Unsupervised Voice Activity Detection by Modeling Source and System Information using Zero Frequency Filtering
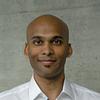
Unsupervised Voice Activity Detection by Modeling Source and System Information using Zero Frequency Filtering
Eklavya Sarkar, RaviShankar Prasad, Mathew Magimai. -Doss
AbstractVoice activity detection (VAD) is an important pre-processing step for speech technology applications. The task consists of deriving segment boundaries of audio signals which contain voicing information. In recent years, it has been shown that voice source and vocal tract system information can be extracted using zero-frequency filtering (ZFF) without making any explicit model assumptions about the speech signal. This paper investigates the potential of zero-frequency filtering for jointly modeling voice source and vocal tract system information, and proposes two approaches for VAD. The first approach demarcates voiced regions using a composite signal composed of different zero-frequency filtered signals. The second approach feeds the composite signal as input to the rVAD algorithm. These approaches are compared with other supervised and unsupervised VAD methods in the literature, and are evaluated on the Aurora-2 database, across a range of SNRs (20 to -5 dB). Our studies show that the proposed ZFF-based methods perform comparable to state-of-art VAD methods and are more invariant to added degradation and different channel characteristics.