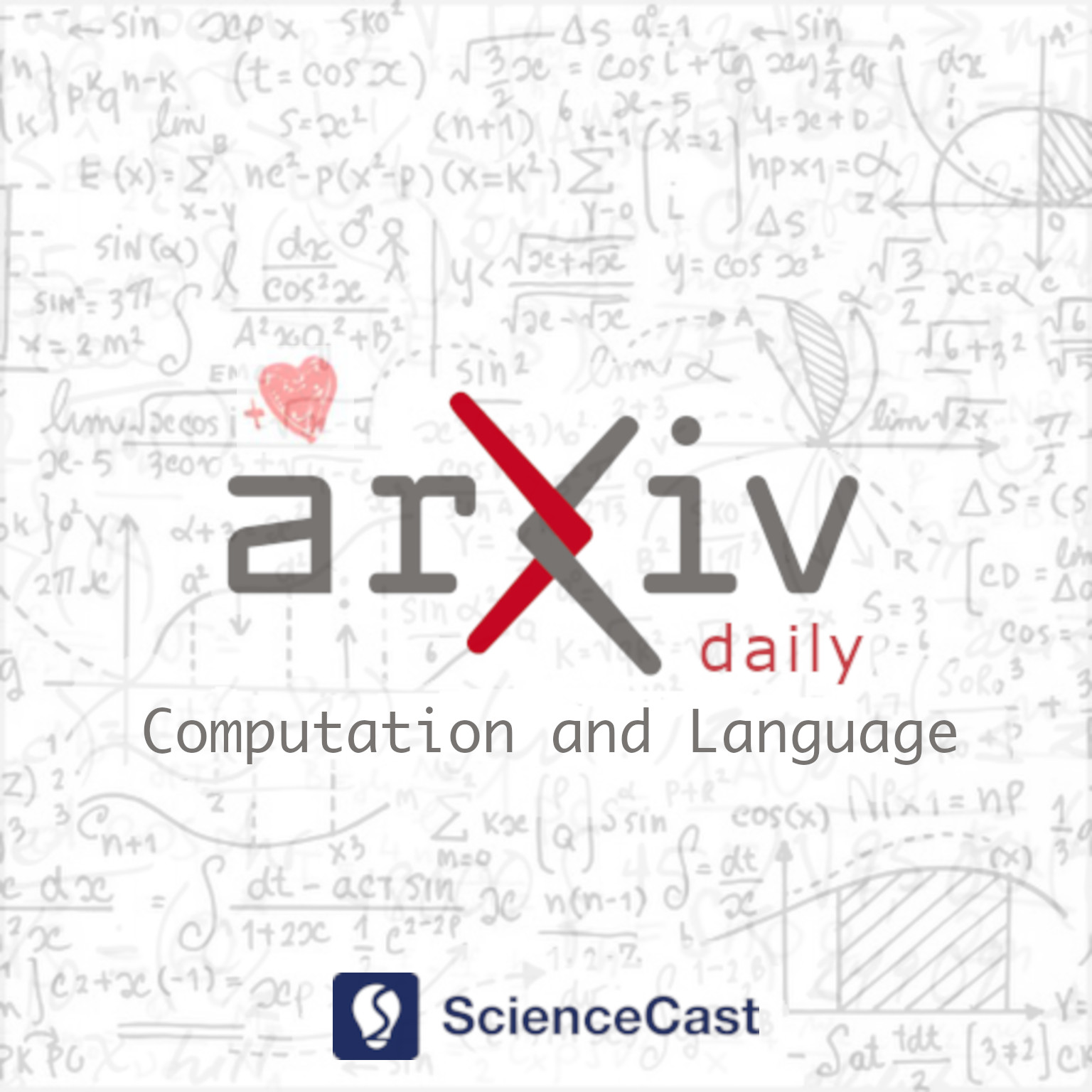
Computation and Language (cs.CL)
Fri, 21 Apr 2023
1.Improving Grounded Language Understanding in a Collaborative Environment by Interacting with Agents Through Help Feedback
Authors:Nikhil Mehta, Milagro Teruel, Patricio Figueroa Sanz, Xin Deng, Ahmed Hassan Awadallah, Julia Kiseleva
Abstract: Many approaches to Natural Language Processing (NLP) tasks often treat them as single-step problems, where an agent receives an instruction, executes it, and is evaluated based on the final outcome. However, human language is inherently interactive, as evidenced by the back-and-forth nature of human conversations. In light of this, we posit that human-AI collaboration should also be interactive, with humans monitoring the work of AI agents and providing feedback that the agent can understand and utilize. Further, the AI agent should be able to detect when it needs additional information and proactively ask for help. Enabling this scenario would lead to more natural, efficient, and engaging human-AI collaborations. In this work, we explore these directions using the challenging task defined by the IGLU competition, an interactive grounded language understanding task in a MineCraft-like world. We explore multiple types of help players can give to the AI to guide it and analyze the impact of this help in AI behavior, resulting in performance improvements.
2.Eyettention: An Attention-based Dual-Sequence Model for Predicting Human Scanpaths during Reading
Authors:Shuwen Deng, David R. Reich, Paul Prasse, Patrick Haller, Tobias Scheffer, Lena A. Jäger
Abstract: Eye movements during reading offer insights into both the reader's cognitive processes and the characteristics of the text that is being read. Hence, the analysis of scanpaths in reading have attracted increasing attention across fields, ranging from cognitive science over linguistics to computer science. In particular, eye-tracking-while-reading data has been argued to bear the potential to make machine-learning-based language models exhibit a more human-like linguistic behavior. However, one of the main challenges in modeling human scanpaths in reading is their dual-sequence nature: the words are ordered following the grammatical rules of the language, whereas the fixations are chronologically ordered. As humans do not strictly read from left-to-right, but rather skip or refixate words and regress to previous words, the alignment of the linguistic and the temporal sequence is non-trivial. In this paper, we develop Eyettention, the first dual-sequence model that simultaneously processes the sequence of words and the chronological sequence of fixations. The alignment of the two sequences is achieved by a cross-sequence attention mechanism. We show that Eyettention outperforms state-of-the-art models in predicting scanpaths. We provide an extensive within- and across-data set evaluation on different languages. An ablation study and qualitative analysis support an in-depth understanding of the model's behavior.
3.Which Factors Predict the Chat Experience of a Natural Language Generation Dialogue Service?
Authors:Eason Chen
Abstract: In this paper, we proposed a conceptual model to predict the chat experience in a natural language generation dialog system. We evaluated the model with 120 participants with Partial Least Squares Structural Equation Modeling (PLS-SEM) and obtained an R-square (R2) with 0.541. The model considers various factors, including the prompts used for generation; coherence, sentiment, and similarity in the conversation; and users' perceived dialog agents' favorability. We then further explore the effectiveness of the subset of our proposed model. The results showed that users' favorability and coherence, sentiment, and similarity in the dialogue are positive predictors of users' chat experience. Moreover, we found users may prefer dialog agents with characteristics of Extroversion, Openness, Conscientiousness, Agreeableness, and Non-Neuroticism. Through our research, an adaptive dialog system might use collected data to infer factors in our model, predict the chat experience for users through these factors, and optimize it by adjusting prompts.
4.Downstream Task-Oriented Neural Tokenizer Optimization with Vocabulary Restriction as Post Processing
Authors:Tatsuya Hiraoka, Tomoya Iwakura
Abstract: This paper proposes a method to optimize tokenization for the performance improvement of already trained downstream models. Our method generates tokenization results attaining lower loss values of a given downstream model on the training data for restricting vocabularies and trains a tokenizer reproducing the tokenization results. Therefore, our method can be applied to variety of tokenization methods, while existing work cannot due to the simultaneous learning of the tokenizer and the downstream model. This paper proposes an example of the BiLSTM-based tokenizer with vocabulary restriction, which can capture wider contextual information for the tokenization process than non-neural-based tokenization methods used in existing work. Experimental results on text classification in Japanese, Chinese, and English text classification tasks show that the proposed method improves performance compared to the existing methods for tokenization optimization.
5.Tokenization Tractability for Human and Machine Learning Model: An Annotation Study
Authors:Tatsuya Hiraoka, Tomoya Iwakura
Abstract: Is tractable tokenization for humans also tractable for machine learning models? This study investigates relations between tractable tokenization for humans (e.g., appropriateness and readability) and one for models of machine learning (e.g., performance on an NLP task). We compared six tokenization methods on the Japanese commonsense question-answering dataset (JCommmonsenseQA in JGLUE). We tokenized question texts of the QA dataset with different tokenizers and compared the performance of human annotators and machine-learning models. Besides,we analyze relationships among the performance, appropriateness of tokenization, and response time to questions. This paper provides a quantitative investigation result that shows the tractable tokenizations for humans and machine learning models are not necessarily the same as each other.
6.Better Sign Language Translation with Monolingual Data
Authors:Ru Peng, Yawen Zeng, Junbo Zhao
Abstract: Sign language translation (SLT) systems, which are often decomposed into video-to-gloss (V2G) recognition and gloss-to-text (G2T) translation through the pivot gloss, heavily relies on the availability of large-scale parallel G2T pairs. However, the manual annotation of pivot gloss, which is a sequence of transcribed written-language words in the order in which they are signed, further exacerbates the scarcity of data for SLT. To address this issue, this paper proposes a simple and efficient rule transformation method to transcribe the large-scale target monolingual data into its pseudo glosses automatically for enhancing the SLT translation. Empirical results show that the proposed approach can significantly improve the performance of SLT, especially achieving state-of-the-art results on two SLT benchmark datasets PHEONIX-WEATHER 2014T and ASLG-PC12. Our code has been released at: https://github.com/pengr/Mono\_SLT.
7.Text2Time: Transformer-based article time period predictor
Authors:Karthick Prasad Gunasekaran, B Chase Babrich, Saurabh Shirodkar, Hee Hwang
Abstract: We explore the problem of predicting the publication period of text document, such as a news article, using the text from that document. In order to do so, we created our own extensive labeled dataset of over 350,000 news articles published by The New York Times over six decades. We then provide an implementation of a simple Naive Bayes baseline model, which surprisingly achieves decent performance in terms of accuracy.Finally, for our approach, we use a pretrained BERT model fine-tuned for the task of text classification. This model exceeds our expectations and provides some very impressive results in terms of accurately classifying news articles into their respective publication decades. The results beat the performance of the few previously tried models for this relatively unexplored task of time prediction from text.
8.LEIA: Linguistic Embeddings for the Identification of Affect
Authors:Segun Taofeek Aroyehun, Lukas Malik, Hannah Metzler, Nikolas Haimerl, Anna Di Natale, David Garcia
Abstract: The wealth of text data generated by social media has enabled new kinds of analysis of emotions with language models. These models are often trained on small and costly datasets of text annotations produced by readers who guess the emotions expressed by others in social media posts. This affects the quality of emotion identification methods due to training data size limitations and noise in the production of labels used in model development. We present LEIA, a model for emotion identification in text that has been trained on a dataset of more than 6 million posts with self-annotated emotion labels for happiness, affection, sadness, anger, and fear. LEIA is based on a word masking method that enhances the learning of emotion words during model pre-training. LEIA achieves macro-F1 values of approximately 73 on three in-domain test datasets, outperforming other supervised and unsupervised methods in a strong benchmark that shows that LEIA generalizes across posts, users, and time periods. We further perform an out-of-domain evaluation on five different datasets of social media and other sources, showing LEIA's robust performance across media, data collection methods, and annotation schemes. Our results show that LEIA generalizes its classification of anger, happiness, and sadness beyond the domain it was trained on. LEIA can be applied in future research to provide better identification of emotions in text from the perspective of the writer. The models produced for this article are publicly available at https://huggingface.co/LEIA
9.Evaluating Transformer Language Models on Arithmetic Operations Using Number Decomposition
Authors:Matteo Muffo, Aldo Cocco, Enrico Bertino
Abstract: In recent years, Large Language Models such as GPT-3 showed remarkable capabilities in performing NLP tasks in the zero and few shot settings. On the other hand, the experiments highlighted the difficulty of GPT-3 in carrying out tasks that require a certain degree of reasoning, such as arithmetic operations. In this paper we evaluate the ability of Transformer Language Models to perform arithmetic operations following a pipeline that, before performing computations, decomposes numbers in units, tens, and so on. We denote the models fine-tuned with this pipeline with the name Calculon and we test them in the task of performing additions, subtractions and multiplications on the same test sets of GPT-3. Results show an increase of accuracy of 63% in the five-digit addition task. Moreover, we demonstrate the importance of the decomposition pipeline introduced, since fine-tuning the same Language Model without decomposing numbers results in 0% accuracy in the five-digit addition task.
10.Information Extraction from Documents: Question Answering vs Token Classification in real-world setups
Authors:Laurent Lam, Pirashanth Ratnamogan, Joël Tang, William Vanhuffel, Fabien Caspani
Abstract: Research in Document Intelligence and especially in Document Key Information Extraction (DocKIE) has been mainly solved as Token Classification problem. Recent breakthroughs in both natural language processing (NLP) and computer vision helped building document-focused pre-training methods, leveraging a multimodal understanding of the document text, layout and image modalities. However, these breakthroughs also led to the emergence of a new DocKIE subtask of extractive document Question Answering (DocQA), as part of the Machine Reading Comprehension (MRC) research field. In this work, we compare the Question Answering approach with the classical token classification approach for document key information extraction. We designed experiments to benchmark five different experimental setups : raw performances, robustness to noisy environment, capacity to extract long entities, fine-tuning speed on Few-Shot Learning and finally Zero-Shot Learning. Our research showed that when dealing with clean and relatively short entities, it is still best to use token classification-based approach, while the QA approach could be a good alternative for noisy environment or long entities use-cases.
11.BERT Based Clinical Knowledge Extraction for Biomedical Knowledge Graph Construction and Analysis
Authors:Ayoub Harnoune, Maryem Rhanoui, Mounia Mikram, Siham Yousfi, Zineb Elkaimbillah, Bouchra El Asri
Abstract: Background : Knowledge is evolving over time, often as a result of new discoveries or changes in the adopted methods of reasoning. Also, new facts or evidence may become available, leading to new understandings of complex phenomena. This is particularly true in the biomedical field, where scientists and physicians are constantly striving to find new methods of diagnosis, treatment and eventually cure. Knowledge Graphs (KGs) offer a real way of organizing and retrieving the massive and growing amount of biomedical knowledge. Objective : We propose an end-to-end approach for knowledge extraction and analysis from biomedical clinical notes using the Bidirectional Encoder Representations from Transformers (BERT) model and Conditional Random Field (CRF) layer. Methods : The approach is based on knowledge graphs, which can effectively process abstract biomedical concepts such as relationships and interactions between medical entities. Besides offering an intuitive way to visualize these concepts, KGs can solve more complex knowledge retrieval problems by simplifying them into simpler representations or by transforming the problems into representations from different perspectives. We created a biomedical Knowledge Graph using using Natural Language Processing models for named entity recognition and relation extraction. The generated biomedical knowledge graphs (KGs) are then used for question answering. Results : The proposed framework can successfully extract relevant structured information with high accuracy (90.7% for Named-entity recognition (NER), 88% for relation extraction (RE)), according to experimental findings based on real-world 505 patient biomedical unstructured clinical notes. Conclusions : In this paper, we propose a novel end-to-end system for the construction of a biomedical knowledge graph from clinical textual using a variation of BERT models.
12.DIN-SQL: Decomposed In-Context Learning of Text-to-SQL with Self-Correction
Authors:Mohammadreza Pourreza, Davood Rafiei
Abstract: We study the problem of decomposing a complex text-to-sql task into smaller sub-tasks and how such a decomposition can significantly improve the performance of Large Language Models (LLMs) in the reasoning process. There is currently a significant gap between the performance of fine-tuned models and prompting approaches using LLMs on challenging text-to-sql datasets such as Spider. We show that SQL queries, despite their declarative structure, can be broken down into sub-problems and the solutions of those sub-problems can be fed into LLMs to significantly improve their performance. Our experiments with three LLMs show that this approach consistently improves their performance by roughly 10%, pushing the accuracy of LLMs towards state-of-the-art, and even beating large fine-tuned models on the holdout Spider dataset.
13.ChatABL: Abductive Learning via Natural Language Interaction with ChatGPT
Authors:Tianyang Zhong, Yaonai Wei, Li Yang, Zihao Wu, Zhengliang Liu, Xiaozheng Wei, Wenjun Li, Junjie Yao, Chong Ma, Xiang Li, Dajiang Zhu, Xi Jiang, Junwei Han, Dinggang Shen, Tianming Liu, Tuo Zhang
Abstract: Large language models (LLMs) such as ChatGPT have recently demonstrated significant potential in mathematical abilities, providing valuable reasoning paradigm consistent with human natural language. However, LLMs currently have difficulty in bridging perception, language understanding and reasoning capabilities due to incompatibility of the underlying information flow among them, making it challenging to accomplish tasks autonomously. On the other hand, abductive learning (ABL) frameworks for integrating the two abilities of perception and reasoning has seen significant success in inverse decipherment of incomplete facts, but it is limited by the lack of semantic understanding of logical reasoning rules and the dependence on complicated domain knowledge representation. This paper presents a novel method (ChatABL) for integrating LLMs into the ABL framework, aiming at unifying the three abilities in a more user-friendly and understandable manner. The proposed method uses the strengths of LLMs' understanding and logical reasoning to correct the incomplete logical facts for optimizing the performance of perceptual module, by summarizing and reorganizing reasoning rules represented in natural language format. Similarly, perceptual module provides necessary reasoning examples for LLMs in natural language format. The variable-length handwritten equation deciphering task, an abstract expression of the Mayan calendar decoding, is used as a testbed to demonstrate that ChatABL has reasoning ability beyond most existing state-of-the-art methods, which has been well supported by comparative studies. To our best knowledge, the proposed ChatABL is the first attempt to explore a new pattern for further approaching human-level cognitive ability via natural language interaction with ChatGPT.
14.Inducing anxiety in large language models increases exploration and bias
Authors:Julian Coda-Forno, Kristin Witte, Akshay K. Jagadish, Marcel Binz, Zeynep Akata, Eric Schulz
Abstract: Large language models are transforming research on machine learning while galvanizing public debates. Understanding not only when these models work well and succeed but also why they fail and misbehave is of great societal relevance. We propose to turn the lens of computational psychiatry, a framework used to computationally describe and modify aberrant behavior, to the outputs produced by these models. We focus on the Generative Pre-Trained Transformer 3.5 and subject it to tasks commonly studied in psychiatry. Our results show that GPT-3.5 responds robustly to a common anxiety questionnaire, producing higher anxiety scores than human subjects. Moreover, GPT-3.5's responses can be predictably changed by using emotion-inducing prompts. Emotion-induction not only influences GPT-3.5's behavior in a cognitive task measuring exploratory decision-making but also influences its behavior in a previously-established task measuring biases such as racism and ableism. Crucially, GPT-3.5 shows a strong increase in biases when prompted with anxiety-inducing text. Thus, it is likely that how prompts are communicated to large language models has a strong influence on their behavior in applied settings. These results progress our understanding of prompt engineering and demonstrate the usefulness of methods taken from computational psychiatry for studying the capable algorithms to which we increasingly delegate authority and autonomy.
15.Emergent and Predictable Memorization in Large Language Models
Authors:Stella Biderman, USVSN Sai Prashanth, Lintang Sutawika, Hailey Schoelkopf, Quentin Anthony, Shivanshu Purohit, Edward Raf
Abstract: Memorization, or the tendency of large language models (LLMs) to output entire sequences from their training data verbatim, is a key concern for safely deploying language models. In particular, it is vital to minimize a model's memorization of sensitive datapoints such as those containing personal identifiable information (PII). The prevalence of such undesirable memorization can pose issues for model trainers, and may even require discarding an otherwise functional model. We therefore seek to predict which sequences will be memorized before a large model's full train-time by extrapolating the memorization behavior of lower-compute trial runs. We measure memorization of the Pythia model suite, and find that intermediate checkpoints are better predictors of a model's memorization behavior than smaller fully-trained models. We additionally provide further novel discoveries on the distribution of memorization scores across models and data.