Emergent World Representations: Exploring a Sequence Model Trained on a Synthetic Task
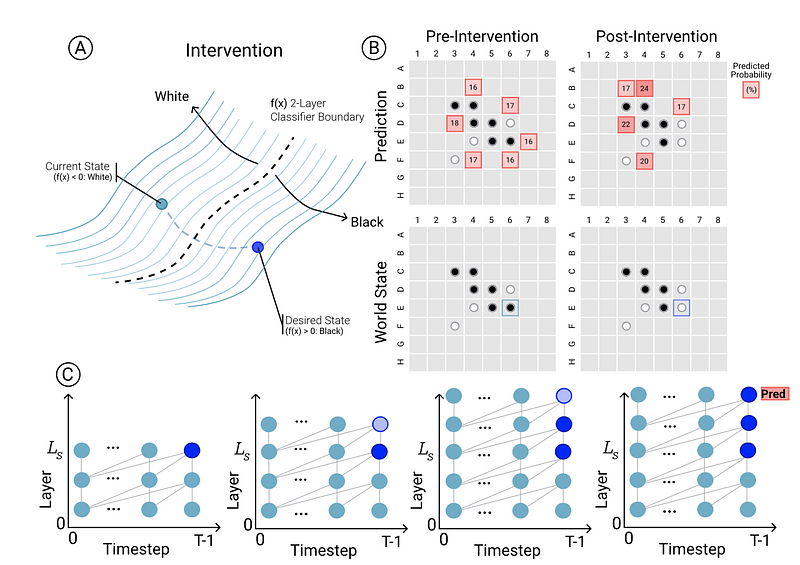
Emergent World Representations: Exploring a Sequence Model Trained on a Synthetic Task
Kenneth Li, Aspen K. Hopkins, David Bau, Fernanda Viégas, Hanspeter Pfister, Martin Wattenberg
AbstractLanguage models show a surprising range of capabilities, but the source of their apparent competence is unclear. Do these networks just memorize a collection of surface statistics, or do they rely on internal representations of the process that generates the sequences they see? We investigate this question by applying a variant of the GPT model to the task of predicting legal moves in a simple board game, Othello. Although the network has no a priori knowledge of the game or its rules, we uncover evidence of an emergent nonlinear internal representation of the board state. Interventional experiments indicate this representation can be used to control the output of the network and create "latent saliency maps" that can help explain predictions in human terms.