VideoFACT: Detecting Video Forgeries Using Attention, Scene Context, and Forensic Traces
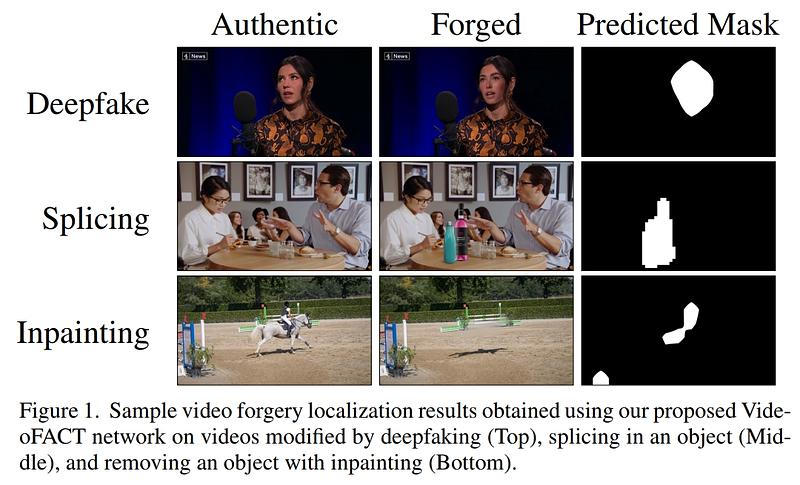
VideoFACT: Detecting Video Forgeries Using Attention, Scene Context, and Forensic Traces
Tai D. Nguyen, Shengbang Fang, Matthew C. Stamm
AbstractFake videos represent an important misinformation threat. While existing forensic networks have demonstrated strong performance on image forgeries, recent results reported on the Adobe VideoSham dataset show that these networks fail to identify fake content in videos. In this paper, we show that this is due to video coding, which introduces local variation into forensic traces. In response, we propose VideoFACT - a new network that is able to detect and localize a wide variety of video forgeries and manipulations. To overcome challenges that existing networks face when analyzing videos, our network utilizes both forensic embeddings to capture traces left by manipulation, context embeddings to control for variation in forensic traces introduced by video coding, and a deep self-attention mechanism to estimate the quality and relative importance of local forensic embeddings. We create several new video forgery datasets and use these, along with publicly available data, to experimentally evaluate our network's performance. These results show that our proposed network is able to identify a diverse set of video forgeries, including those not encountered during training. Furthermore, we show that our network can be fine-tuned to achieve even stronger performance on challenging AI-based manipulations.