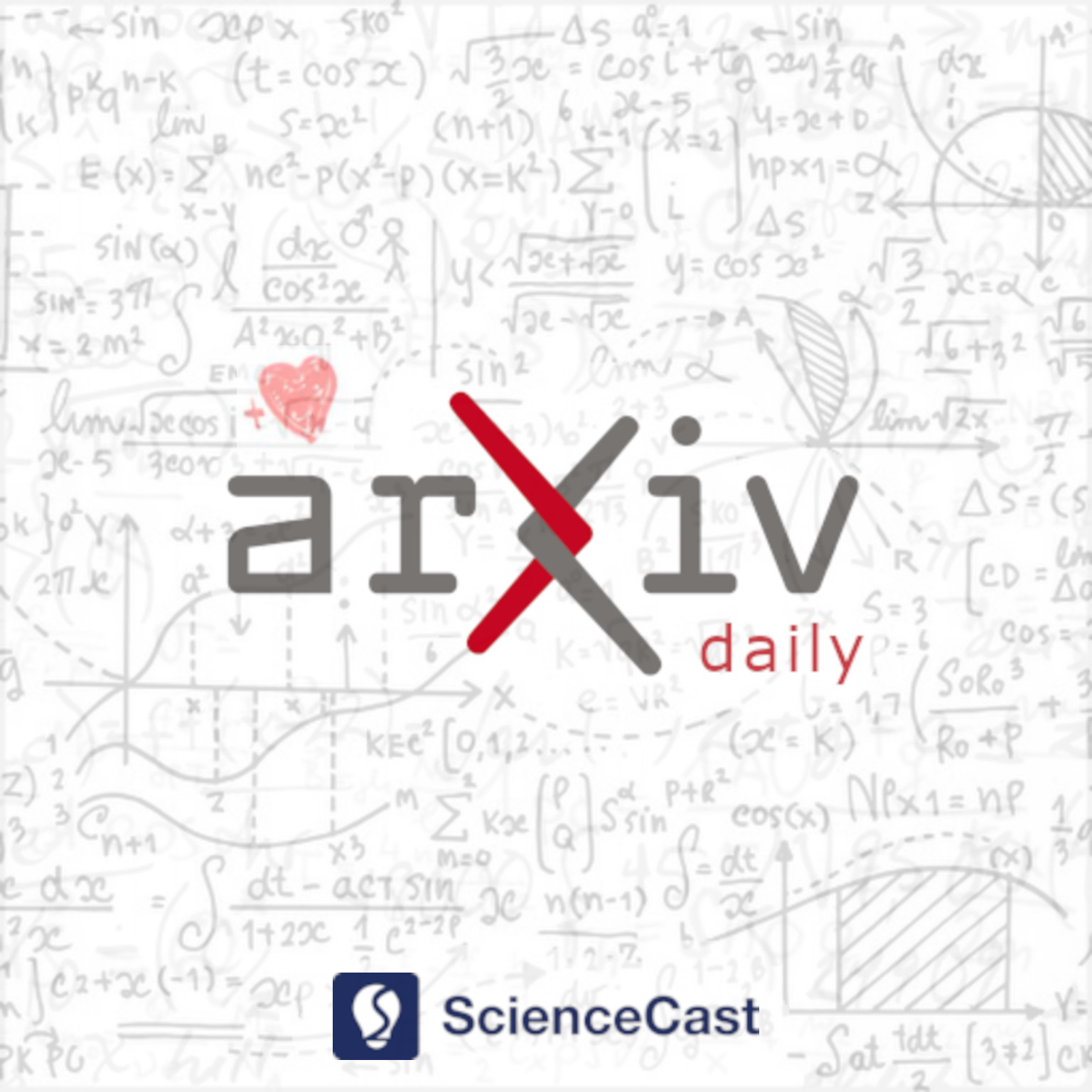
Materials Science (cond-mat.mtrl-sci)
Wed, 21 Jun 2023
1.First-principles prediction of structural, magnetic properties of Cr-substituted strontium hexaferrite, and its site preference
Authors:Binod Regmi, Dinesh Thapa, Bipin Lamichhane, Seong-Gon Kim
Abstract: To investigate the structural and magnetic properties of Cr-doped M-type strontium hexaferrite (SrFe$_{12}$O$_{19}$) with x = (0.0, 0.5, 1.0), we perform first-principles total-energy calculations relied on density functional theory. Based on the calculation of the substitution energy of Cr in strontium hexaferrite and formation probability analysis, we conclude that the doped Cr atoms prefer to occupy the 2a, 12k, and 4f$_{2}$ sites which is in good agreement with the experimental findings. Due to Cr$^{3+}$ ion moment, 3 {$\mu_B$}, smaller than that of Fe$^{3+}$ ion, 5 {$\mu_B$}, saturation magnetization (M$_{s}$) reduce rapidly as the concentration of Cr increases in strontium hexaferrite. The magnetic anisotropic field $\left(H_{a}\right)$ rises with an increasing fraction of Cr despite a significant reduction of magnetization and a slight increase of magnetocrystalline anisotropy $\left(K_{1}\right)$.The cause for the rise in magnetic anisotropy field $\left(H_{a}\right)$ with an increasing fraction of Cr is further emphasized by our formation probability study. Cr$^{3+}$ ions prefer to occupy the 2a sites at lower temperatures, but as the temperature rises, it is more likely that they will occupy the 12k site. Cr$^{3+}$ ions are more likely to occupy the 12k site than the 2a site at a specific annealing temperature (>700{\deg}C).
2.An Analytical Model to Quantify the Local Lattice Distortion of Refractory High Entropy Alloys
Authors:Zhiling Luo, Wang Gao, Qing Jiang
Abstract: Local lattice distortion (LLD) of high entropy alloys (HEAs) especially refractory HEAs, which is different from one lattice site to another, determines the mechanical properties of HEAs such as yield strength and radiation resistance, and is crucial to modulating catalytic activity of HEAs via the atomic strain. In particular, this site-to-site LLD is strongly coupled with the short-range order (SRO) of HEAs. Therefore it is essential to reveal the physical picture of LLD. However, the random distribution of multi-principal constituents of HEAs prohibits the understanding of LLD, including the determinants of LLD and their coupling rules. Herein, we build the first analytical model to realize the site-to-site prediction of LLD in refractory HEAs, by using the neighbor number ratio of central atoms, the central-atom radii, the standard deviation of constituent radii and the constituent number, which demonstrates that LLD surprisingly exhibits a similar mechanism as the relaxation of metal surfaces. The involved parameters depend only on the radii of constituents and are readily accessible. Moreover, our scheme determines not only LLD but also the average lattice distortion, which enables us to predict the phase stability and yield strength of HEAs. These results build a novel physical picture of LLD, in particular the quantitative relationship between LLD and SRO, which lay a solid foundation for the further target-oriented design of HEAs.
3.Designing Pr-based Advanced Photoluminescent Materials using Machine Learning and Density Functional Theory
Authors:Upendra Kumar, Hyeon Woo Kim, Sobhit Singh, Hyunseok Ko, Sung Beom Cho
Abstract: This work presents a machine learning approach to predict novel perovskite oxide materials in the Pr-Al-O and Pr-Sc-O compound families with the potential for photoluminescence applications. The predicted materials exhibit a large bandgap and high Debye temperature, and have remained unexplored thus far. The predicted compounds (Pr$_3$AlO$_6$, Pr$_4$Al$_2$O$_9$, Pr$_3$ScO$_6$ and Pr$_3$Sc$_5$O$_{12}$) are screened using machine learning approach, which are then confirmed by density functional theory calculations. The study includes the calculation of the bandgap and density of states to determine electronic properties, and the optical absorption and emission spectra to determine optical properties. Mechanical stability of the predicted compounds, as demonstrated by satisfying the Born-Huang criterion. By combining machine learning and density functional theory, this work offers a more efficient and comprehensive approach to materials discovery and design.