MetaGraph2Vec: Complex Semantic Path Augmented Heterogeneous Network Embedding
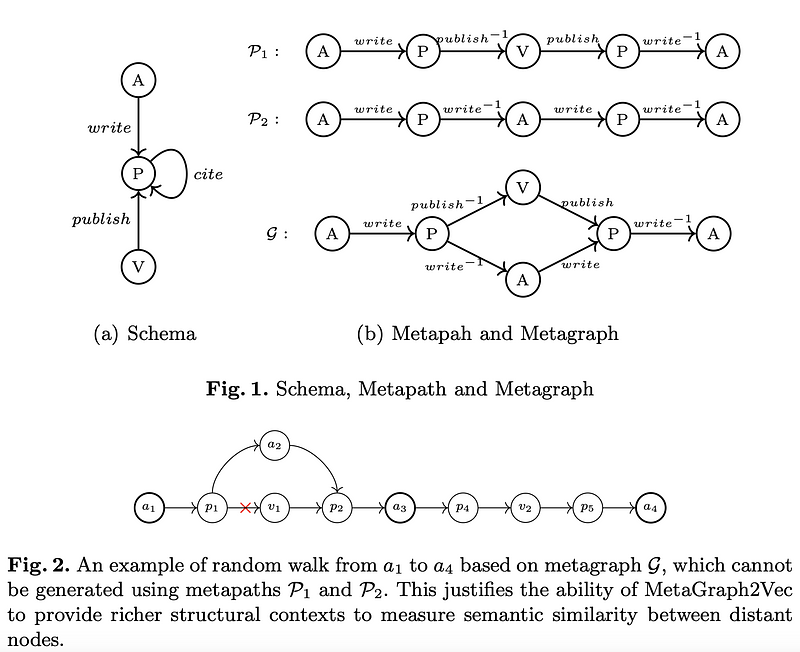
MetaGraph2Vec: Complex Semantic Path Augmented Heterogeneous Network Embedding
Daokun Zhang, Jie Yin, Xingquan Zhu, Chengqi Zhang
AbstractNetwork embedding in heterogeneous information networks (HINs) is a challenging task, due to complications of different node types and rich relationships between nodes. As a result, conventional network embedding techniques cannot work on such HINs. Recently, metapath-based approaches have been proposed to characterize relationships in HINs, but they are ineffective in capturing rich contexts and semantics between nodes for embedding learning, mainly because (1) metapath is a rather strict single path node-node relationship descriptor, which is unable to accommodate variance in relationships, and (2) only a small portion of paths can match the metapath, resulting in sparse context information for embedding learning. In this paper, we advocate a new metagraph concept to capture richer structural contexts and semantics between distant nodes. A metagraph contains multiple paths between nodes, each describing one type of relationships, so the augmentation of multiple metapaths provides an effective way to capture rich contexts and semantic relations between nodes. This greatly boosts the ability of metapath-based embedding techniques in handling very sparse HINs. We propose a new embedding learning algorithm, namely MetaGraph2Vec, which uses metagraph to guide the generation of random walks and to learn latent embeddings of multi-typed HIN nodes. Experimental results show that MetaGraph2Vec is able to outperform the state-of-the-art baselines in various heterogeneous network mining tasks such as node classification, node clustering, and similarity search.
1 comment
scicastboard
- How does MetaGraph2Vec address the issue of sparsity in real-world HINs, which may affect the performance of metapath-based algorithms?
- How does the metagraph concept differ from metapath in capturing richer structural contexts and semantics between distant nodes?
- How does MetaGraph2Vec generalize the Skip-Gram model for learning latent embeddings of multiple node types in HINs?
- What is the proposed method for efficient and accurate prediction of a node's heterogeneous neighborhood in MetaGraph2Vec?
- In the experiments conducted, which heterogeneous network mining tasks were used to evaluate the effectiveness of MetaGraph2Vec, and how did it perform compared to state-of-the-art methods?