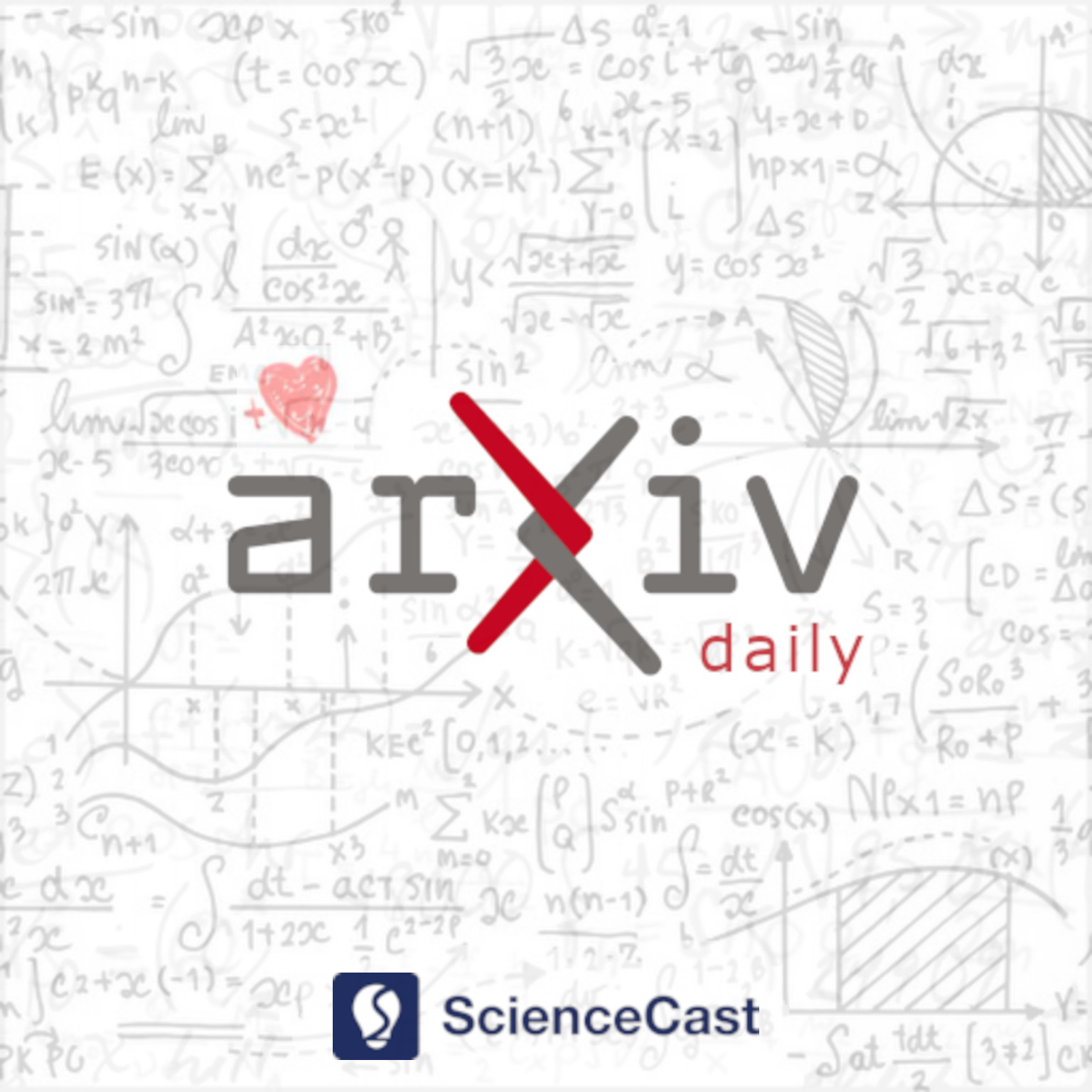
General Economics (econ.GN)
Thu, 14 Sep 2023
1.Computer says 'no': Exploring systemic hiring bias in ChatGPT using an audit approach
Authors:Louis Lippens
Abstract: Large language models offer significant potential for optimising professional activities, such as streamlining personnel selection procedures. However, concerns exist about these models perpetuating systemic biases embedded into their pre-training data. This study explores whether ChatGPT, a chatbot producing human-like responses to language tasks, displays ethnic or gender bias in job applicant screening. Using a correspondence audit approach, I simulated a CV screening task in which I instructed the chatbot to rate fictitious applicant profiles only differing in names, signalling ethnic and gender identity. Comparing ratings of Arab, Asian, Black American, Central African, Dutch, Eastern European, Hispanic, Turkish, and White American male and female applicants, I show that ethnic and gender identity influence ChatGPT's evaluations. The ethnic bias appears to arise partly from the prompts' language and partly from ethnic identity cues in applicants' names. Although ChatGPT produces no overall gender bias, I find some evidence for a gender-ethnicity interaction effect. These findings underscore the importance of addressing systemic bias in language model-driven applications to ensure equitable treatment across demographic groups. Practitioners aspiring to adopt these tools should practice caution, given the adverse impact they can produce, especially when using them for selection decisions involving humans.