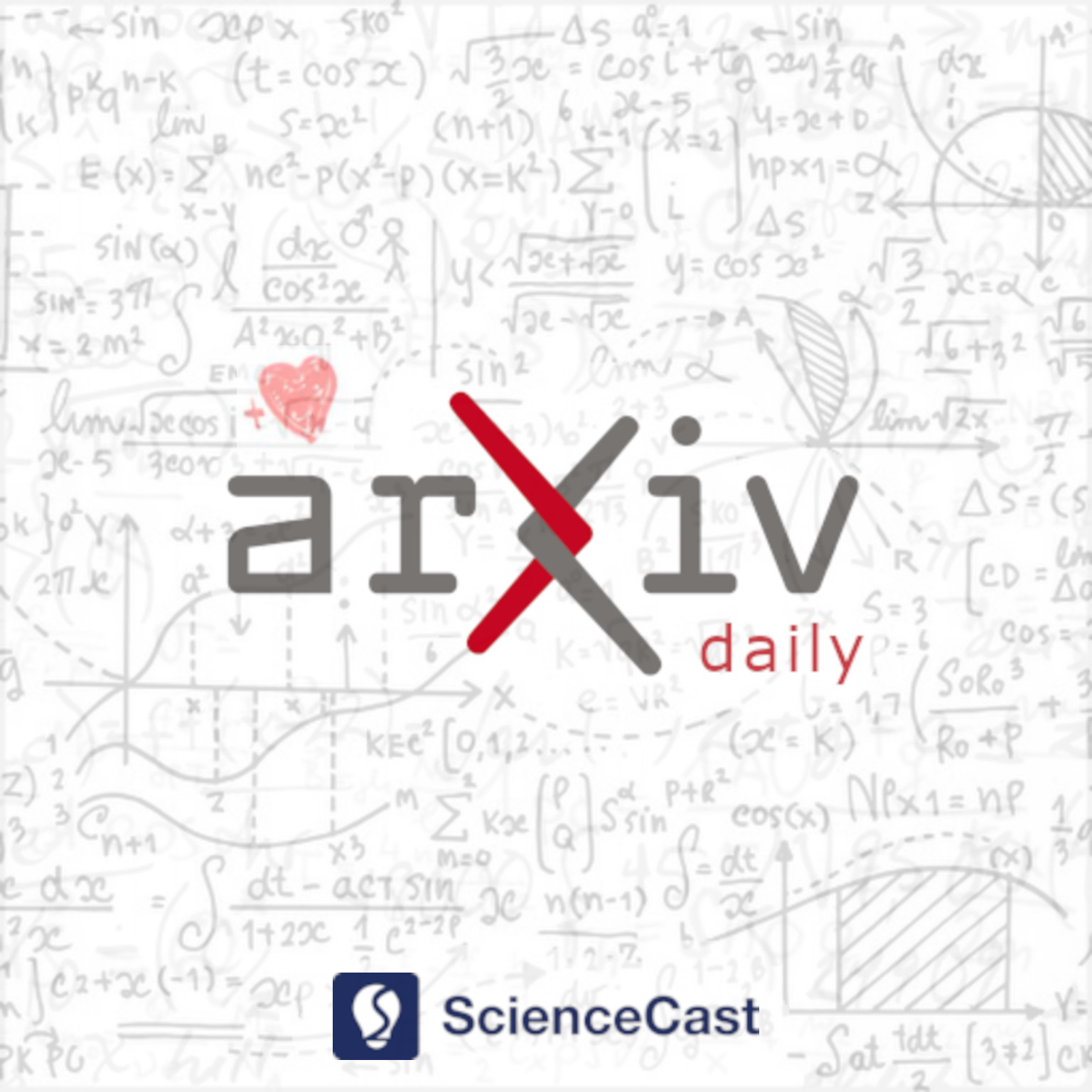
Databases (cs.DB)
Wed, 19 Jul 2023
1.Validation of Modern JSON Schema: Formalization and Complexity
Authors:Lyes Attouche, Mohamed-Amine Baazizi, Dario Colazzo, Giorgio Ghelli, Carlo Sartiani, Stefanie Scherzinger
Abstract: JSON Schema is the de-facto standard schema language for JSON data. The language went through many minor revisions, but the most recent versions of the language added two novel features, dynamic references and annotation-dependent validation, that change the evaluation model. Modern JSON Schema is the name used to indicate all versions from Draft 2019-09, which are characterized by these new features, while Classical JSON Schema is used to indicate the previous versions. These new "modern" features make the schema language quite difficult to understand, and have generated many discussions about the correct interpretation of their official specifications; for this reason we undertook the task of their formalization. During this process, we also analyzed the complexity of data validation in Modern JSON Schema, with the idea of confirming the PTIME complexity of Classical JSON Schema validation, and we were surprised to discover a completely different truth: data validation, that is expected to be an extremely efficient process, acquires, with Modern JSON Schema features, a PSPACE complexity. In this paper, we give the first formal description of Modern JSON Schema, which we consider a central contribution of the work that we present here. We then prove that its data validation problem is PSPACE-complete. We prove that the origin of the problem lies in dynamic references, and not in annotation-dependent validation. We study the schema and data complexities, showing that the problem is PSPACE-complete with respect to the schema size even with a fixed instance, but is in PTIME when the schema is fixed and only the instance size is allowed to vary. Finally, we run experiments that show that there are families of schemas where the difference in asymptotic complexity between dynamic and static references is extremely visible, even with small schemas.
2.Efficient Non-Learning Similar Subtrajectory Search
Authors:Jiabao Jin, Peng Cheng, Lei Chen, Xuemin Lin, Wenjie Zhang
Abstract: Similar subtrajectory search is a finer-grained operator that can better capture the similarities between one query trajectory and a portion of a data trajectory than the traditional similar trajectory search, which requires the two checked trajectories are similar to each other in whole. Many real applications (e.g., trajectory clustering and trajectory join) utilize similar subtrajectory search as a basic operator. It is considered that the time complexity is O(mn^2) for exact algorithms to solve the similar subtrajectory search problem under most trajectory distance functions in the existing studies, where m is the length of the query trajectory and n is the length of the data trajectory. In this paper, to the best of our knowledge, we are the first to propose an exact algorithm to solve the similar subtrajectory search problem in O(mn) time for most of widely used trajectory distance functions (e.g., WED, DTW, ERP, EDR and Frechet distance). Through extensive experiments on three real datasets, we demonstrate the efficiency and effectiveness of our proposed algorithms.