PEFT-SP: Parameter-Efficient Fine-Tuning on Large Protein Language Models Improves Signal Peptide Prediction
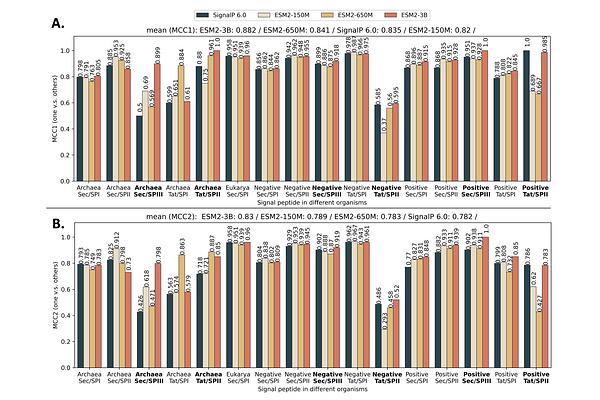
PEFT-SP: Parameter-Efficient Fine-Tuning on Large Protein Language Models Improves Signal Peptide Prediction
Zeng, S.; Wang, D.; Xu, D.
AbstractSignal peptides (SP) play a crucial role in protein translocation in cells. The development of large protein language models (PLMs) provides a new opportunity for SP prediction, especially for the categories with limited annotated data. We present a Parameter-Efficient Fine-Tuning (PEFT) framework for SP predic- tion, PEFT-SP, to effectively utilize pre-trained PLMs. We implanted low-rank adaptation (LoRA) into ESM- 2 models to better leverage the protein sequence evolutionary knowledge of PLMs. Experiments show that PEFT-SP using LoRA enhances state-of-the-art results, leading to a maximum MCC2 gain of 0.372 for SPs with small training samples and an overall MCC2 gain of 0.048. Furthermore, we also employed two other PEFT methods, i.e., Prompt Tunning and Adapter Tuning, into ESM-2 for SP prediction. More elaborate ex- periments show that PEFT-SP using Adapter Tuning can also improve the state-of-the-art results with up to 0.202 MCC2 gain for SPs with small training samples and an overall MCC2 gain of 0.030. LoRA requires fewer computing resources and less memory compared to Adapter, making it possible to adapt larger and more powerful protein models for SP prediction.