Emergent representations in networks trained with the Forward-Forward algorithm
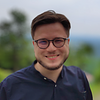
Emergent representations in networks trained with the Forward-Forward algorithm
Niccolò Tosato, Lorenzo Basile, Emanuele Ballarin, Giuseppe de Alteriis, Alberto Cazzaniga, Alessio Ansuini
AbstractThe Backpropagation algorithm, widely used to train neural networks, has often been criticised for its lack of biological realism. In an attempt to find a more biologically plausible alternative, and avoid to back-propagate gradients in favour of using local learning rules, the recently introduced Forward-Forward algorithm replaces the traditional forward and backward passes of Backpropagation with two forward passes. In this work, we show that internal representations obtained with the Forward-Forward algorithm organize into robust, category-specific ensembles, composed by an extremely low number of active units (high sparsity). This is remarkably similar to what is observed in cortical representations during sensory processing. While not found in models trained with standard Backpropagation, sparsity emerges also in networks optimized by Backpropagation, on the same training objective of Forward-Forward. These results suggest that the learning procedure proposed by Forward-Forward may be superior to Backpropagation in modelling learning in the cortex, even when a backward pass is used.