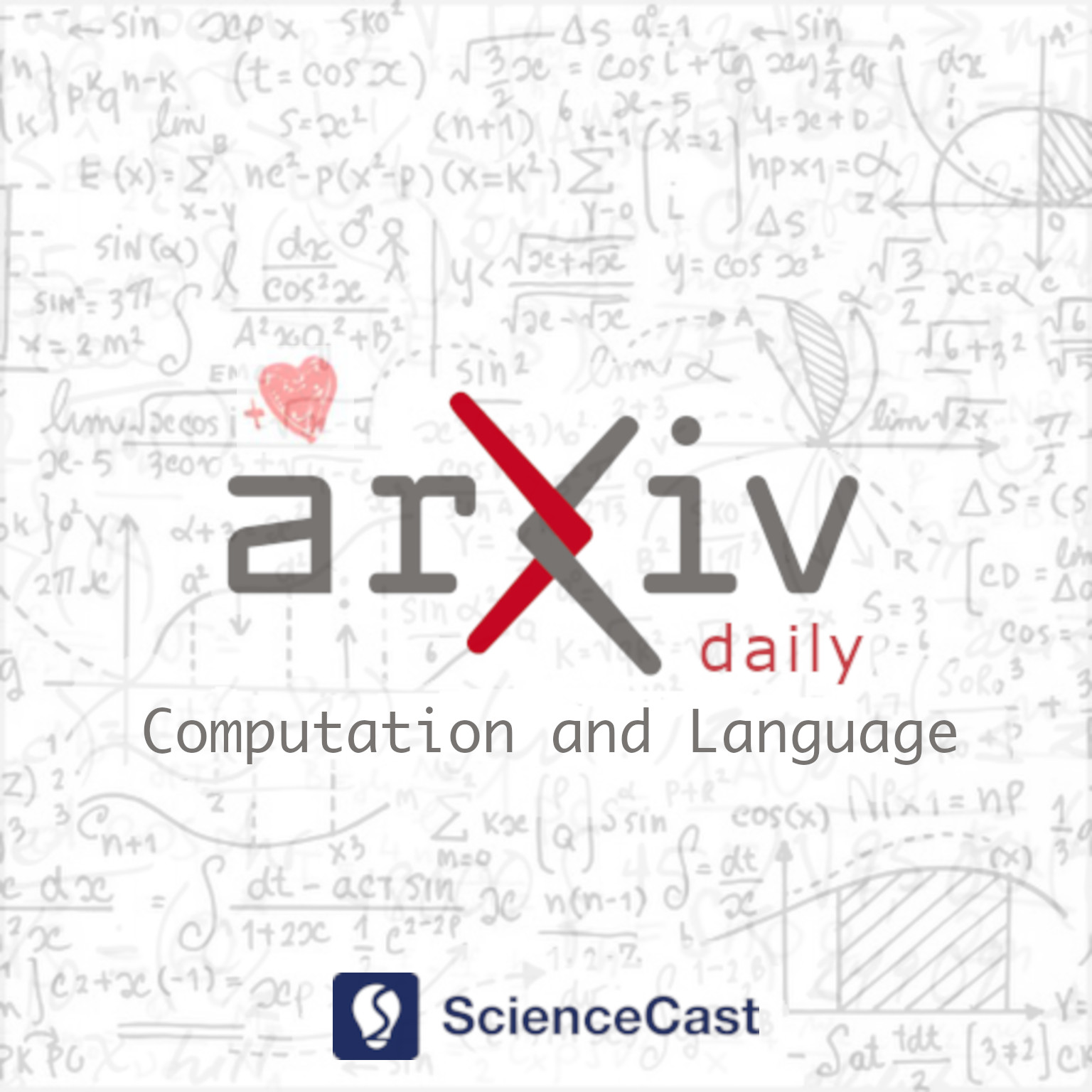
Computation and Language (cs.CL)
Thu, 18 May 2023
1.NoisywikiHow: A Benchmark for Learning with Real-world Noisy Labels in Natural Language Processing
Authors:Tingting Wu, Xiao Ding, Minji Tang, Hao Zhang, Bing Qin, Ting Liu
Abstract: Large-scale datasets in the real world inevitably involve label noise. Deep models can gradually overfit noisy labels and thus degrade model generalization. To mitigate the effects of label noise, learning with noisy labels (LNL) methods are designed to achieve better generalization performance. Due to the lack of suitable datasets, previous studies have frequently employed synthetic label noise to mimic real-world label noise. However, synthetic noise is not instance-dependent, making this approximation not always effective in practice. Recent research has proposed benchmarks for learning with real-world noisy labels. However, the noise sources within may be single or fuzzy, making benchmarks different from data with heterogeneous label noises in the real world. To tackle these issues, we contribute NoisywikiHow, the largest NLP benchmark built with minimal supervision. Specifically, inspired by human cognition, we explicitly construct multiple sources of label noise to imitate human errors throughout the annotation, replicating real-world noise, whose corruption is affected by both ground-truth labels and instances. Moreover, we provide a variety of noise levels to support controlled experiments on noisy data, enabling us to evaluate LNL methods systematically and comprehensively. After that, we conduct extensive multi-dimensional experiments on a broad range of LNL methods, obtaining new and intriguing findings.
2.Flatness-Aware Prompt Selection Improves Accuracy and Sample Efficiency
Authors:Lingfeng Shen, Weiting Tan, Boyuan Zheng, Daniel Khashabi
Abstract: With growing capabilities of large language models, prompting them has become the dominant way to access them. This has motivated the development of strategies for automatically selecting effective language prompts. In this paper, we introduce prompt flatness, a new metric to quantify the expected utility of a language prompt. This metric is inspired by flatness regularization in statistical learning that quantifies the robustness of the model towards its parameter perturbations. We provide theoretical foundations for this metric and its relationship with other prompt selection metrics, providing a comprehensive understanding of existing methods. Empirically, we show that combining prompt flatness with existing metrics improves both performance and sample efficiency. Our metric outperforms the previous prompt selection metrics with an average increase of 5% in accuracy and 10% in Pearson correlation across 6 classification benchmarks.
3.Analyzing Norm Violations in Live-Stream Chat
Authors:Jihyung Moon, Dong-Ho Lee, Hyundong Cho, Woojeong Jin, Chan Young Park, Minwoo Kim, Jonathan May, Jay Pujara, Sungjoon Park
Abstract: Toxic language, such as hate speech, can deter users from participating in online communities and enjoying popular platforms. Previous approaches to detecting toxic language and norm violations have been primarily concerned with conversations from online forums and social media, such as Reddit and Twitter. These approaches are less effective when applied to conversations on live-streaming platforms, such as Twitch and YouTube Live, as each comment is only visible for a limited time and lacks a thread structure that establishes its relationship with other comments. In this work, we share the first NLP study dedicated to detecting norm violations in conversations on live-streaming platforms. We define norm violation categories in live-stream chats and annotate 4,583 moderated comments from Twitch. We articulate several facets of live-stream data that differ from other forums, and demonstrate that existing models perform poorly in this setting. By conducting a user study, we identify the informational context humans use in live-stream moderation, and train models leveraging context to identify norm violations. Our results show that appropriate contextual information can boost moderation performance by 35\%.
4.Counterfactual Debiasing for Generating Factually Consistent Text Summaries
Authors:Chenhe Dong, Yuexiang Xie, Yaliang Li, Ying Shen
Abstract: Despite substantial progress in abstractive text summarization to generate fluent and informative texts, the factual inconsistency in the generated summaries remains an important yet challenging problem to be solved. In this paper, we construct causal graphs for abstractive text summarization and identify the intrinsic causes of the factual inconsistency, i.e., the language bias and irrelevancy bias, and further propose a debiasing framework, named CoFactSum, to alleviate the causal effects of these biases by counterfactual estimation. Specifically, the proposed CoFactSum provides two counterfactual estimation strategies, i.e., Explicit Counterfactual Masking with an explicit dynamic masking strategy, and Implicit Counterfactual Training with an implicit discriminative cross-attention mechanism. Meanwhile, we design a Debiasing Degree Adjustment mechanism to dynamically adapt the debiasing degree at each decoding step. Extensive experiments on two widely-used summarization datasets demonstrate the effectiveness of CoFactSum in enhancing the factual consistency of generated summaries compared with several baselines.
5.Ditto: A Simple and Efficient Approach to Improve Sentence Embeddings
Authors:Qian Chen, Wen Wang, Qinglin Zhang, Siqi Zheng, Chong Deng, Hai Yu, Jiaqing Liu, Yukun Ma, Chong Zhang
Abstract: Prior studies diagnose the anisotropy problem in sentence representations from pre-trained language models, e.g., BERT, without fine-tuning. Our analysis reveals that the sentence embeddings from BERT suffer from a bias towards uninformative words, limiting the performance in semantic textual similarity (STS) tasks. To address this bias, we propose a simple and efficient unsupervised approach, Diagonal Attention Pooling (Ditto), which weights words with model-based importance estimations and computes the weighted average of word representations from pre-trained models as sentence embeddings. Ditto can be easily applied to any pre-trained language model as a postprocessing operation. Compared to prior sentence embedding approaches, Ditto does not add parameters nor requires any learning. Empirical evaluations demonstrate that our proposed Ditto can alleviate the anisotropy problem and improve various pre-trained models on STS tasks.
6.CLEME: Debiasing Multi-reference Evaluation for Grammatical Error Correction
Authors:Jingheng Ye, Yinghui Li, Qingyu Zhou, Yangning Li, Shirong Ma, Hai-Tao Zheng, Ying Shen
Abstract: It is intractable to evaluate the performance of Grammatical Error Correction (GEC) systems since GEC is a highly subjective task. Designing an evaluation metric that is as objective as possible is crucial to the development of GEC task. Previous mainstream evaluation metrics, i.e., reference-based metrics, introduce bias into the multi-reference evaluation because they extract edits without considering the presence of multiple references. To overcome the problem, we propose Chunk-LEvel Multi-reference Evaluation (CLEME) designed to evaluate GEC systems in multi-reference settings. First, CLEME builds chunk sequences with consistent boundaries for the source, the hypothesis and all the references, thus eliminating the bias caused by inconsistent edit boundaries. Then, based on the discovery that there exist boundaries between different grammatical errors, we automatically determine the grammatical error boundaries and compute F$_{0.5}$ scores in a novel way. Our proposed CLEME approach consistently and substantially outperforms existing reference-based GEC metrics on multiple reference sets in both corpus-level and sentence-level settings. Extensive experiments and detailed analyses demonstrate the correctness of our discovery and the effectiveness of our designed evaluation metric.
7.Deep Learning Methods for Extracting Metaphorical Names of Flowers and Plants
Authors:Amal Haddad Haddad, Damith Premasiri, Tharindu Ranasinghe, Ruslan Mitkov
Abstract: The domain of Botany is rich with metaphorical terms. Those terms play an important role in the description and identification of flowers and plants. However, the identification of such terms in discourse is an arduous task. This leads in some cases to committing errors during translation processes and lexicographic tasks. The process is even more challenging when it comes to machine translation, both in the cases of single-word terms and multi-word terms. One of the recent concerns of Natural Language Processing (NLP) applications and Machine Translation (MT) technologies is the automatic identification of metaphor-based words in discourse through Deep Learning (DL). In this study, we seek to fill this gap through the use of thirteen popular transformer based models, as well as ChatGPT, and we show that discriminative models perform better than GPT-3.5 model with our best performer reporting 92.2349% F1 score in metaphoric flower and plant names identification task.
8.A Lexical-aware Non-autoregressive Transformer-based ASR Model
Authors:Chong-En Lin, Kuan-Yu Chen
Abstract: Non-autoregressive automatic speech recognition (ASR) has become a mainstream of ASR modeling because of its fast decoding speed and satisfactory result. To further boost the performance, relaxing the conditional independence assumption and cascading large-scaled pre-trained models are two active research directions. In addition to these strategies, we propose a lexical-aware non-autoregressive Transformer-based (LA-NAT) ASR framework, which consists of an acoustic encoder, a speech-text shared encoder, and a speech-text shared decoder. The acoustic encoder is used to process the input speech features as usual, and the speech-text shared encoder and decoder are designed to train speech and text data simultaneously. By doing so, LA-NAT aims to make the ASR model aware of lexical information, so the resulting model is expected to achieve better results by leveraging the learned linguistic knowledge. A series of experiments are conducted on the AISHELL-1, CSJ, and TEDLIUM 2 datasets. According to the experiments, the proposed LA-NAT can provide superior results than other recently proposed non-autoregressive ASR models. In addition, LA-NAT is a relatively compact model than most non-autoregressive ASR models, and it is about 58 times faster than the classic autoregressive model.
9.TAPIR: Learning Adaptive Revision for Incremental Natural Language Understanding with a Two-Pass Model
Authors:Patrick Kahardipraja, Brielen Madureira, David Schlangen
Abstract: Language is by its very nature incremental in how it is produced and processed. This property can be exploited by NLP systems to produce fast responses, which has been shown to be beneficial for real-time interactive applications. Recent neural network-based approaches for incremental processing mainly use RNNs or Transformers. RNNs are fast but monotonic (cannot correct earlier output, which can be necessary in incremental processing). Transformers, on the other hand, consume whole sequences, and hence are by nature non-incremental. A restart-incremental interface that repeatedly passes longer input prefixes can be used to obtain partial outputs, while providing the ability to revise. However, this method becomes costly as the sentence grows longer. In this work, we propose the Two-pass model for AdaPtIve Revision (TAPIR) and introduce a method to obtain an incremental supervision signal for learning an adaptive revision policy. Experimental results on sequence labelling show that our model has better incremental performance and faster inference speed compared to restart-incremental Transformers, while showing little degradation on full sequences.
10.Large Language Models can be Guided to Evade AI-Generated Text Detection
Authors:Ning Lu, Shengcai Liu, Rui He, Ke Tang
Abstract: Large Language Models (LLMs) have demonstrated exceptional performance in a variety of tasks, including essay writing and question answering. However, it is crucial to address the potential misuse of these models, which can lead to detrimental outcomes such as plagiarism and spamming. Recently, several detectors have been proposed, including fine-tuned classifiers and various statistical methods. In this study, we reveal that with the aid of carefully crafted prompts, LLMs can effectively evade these detection systems. We propose a novel Substitution-based In-Context example Optimization method (SICO) to automatically generate such prompts. On three real-world tasks where LLMs can be misused, SICO successfully enables ChatGPT to evade six existing detectors, causing a significant 0.54 AUC drop on average. Surprisingly, in most cases these detectors perform even worse than random classifiers. These results firmly reveal the vulnerability of existing detectors. Finally, the strong performance of SICO suggests itself as a reliable evaluation protocol for any new detector in this field.
11.Advancing Full-Text Search Lemmatization Techniques with Paradigm Retrieval from OpenCorpora
Authors:Dmitriy Kalugin-Balashov
Abstract: In this paper, we unveil a groundbreaking method to amplify full-text search lemmatization, utilizing the OpenCorpora dataset and a bespoke paradigm retrieval algorithm. Our primary aim is to streamline the extraction of a word's primary form or lemma - a crucial factor in full-text search. Additionally, we propose a compact dictionary storage strategy, significantly boosting the speed and precision of lemma retrieval.
12.TEPrompt: Task Enlightenment Prompt Learning for Implicit Discourse Relation Recognition
Authors:Wei Xiang, Chao Liang, Bang Wang
Abstract: Implicit Discourse Relation Recognition (IDRR) aims at classifying the relation sense between two arguments without an explicit connective. Recently, the ConnPrompt~\cite{Wei.X:et.al:2022:COLING} has leveraged the powerful prompt learning for IDRR based on the fusion of multi-prompt decisions from three different yet much similar connective prediction templates. Instead of multi-prompt ensembling, we propose to design auxiliary tasks with enlightened prompt learning for the IDRR task. Although an auxiliary task is not used to directly output final prediction, we argue that during the joint training some of its learned features can be useful to boost the main task. In light of such motivations, we propose a task enlightenment prompt learning model, called TEPrompt, to fuse learned features from three related tasks for IDRR. In particular, the TEPrompt contains three tasks, viz., Discourse Relation Recognition (DRR), Sense Semantics Classification (SSC) and Annotated Connective Prediction (ACP), each with a unique prompt template and an answer space. In the training phase, we jointly train three prompt learning tasks with shared argument representation. In the testing phase, we only take the DRR output with fused features as the final IDRR decision. Experiments with the same conditions have shown that the proposed TEPrompt outperforms the ConnPrompt. This can be attributed to the promoted decision features and language models benefited from joint-training of auxiliary tasks.
13.EventNet-ITA: Italian Frame Parsing for Events
Authors:Marco Rovera
Abstract: This paper introduces EventNet-ITA, a large, multi-domain corpus annotated with event frames for Italian, and presents an efficient approach for multi-label Frame Parsing. The approach is then evaluated on the dataset. Covering a wide range of individual, social and historical phenomena, the main contribution of EventNet-ITA is to provide the research community with a resource for textual event mining and a novel and extensive tool for Frame Parsing in Italian.
14.Take a Break in the Middle: Investigating Subgoals towards Hierarchical Script Generation
Authors:Xinze Li, Yixin Cao, Muhao Chen, Aixin Sun
Abstract: Goal-oriented Script Generation is a new task of generating a list of steps that can fulfill the given goal. In this paper, we propose to extend the task from the perspective of cognitive theory. Instead of a simple flat structure, the steps are typically organized hierarchically - Human often decompose a complex task into subgoals, where each subgoal can be further decomposed into steps. To establish the benchmark, we contribute a new dataset, propose several baseline methods, and set up evaluation metrics. Both automatic and human evaluation verify the high-quality of dataset, as well as the effectiveness of incorporating subgoals into hierarchical script generation. Furthermore, We also design and evaluate the model to discover subgoal, and find that it is a bit more difficult to decompose the goals than summarizing from segmented steps.
15.Emergent Communication with Attention
Authors:Ryokan Ri, Ryo Ueda, Jason Naradowsky
Abstract: To develop computational agents that better communicate using their own emergent language, we endow the agents with an ability to focus their attention on particular concepts in the environment. Humans often understand an object or scene as a composite of concepts and those concepts are further mapped onto words. We implement this intuition as cross-modal attention mechanisms in Speaker and Listener agents in a referential game and show attention leads to more compositional and interpretable emergent language. We also demonstrate how attention aids in understanding the learned communication protocol by investigating the attention weights associated with each message symbol and the alignment of attention weights between Speaker and Listener agents. Overall, our results suggest that attention is a promising mechanism for developing more human-like emergent language.
16.Causal Document-Grounded Dialogue Pre-training
Authors:Yingxiu Zhao, Bowen Yu, Haiyang Yu, Bowen Li, Chao Wang, Fei Huang, Yongbin Li, Nevin L. Zhang
Abstract: The goal of document-grounded dialogue (DocGD) is to generate a response by grounding the evidence in a supporting document in accordance with the dialogue context. This process involves four variables that are causally connected. Recently, task-specific pre-training has greatly boosted performances on many downstream tasks. Existing DocGD methods, however, continue to rely on general pre-trained language models without a specifically tailored pre-training approach that explicitly captures the causal relationships. To tackle this issue, we are the first to present a causally-complete dataset construction strategy for building million-level DocGD pre-training corpora. To better capture causality, we further propose a causally-perturbed pre-training strategy, which introduces causal perturbations on the variables and optimizes the overall causal effect. Experiments on three benchmark datasets demonstrate that our causal pre-training achieves considerable and consistent improvements under fully-supervised, low-resource, few-shot, and zero-shot settings.
17.Multilingual Event Extraction from Historical Newspaper Adverts
Authors:Nadav Borenstein, Natalia da Silva Perez, Isabelle Augenstein
Abstract: NLP methods can aid historians in analyzing textual materials in greater volumes than manually feasible. Developing such methods poses substantial challenges though. First, acquiring large, annotated historical datasets is difficult, as only domain experts can reliably label them. Second, most available off-the-shelf NLP models are trained on modern language texts, rendering them significantly less effective when applied to historical corpora. This is particularly problematic for less well studied tasks, and for languages other than English. This paper addresses these challenges while focusing on the under-explored task of event extraction from a novel domain of historical texts. We introduce a new multilingual dataset in English, French, and Dutch composed of newspaper ads from the early modern colonial period reporting on enslaved people who liberated themselves from enslavement. We find that: 1) even with scarce annotated data, it is possible to achieve surprisingly good results by formulating the problem as an extractive QA task and leveraging existing datasets and models for modern languages; and 2) cross-lingual low-resource learning for historical languages is highly challenging, and machine translation of the historical datasets to the considered target languages is, in practice, often the best-performing solution.
18.On the Off-Target Problem of Zero-Shot Multilingual Neural Machine Translation
Authors:Liang Chen, Shuming Ma, Dongdong Zhang, Furu Wei, Baobao Chang
Abstract: While multilingual neural machine translation has achieved great success, it suffers from the off-target issue, where the translation is in the wrong language. This problem is more pronounced on zero-shot translation tasks. In this work, we find that failing in encoding discriminative target language signal will lead to off-target and a closer lexical distance (i.e., KL-divergence) between two languages' vocabularies is related with a higher off-target rate. We also find that solely isolating the vocab of different languages in the decoder can alleviate the problem. Motivated by the findings, we propose Language Aware Vocabulary Sharing (LAVS), a simple and effective algorithm to construct the multilingual vocabulary, that greatly alleviates the off-target problem of the translation model by increasing the KL-divergence between languages. We conduct experiments on a multilingual machine translation benchmark in 11 languages. Experiments show that the off-target rate for 90 translation tasks is reduced from 29\% to 8\%, while the overall BLEU score is improved by an average of 1.9 points without extra training cost or sacrificing the supervised directions' performance. We release the code at \href{https://github.com/chenllliang/Off-Target-MNMT}{https://github.com/chenllliang/Off-Target-MNMT} for reproduction.
19.Making More of Little Data: Improving Low-Resource Automatic Speech Recognition Using Data Augmentation
Authors:Martijn Bartelds, Nay San, Bradley McDonnell, Dan Jurafsky, Martijn Wieling
Abstract: The performance of automatic speech recognition (ASR) systems has advanced substantially in recent years, particularly for languages for which a large amount of transcribed speech is available. Unfortunately, for low-resource languages, such as minority languages, regional languages or dialects, ASR performance generally remains much lower. In this study, we investigate whether data augmentation techniques could help improve low-resource ASR performance, focusing on four typologically diverse minority languages or language variants (West Germanic: Gronings, West-Frisian; Malayo-Polynesian: Besemah, Nasal). For all four languages, we examine the use of self-training, where an ASR system trained with the available human-transcribed data is used to generate transcriptions, which are then combined with the original data to train a new ASR system. For Gronings, for which there was a pre-existing text-to-speech (TTS) system available, we also examined the use of TTS to generate ASR training data from text-only sources. We find that using a self-training approach consistently yields improved performance (a relative WER reduction up to 20.5% compared to using an ASR system trained on 24 minutes of manually transcribed speech). The performance gain from TTS augmentation for Gronings was even stronger (up to 25.5% relative reduction in WER compared to a system based on 24 minutes of manually transcribed speech). In sum, our results show the benefit of using self-training or (if possible) TTS-generated data as an efficient solution to overcome the limitations of data availability for resource-scarce languages in order to improve ASR performance.
20.NollySenti: Leveraging Transfer Learning and Machine Translation for Nigerian Movie Sentiment Classification
Authors:Iyanuoluwa Shode, David Ifeoluwa Adelani, Jing Peng, Anna Feldman
Abstract: Africa has over 2000 indigenous languages but they are under-represented in NLP research due to lack of datasets. In recent years, there have been progress in developing labeled corpora for African languages. However, they are often available in a single domain and may not generalize to other domains. In this paper, we focus on the task of sentiment classification for cross domain adaptation. We create a new dataset, NollySenti - based on the Nollywood movie reviews for five languages widely spoken in Nigeria (English, Hausa, Igbo, Nigerian-Pidgin, and Yoruba. We provide an extensive empirical evaluation using classical machine learning methods and pre-trained language models. Leveraging transfer learning, we compare the performance of cross-domain adaptation from Twitter domain, and cross-lingual adaptation from English language. Our evaluation shows that transfer from English in the same target domain leads to more than 5% improvement in accuracy compared to transfer from Twitter in the same language. To further mitigate the domain difference, we leverage machine translation (MT) from English to other Nigerian languages, which leads to a further improvement of 7% over cross-lingual evaluation. While MT to low-resource languages are often of low quality, through human evaluation, we show that most of the translated sentences preserve the sentiment of the original English reviews.
21.Multi-CrossRE A Multi-Lingual Multi-Domain Dataset for Relation Extraction
Authors:Elisa Bassignana, Filip Ginter, Sampo Pyysalo, Rob van der Goot, Barbara Plank
Abstract: Most research in Relation Extraction (RE) involves the English language, mainly due to the lack of multi-lingual resources. We propose Multi-CrossRE, the broadest multi-lingual dataset for RE, including 26 languages in addition to English, and covering six text domains. Multi-CrossRE is a machine translated version of CrossRE (Bassignana and Plank, 2022), with a sub-portion including more than 200 sentences in seven diverse languages checked by native speakers. We run a baseline model over the 26 new datasets and--as sanity check--over the 26 back-translations to English. Results on the back-translated data are consistent with the ones on the original English CrossRE, indicating high quality of the translation and the resulting dataset.
22.Less is More! A slim architecture for optimal language translation
Authors:Luca Herranz-Celotti, Ermal Rrapaj
Abstract: The softmax attention mechanism has emerged as a noteworthy development in the field of Artificial Intelligence research, building on the successes of Transformer-based architectures. However, their ever increasing sizes necessitate ever increasing computational memory, that limits their usage. We propose KgV, a sigmoid gating mechanism that, in conjunction with softmax attention, significantly boosts performance without increasing architecture size. To amend the size requirements, we leverage Tensor Chains to identify and prune the excess parameters. We find that such excess resides primarily within the embedding layer, and not in the output linear layer. To further improve embedding and significantly reduce parameters, we introduce H-SoftPOS, a hierarchical embedding layer which simultaneously enhances performance. Remarkably, on the WMT14 English-German validation set, our approach yields a threefold reduction in perplexity, surpassing the current state-of-the-art, while reducing parameter counts also by a factor of 3. When we further reduce the number of parameters up to sevenfold, we can still achieve a 21\% decrease in perplexity with respect to the baseline Transformer. To understand generalization capabilities, we conduct experiments on the 7 language pairs of the WMT17 dataset. Our method outperforms existing techniques in terms of test loss while simultaneously halving the number of parameters. Moreover, we observe a 70 times reduction in variance with respect to the prior state-of-the-art. In conclusion, our proposed method yields significant improvements in performance and much lower memory cost. We call the resulting architecture Anthe.
23.How does the task complexity of masked pretraining objectives affect downstream performance?
Authors:Atsuki Yamaguchi, Hiroaki Ozaki, Terufumi Morishita, Gaku Morio, Yasuhiro Sogawa
Abstract: Masked language modeling (MLM) is a widely used self-supervised pretraining objective, where a model needs to predict an original token that is replaced with a mask given contexts. Although simpler and computationally efficient pretraining objectives, e.g., predicting the first character of a masked token, have recently shown comparable results to MLM, no objectives with a masking scheme actually outperform it in downstream tasks. Motivated by the assumption that their lack of complexity plays a vital role in the degradation, we validate whether more complex masked objectives can achieve better results and investigate how much complexity they should have to perform comparably to MLM. Our results using GLUE, SQuAD, and Universal Dependencies benchmarks demonstrate that more complicated objectives tend to show better downstream results with at least half of the MLM complexity needed to perform comparably to MLM. Finally, we discuss how we should pretrain a model using a masked objective from the task complexity perspective.
24.The Web Can Be Your Oyster for Improving Large Language Models
Authors:Junyi Li, Tianyi Tang, Wayne Xin Zhao, Jingyuan Wang, Jian-Yun Nie, Ji-Rong Wen
Abstract: Large language models (LLMs) encode a large amount of world knowledge. However, as such knowledge is frozen at the time of model training, the models become static and limited by the training data at that time. In order to further improve the capacity of LLMs for knowledge-intensive tasks, we consider augmenting LLMs with the large-scale web using search engine. Unlike previous augmentation sources (e.g., Wikipedia data dump), the web provides broader, more comprehensive and constantly updated information. In this paper, we present a web-augmented LLM UNIWEB, which is trained over 16 knowledge-intensive tasks in a unified text-to-text format. Instead of simply using the retrieved contents from web, our approach has made two major improvements. Firstly, we propose an adaptive search engine assisted learning method that can self-evaluate the confidence level of LLM's predictions, and adaptively determine when to refer to the web for more data, which can avoid useless or noisy augmentation from web. Secondly, we design a pretraining task, i.e., continual knowledge learning, based on salient spans prediction, to reduce the discrepancy between the encoded and retrieved knowledge. Experiments on a wide range of knowledge-intensive tasks show that our model significantly outperforms previous retrieval-augmented methods.
25.SpeechGPT: Empowering Large Language Models with Intrinsic Cross-Modal Conversational Abilities
Authors:Dong Zhang, Shimin Li, Xin Zhang, Jun Zhan, Pengyu Wang, Yaqian Zhou, Xipeng Qiu
Abstract: Multi-modal large language models are regarded as a crucial step towards Artificial General Intelligence (AGI) and have garnered significant interest with the emergence of ChatGPT. However, current speech-language models typically adopt the cascade paradigm, preventing inter-modal knowledge transfer. In this paper, we propose SpeechGPT, a large language model with intrinsic cross-modal conversational abilities, capable of perceiving and generating multi-model content. With discrete speech representations, we first construct SpeechInstruct, a large-scale cross-modal speech instruction dataset. Additionally, we employ a three-stage training strategy that includes modality-adaptation pre-training, cross-modal instruction fine-tuning, and chain-of-modality instruction fine-tuning. The experimental results demonstrate that SpeechGPT has an impressive capacity to follow multi-modal human instructions and highlight the potential of handling multiple modalities with one model. Demos are shown in https://0nutation.github.io/SpeechGPT.github.io/.
26.Taxonomy Completion with Probabilistic Scorer via Box Embedding
Authors:Wei Xue, Yongliang Shen, Wenqi Ren, Jietian Guo, Siliang Pu, Weiming Lu
Abstract: Taxonomy completion, a task aimed at automatically enriching an existing taxonomy with new concepts, has gained significant interest in recent years. Previous works have introduced complex modules, external information, and pseudo-leaves to enrich the representation and unify the matching process of attachment and insertion. While they have achieved good performance, these introductions may have brought noise and unfairness during training and scoring. In this paper, we present TaxBox, a novel framework for taxonomy completion that maps taxonomy concepts to box embeddings and employs two probabilistic scorers for concept attachment and insertion, avoiding the need for pseudo-leaves. Specifically, TaxBox consists of three components: (1) a graph aggregation module to leverage the structural information of the taxonomy and two lightweight decoders that map features to box embedding and capture complex relationships between concepts; (2) two probabilistic scorers that correspond to attachment and insertion operations and ensure the avoidance of pseudo-leaves; and (3) three learning objectives that assist the model in mapping concepts more granularly onto the box embedding space. Experimental results on four real-world datasets suggest that TaxBox outperforms baseline methods by a considerable margin and surpasses previous state-of-art methods to a certain extent.
27.Silver Syntax Pre-training for Cross-Domain Relation Extraction
Authors:Elisa Bassignana, Filip Ginter, Sampo Pyysalo, Rob van der Goot, Barbara Plank
Abstract: Relation Extraction (RE) remains a challenging task, especially when considering realistic out-of-domain evaluations. One of the main reasons for this is the limited training size of current RE datasets: obtaining high-quality (manually annotated) data is extremely expensive and cannot realistically be repeated for each new domain. An intermediate training step on data from related tasks has shown to be beneficial across many NLP tasks.However, this setup still requires supplementary annotated data, which is often not available. In this paper, we investigate intermediate pre-training specifically for RE. We exploit the affinity between syntactic structure and semantic RE, and identify the syntactic relations which are closely related to RE by being on the shortest dependency path between two entities. We then take advantage of the high accuracy of current syntactic parsers in order to automatically obtain large amounts of low-cost pre-training data. By pre-training our RE model on the relevant syntactic relations, we are able to outperform the baseline in five out of six cross-domain setups, without any additional annotated data.
28.Generalized Multiple Intent Conditioned Slot Filling
Authors:Harshil Shah, Arthur Wilcke, Marius Cobzarenco, Cristi Cobzarenco, Edward Challis, David Barber
Abstract: Natural language understanding includes the tasks of intent detection (identifying a user's objectives) and slot filling (extracting the entities relevant to those objectives). Prior slot filling methods assume that each intent type cannot occur more than once within a message, however this is often not a valid assumption for real-world settings. In this work, we generalize slot filling by removing the constraint of unique intents in a message. We cast this as a JSON generation task and approach it using a language model. We create a pre-training dataset by combining DBpedia and existing slot filling datasets that we convert for JSON generation. We also generate an in-domain dataset using GPT-3. We train T5 models for this task (with and without exemplars in the prompt) and find that both training datasets improve performance, and that the model is able to generalize to intent types not seen during training.
29.Uncertainty Guided Label Denoising for Document-level Distant Relation Extraction
Authors:Qi Sun, Kun Huang, Xiaocui Yang, Pengfei Hong, Kun Zhang, Soujanya Poria
Abstract: Document-level relation extraction (DocRE) aims to infer complex semantic relations among entities in a document. Distant supervision (DS) is able to generate massive auto-labeled data, which can improve DocRE performance. Recent works leverage pseudo labels generated by the pre-denoising model to reduce noise in DS data. However, unreliable pseudo labels bring new noise, e.g., adding false pseudo labels and losing correct DS labels. Therefore, how to select effective pseudo labels to denoise DS data is still a challenge in document-level distant relation extraction. To tackle this issue, we introduce uncertainty estimation technology to determine whether pseudo labels can be trusted. In this work, we propose a Document-level distant Relation Extraction framework with Uncertainty Guided label denoising, UGDRE. Specifically, we propose a novel instance-level uncertainty estimation method, which measures the reliability of the pseudo labels with overlapping relations. By further considering the long-tail problem, we design dynamic uncertainty thresholds for different types of relations to filter high-uncertainty pseudo labels. We conduct experiments on two public datasets. Our framework outperforms strong baselines by 1.91 F1 and 2.28 Ign F1 on the RE-DocRED dataset.
30.Trading Syntax Trees for Wordpieces: Target-oriented Opinion Words Extraction with Wordpieces and Aspect Enhancement
Authors:Samuel Mensah, Kai Sun, Nikolaos Aletras
Abstract: State-of-the-art target-oriented opinion word extraction (TOWE) models typically use BERT-based text encoders that operate on the word level, along with graph convolutional networks (GCNs) that incorporate syntactic information extracted from syntax trees. These methods achieve limited gains with GCNs and have difficulty using BERT wordpieces. Meanwhile, BERT wordpieces are known to be effective at representing rare words or words with insufficient context information. To address this issue, this work trades syntax trees for BERT wordpieces by entirely removing the GCN component from the methods' architectures. To enhance TOWE performance, we tackle the issue of aspect representation loss during encoding. Instead of solely utilizing a sentence as the input, we use a sentence-aspect pair. Our relatively simple approach achieves state-of-the-art results on benchmark datasets and should serve as a strong baseline for further research.
31.Learning In-context Learning for Named Entity Recognition
Authors:Jiawei Chen, Yaojie Lu, Hongyu Lin, Jie Lou, Wei Jia, Dai Dai, Hua Wu, Boxi Cao, Xianpei Han, Le Sun
Abstract: Named entity recognition in real-world applications suffers from the diversity of entity types, the emergence of new entity types, and the lack of high-quality annotations. To address the above problems, this paper proposes an in-context learning-based NER approach, which can effectively inject in-context NER ability into PLMs and recognize entities of novel types on-the-fly using only a few demonstrative instances. Specifically, we model PLMs as a meta-function $\mathcal{ \lambda_ {\text{instruction, demonstrations, text}}. M}$, and a new entity extractor can be implicitly constructed by applying new instruction and demonstrations to PLMs, i.e., $\mathcal{ (\lambda . M) }$(instruction, demonstrations) $\to$ $\mathcal{F}$ where $\mathcal{F}$ will be a new entity extractor, i.e., $\mathcal{F}$: text $\to$ entities. To inject the above in-context NER ability into PLMs, we propose a meta-function pre-training algorithm, which pre-trains PLMs by comparing the (instruction, demonstration)-initialized extractor with a surrogate golden extractor. Experimental results on 4 few-shot NER datasets show that our method can effectively inject in-context NER ability into PLMs and significantly outperforms the PLMs+fine-tuning counterparts.
32.Self-supervised Fine-tuning for Improved Content Representations by Speaker-invariant Clustering
Authors:Heng-Jui Chang, Alexander H. Liu, James Glass
Abstract: Self-supervised speech representation models have succeeded in various tasks, but improving them for content-related problems using unlabeled data is challenging. We propose speaker-invariant clustering (Spin), a novel self-supervised learning method that clusters speech representations and performs swapped prediction between the original and speaker-perturbed utterances. Spin disentangles speaker information and preserves content representations with just 45 minutes of fine-tuning on a single GPU. Spin improves pre-trained networks and outperforms prior methods in speech recognition and acoustic unit discovery.
33.A Comparative Study on E-Branchformer vs Conformer in Speech Recognition, Translation, and Understanding Tasks
Authors:Yifan Peng, Kwangyoun Kim, Felix Wu, Brian Yan, Siddhant Arora, William Chen, Jiyang Tang, Suwon Shon, Prashant Sridhar, Shinji Watanabe
Abstract: Conformer, a convolution-augmented Transformer variant, has become the de facto encoder architecture for speech processing due to its superior performance in various tasks, including automatic speech recognition (ASR), speech translation (ST) and spoken language understanding (SLU). Recently, a new encoder called E-Branchformer has outperformed Conformer in the LibriSpeech ASR benchmark, making it promising for more general speech applications. This work compares E-Branchformer and Conformer through extensive experiments using different types of end-to-end sequence-to-sequence models. Results demonstrate that E-Branchformer achieves comparable or better performance than Conformer in almost all evaluation sets across 15 ASR, 2 ST, and 3 SLU benchmarks, while being more stable during training. We will release our training configurations and pre-trained models for reproducibility, which can benefit the speech community.
34.Cross-modality Data Augmentation for End-to-End Sign Language Translation
Authors:Jinhui Ye, Wenxiang Jiao, Xing Wang, Zhaopeng Tu, Hui Xiong
Abstract: End-to-end sign language translation (SLT) aims to convert sign language videos into spoken language texts directly without intermediate representations. It has been a challenging task due to the modality gap between sign videos and texts and the data scarcity of labeled data. To tackle these challenges, we propose a novel Cross-modality Data Augmentation (XmDA) framework to transfer the powerful gloss-to-text translation capabilities to end-to-end sign language translation (i.e. video-to-text) by exploiting pseudo gloss-text pairs from the sign gloss translation model. Specifically, XmDA consists of two key components, namely, cross-modality mix-up and cross-modality knowledge distillation. The former explicitly encourages the alignment between sign video features and gloss embeddings to bridge the modality gap. The latter utilizes the generation knowledge from gloss-to-text teacher models to guide the spoken language text generation. Experimental results on two widely used SLT datasets, i.e., PHOENIX-2014T and CSL-Daily, demonstrate that the proposed XmDA framework significantly and consistently outperforms the baseline models. Extensive analyses confirm our claim that XmDA enhances spoken language text generation by reducing the representation distance between videos and texts, as well as improving the processing of low-frequency words and long sentences.
35.mLongT5: A Multilingual and Efficient Text-To-Text Transformer for Longer Sequences
Authors:David Uthus, Santiago Ontañón, Joshua Ainslie, Mandy Guo
Abstract: We present our work on developing a multilingual, efficient text-to-text transformer that is suitable for handling long inputs. This model, called mLongT5, builds upon the architecture of LongT5, while leveraging the multilingual datasets used for pretraining mT5 and the pretraining tasks of UL2. We evaluate this model on a variety of multilingual summarization and question-answering tasks, and the results show stronger performance for mLongT5 when compared to existing multilingual models such as mBART or M-BERT.
36.Exploiting Biased Models to De-bias Text: A Gender-Fair Rewriting Model
Authors:Chantal Amrhein, Florian Schottmann, Rico Sennrich, Samuel Läubli
Abstract: Natural language generation models reproduce and often amplify the biases present in their training data. Previous research explored using sequence-to-sequence rewriting models to transform biased model outputs (or original texts) into more gender-fair language by creating pseudo training data through linguistic rules. However, this approach is not practical for languages with more complex morphology than English. We hypothesise that creating training data in the reverse direction, i.e. starting from gender-fair text, is easier for morphologically complex languages and show that it matches the performance of state-of-the-art rewriting models for English. To eliminate the rule-based nature of data creation, we instead propose using machine translation models to create gender-biased text from real gender-fair text via round-trip translation. Our approach allows us to train a rewriting model for German without the need for elaborate handcrafted rules. The outputs of this model increased gender-fairness as shown in a human evaluation study.
37.Discourse Centric Evaluation of Machine Translation with a Densely Annotated Parallel Corpus
Authors:Yuchen Eleanor Jiang, Tianyu Liu, Shuming Ma, Dongdong Zhang, Mrinmaya Sachan, Ryan Cotterell
Abstract: Several recent papers claim human parity at sentence-level Machine Translation (MT), especially in high-resource languages. Thus, in response, the MT community has, in part, shifted its focus to document-level translation. Translating documents requires a deeper understanding of the structure and meaning of text, which is often captured by various kinds of discourse phenomena such as consistency, coherence, and cohesion. However, this renders conventional sentence-level MT evaluation benchmarks inadequate for evaluating the performance of context-aware MT systems. This paper presents a new dataset with rich discourse annotations, built upon the large-scale parallel corpus BWB introduced in Jiang et al. (2022). The new BWB annotation introduces four extra evaluation aspects, i.e., entity, terminology, coreference, and quotation, covering 15,095 entity mentions in both languages. Using these annotations, we systematically investigate the similarities and differences between the discourse structures of source and target languages, and the challenges they pose to MT. We discover that MT outputs differ fundamentally from human translations in terms of their latent discourse structures. This gives us a new perspective on the challenges and opportunities in document-level MT. We make our resource publicly available to spur future research in document-level MT and the generalization to other language translation tasks.
38.Aligning Instruction Tasks Unlocks Large Language Models as Zero-Shot Relation Extractors
Authors:Kai Zhang, Bernal Jiménez Gutiérrez, Yu Su
Abstract: Recent work has shown that fine-tuning large language models (LLMs) on large-scale instruction-following datasets substantially improves their performance on a wide range of NLP tasks, especially in the zero-shot setting. However, even advanced instruction-tuned LLMs still fail to outperform small LMs on relation extraction (RE), a fundamental information extraction task. We hypothesize that instruction-tuning has been unable to elicit strong RE capabilities in LLMs due to RE's low incidence in instruction-tuning datasets, making up less than 1% of all tasks (Wang et al., 2022). To address this limitation, we propose QA4RE, a framework that aligns RE with question answering (QA), a predominant task in instruction-tuning datasets. Comprehensive zero-shot RE experiments over four datasets with two series of instruction-tuned LLMs (six LLMs in total) demonstrate that our QA4RE framework consistently improves LLM performance, strongly verifying our hypothesis and enabling LLMs to outperform strong zero-shot baselines by a large margin. Additionally, we provide thorough experiments and discussions to show the robustness, few-shot effectiveness, and strong transferability of our QA4RE framework. This work illustrates a promising way of adapting LLMs to challenging and underrepresented tasks by aligning these tasks with more common instruction-tuning tasks like QA.
39.Efficient Prompting via Dynamic In-Context Learning
Authors:Wangchunshu Zhou, Yuchen Eleanor Jiang, Ryan Cotterell, Mrinmaya Sachan
Abstract: The primary way of building AI applications is shifting from training specialist models to prompting generalist models. A common practice for prompting generalist models, often referred to as in-context learning, is to append a few examples (demonstrations) to the prompt to help the model better understand the task. While effective, in-context learning can be inefficient because it makes the input prompt much longer, consuming valuable space in the context window and leading to larger computational costs. In this paper, we propose DynaICL, a recipe for efficient prompting with black-box generalist models that dynamically allocate in-context examples according to the input complexity and the computational budget. To achieve this, we train a meta controller that predicts the number of in-context examples suitable for the generalist model to make a good prediction based on the performance-efficiency trade-off for a specific input. We then dynamically allocate the number of demonstrations for an input according to predictions from the meta controller and the given computation budget. Experimental results show that dynamic example allocation helps achieve a better performance-efficiency trade-off in two practical settings where computational resources or the required performance is constrained. Specifically, DynaICL saves up to 46% token budget compared to the common practice that allocates the same number of in-context examples to each input. We also find that a meta controller trained on a certain backbone model and tasks can successfully generalize to unseen models and tasks.
40.TrueTeacher: Learning Factual Consistency Evaluation with Large Language Models
Authors:Zorik Gekhman, Jonathan Herzig, Roee Aharoni, Chen Elkind, Idan Szpektor
Abstract: Factual consistency evaluation is often conducted using Natural Language Inference (NLI) models, yet these models exhibit limited success in evaluating summaries. Previous work improved such models with synthetic training data. However, the data is typically based on perturbed human-written summaries, which often differ in their characteristics from real model-generated summaries and have limited coverage of possible factual errors. Alternatively, large language models (LLMs) have recently shown promising results in directly evaluating generative tasks, but are too computationally expensive for practical use. Motivated by these limitations, we introduce TrueTeacher, a method for generating synthetic data by annotating diverse model-generated summaries using a LLM. Unlike prior work, TrueTeacher does not rely on human-written summaries, and is multilingual by nature. Experiments on the TRUE benchmark show that a student model trained using our data, substantially outperforms both the state-of-the-art model with similar capacity, and the LLM teacher. In a systematic study, we compare TrueTeacher to existing synthetic data generation methods and demonstrate its superiority and robustness to domain-shift. Using the the mFACE dataset, we also show that our method generalizes to multilingual scenarios. Finally, we release a large-scale synthetic dataset with 1.4M examples generated using TrueTeacher.