Shift-tolerant Perceptual Similarity Metric
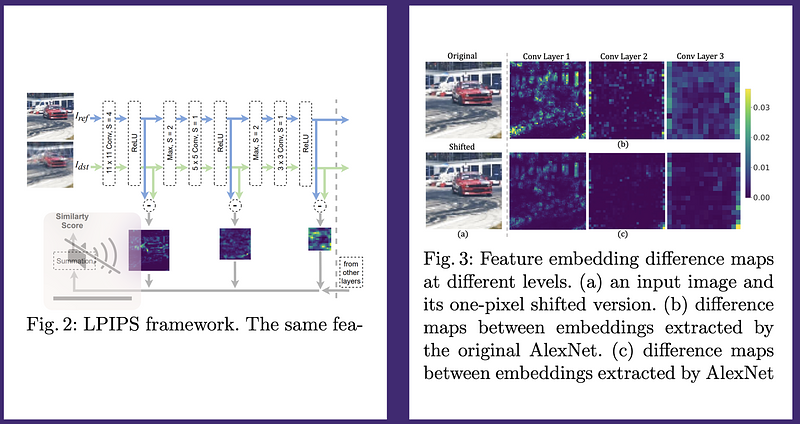
Shift-tolerant Perceptual Similarity Metric
Abhijay Ghildyal, Feng Liu
AbstractExisting perceptual similarity metrics assume an image and its reference are well aligned. As a result, these metrics are often sensitive to a small alignment error that is imperceptible to the human eyes. This paper studies the effect of small misalignment, specifically a small shift between the input and reference image, on existing metrics, and accordingly develops a shift-tolerant similarity metric. This paper builds upon LPIPS, a widely used learned perceptual similarity metric, and explores architectural design considerations to make it robust against imperceptible misalignment. Specifically, we study a wide spectrum of neural network elements, such as anti-aliasing filtering, pooling, striding, padding, and skip connection, and discuss their roles in making a robust metric. Based on our studies, we develop a new deep neural network-based perceptual similarity metric. Our experiments show that our metric is tolerant to imperceptible shifts while being consistent with the human similarity judgment.